Predicting vasovagal syncope from heart rate and blood pressure: A prospective study in 140 subjects.
Heart rhythm(2018)
摘要
BACKGROUND:We developed a vasovagal syncope (VVS) prediction algorithm for use during head-up tilt with simultaneous analysis of heart rate (HR) and systolic blood pressure (SBP). We previously tested this algorithm retrospectively in 1155 subjects, showing sensitivity 95%, specificity 93%, and median prediction time 59 seconds.
OBJECTIVE:The purpose of this prospective, single-center study of 140 subjects was to evaluate this VVS prediction algorithm and to assess whether retrospective results were reproduced and clinically relevant. The primary endpoint was VVS prediction: sensitivity and specificity >80%.
METHODS:In subjects referred for 60° head-up tilt (Italian protocol), noninvasive HR and SBP were supplied to the VVS prediction algorithm: simultaneous analysis of RR intervals, SBP trends, and their variability represented by low-frequency power-generated cumulative risk, which was compared with a predetermined VVS risk threshold. When cumulative risk exceeded threshold, an alert was generated. Prediction time was duration between first alert and syncope.
RESULTS:Of the 140 subjects enrolled, data were usable for 134. Of 83 tilt-positive subjects (61.9%), 81 VVS events were correctly predicted by the algorithm, and of 51 tilt-negative subjects (38.1%), 45 were correctly identified as negative by the algorithm. Resulting algorithm performance was sensitivity 97.6% and specificity 88.2%, meeting the primary endpoint. Mean VVS prediction time was 2 minutes 26 seconds ± 3 minutes 16 seconds (median 1 minute 25 seconds). Using only HR and HR variability (without SBP), mean prediction time reduced to 1 minute 34 seconds ± 1 minute 45 seconds (median 1 minute 13 seconds).
CONCLUSION:The VVS prediction algorithm is a clinically relevant tool and could offer applications, including providing a patient alarm, shortening tilt-test time, and triggering pacing intervention in implantable devices.
更多查看译文
AI 理解论文
溯源树
样例
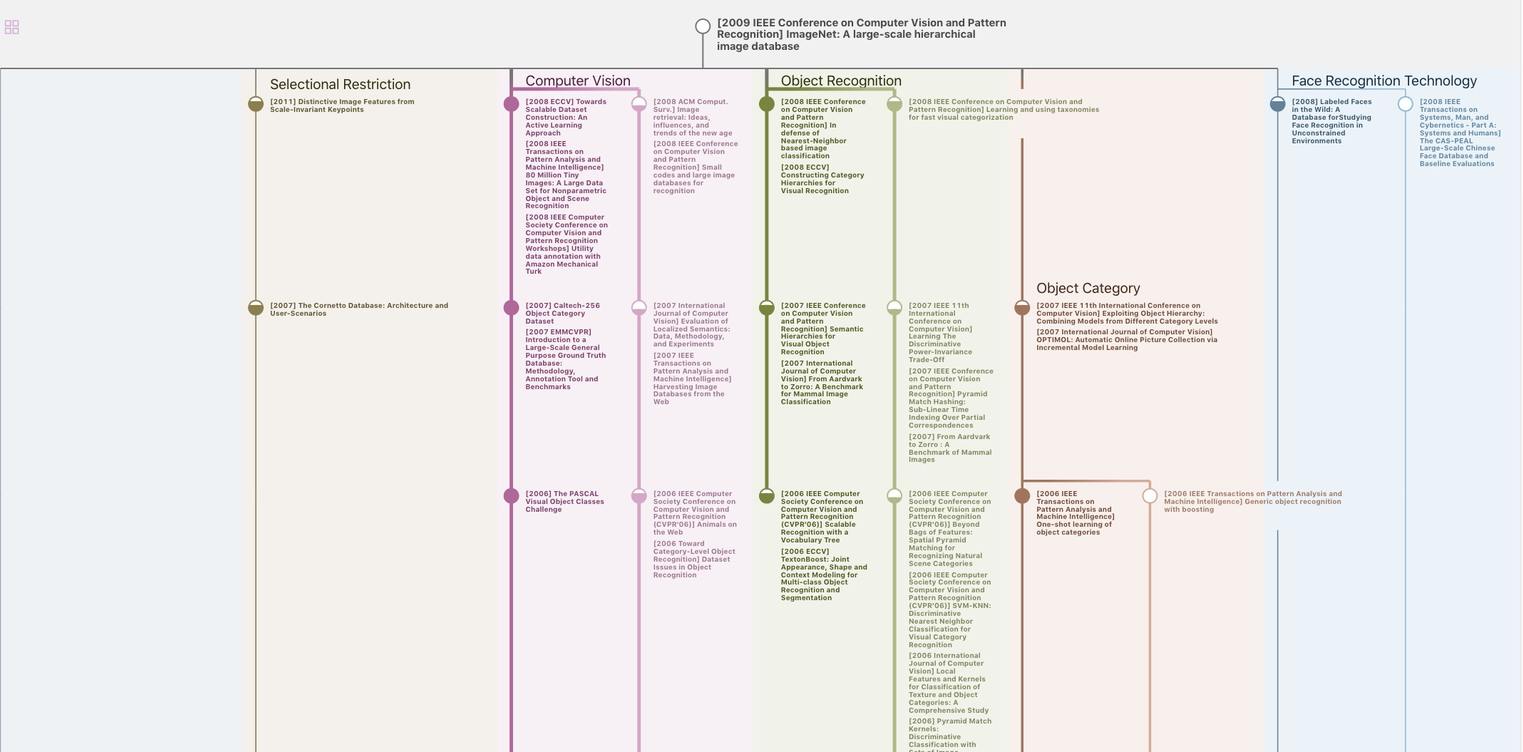
生成溯源树,研究论文发展脉络
Chat Paper
正在生成论文摘要