Projection decomposition algorithm for dual-energy computed tomography via deep neural network.
JOURNAL OF X-RAY SCIENCE AND TECHNOLOGY(2018)
摘要
BACKGROUND: Dual-energy computed tomography (DECT) has been widely used to improve identification of substances from different spectral information. Decomposition of the mixed test samples into two materials relies on a well-calibrated material decomposition function. OBJECTIVE: This work aims to establish and validate a data-driven algorithm for estimation of the decomposition function. METHODS: A deep neural network (DNN) consisting of two sub-nets is proposed to solve the projection decomposition problem. The compressing sub-net, substantially a stack auto-encoder (SAE), learns a compact representation of energy spectrum. The decomposing sub-net with a two-layer structure fits the nonlinear transform between energy projection and basic material thickness. RESULTS: The proposed DNN not only delivers image with lower standard deviation and higher quality in both simulated and real data, and also yields the best performance in cases mixed with photon noise. Moreover, DNN costs only 0.4 s to generate a decomposition solution of 360 x 512 size scale, which is about 200 times faster than the competing algorithms. CONCLUSIONS: The DNN model is applicable to the decomposition tasks with different dual energies. Experimental results demonstrated the strong function fitting ability of DNN. Thus, the Deep learning paradigm provides a promising approach to solve the nonlinear problem in DECT.
更多查看译文
关键词
Dual-energy computed tomography,material decomposition,deep learning,stack auto-encoder
AI 理解论文
溯源树
样例
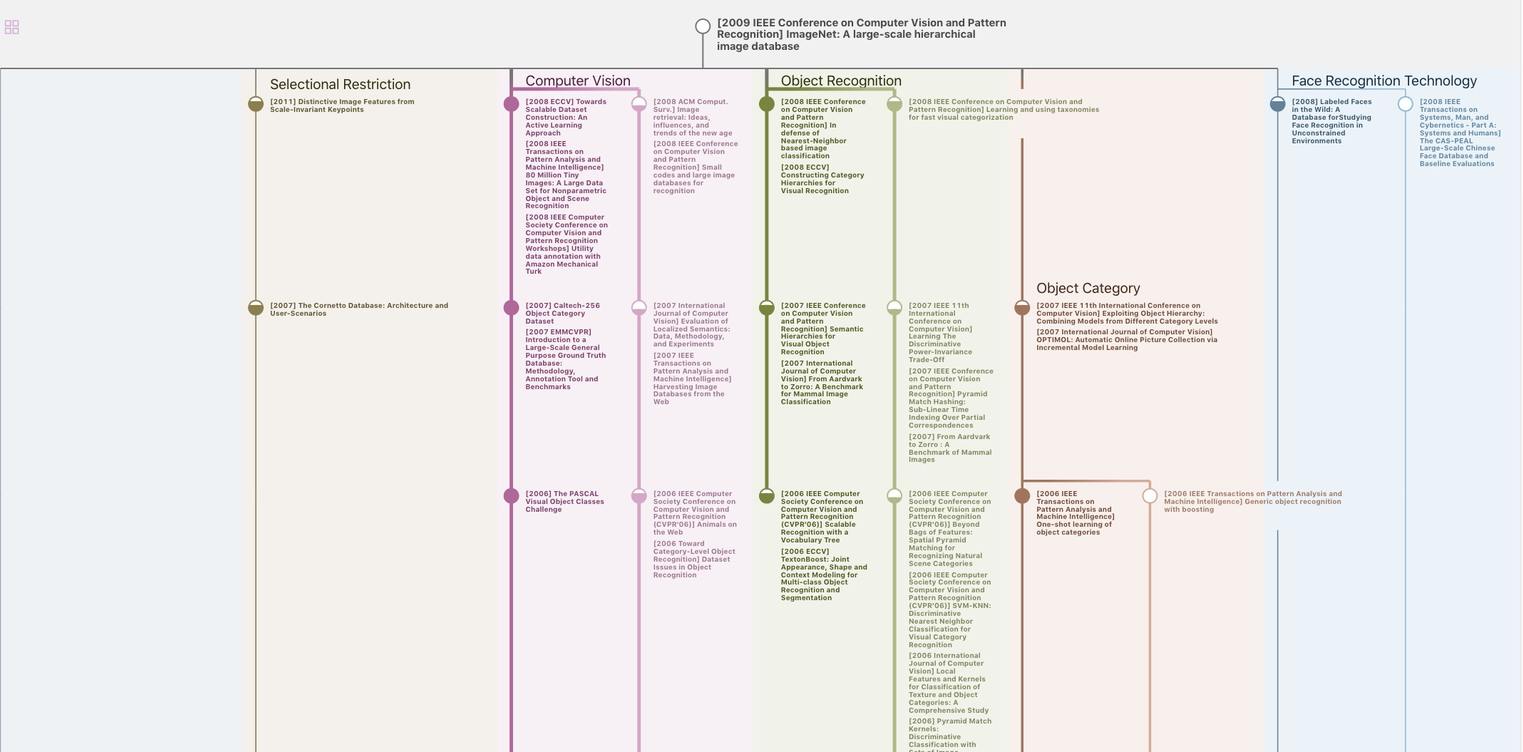
生成溯源树,研究论文发展脉络
Chat Paper
正在生成论文摘要