Exploring Explainable Selection to Control Abstractive Summarization
Proceedings of the AAAI Conference on Artificial Intelligence(2021)
摘要
Like humans, document summarization models can interpret a document'scontents in a number of ways. Unfortunately, the neural models of today arelargely black boxes that provide little explanation of how or why theygenerated a summary in the way they did. Therefore, to begin prying open theblack box and to inject a level of control into the substance of the finalsummary, we developed a novel select-and-generate framework that focuses onexplainability. By revealing the latent centrality and interactions betweensentences, along with scores for sentence novelty and relevance, users aregiven a window into the choices a model is making and an opportunity to guidethose choices in a more desirable direction. A novel pair-wise matrix capturesthe sentence interactions, centrality, and attribute scores, and a mask withtunable attribute thresholds allows the user to control which sentences arelikely to be included in the extraction. A sentence-deployed attentionmechanism in the abstractor ensures the final summary emphasizes the desiredcontent. Additionally, the encoder is adaptable, supporting both Transformer-and BERT-based configurations. In a series of experiments assessed with ROUGEmetrics and two human evaluations, ESCA outperformed eight state-of-the-artmodels on the CNN/DailyMail and NYT50 benchmark datasets.
更多查看译文
关键词
Extraction,Topic Modeling,Word Representation,Syntax-based Translation Models,Language Modeling
AI 理解论文
溯源树
样例
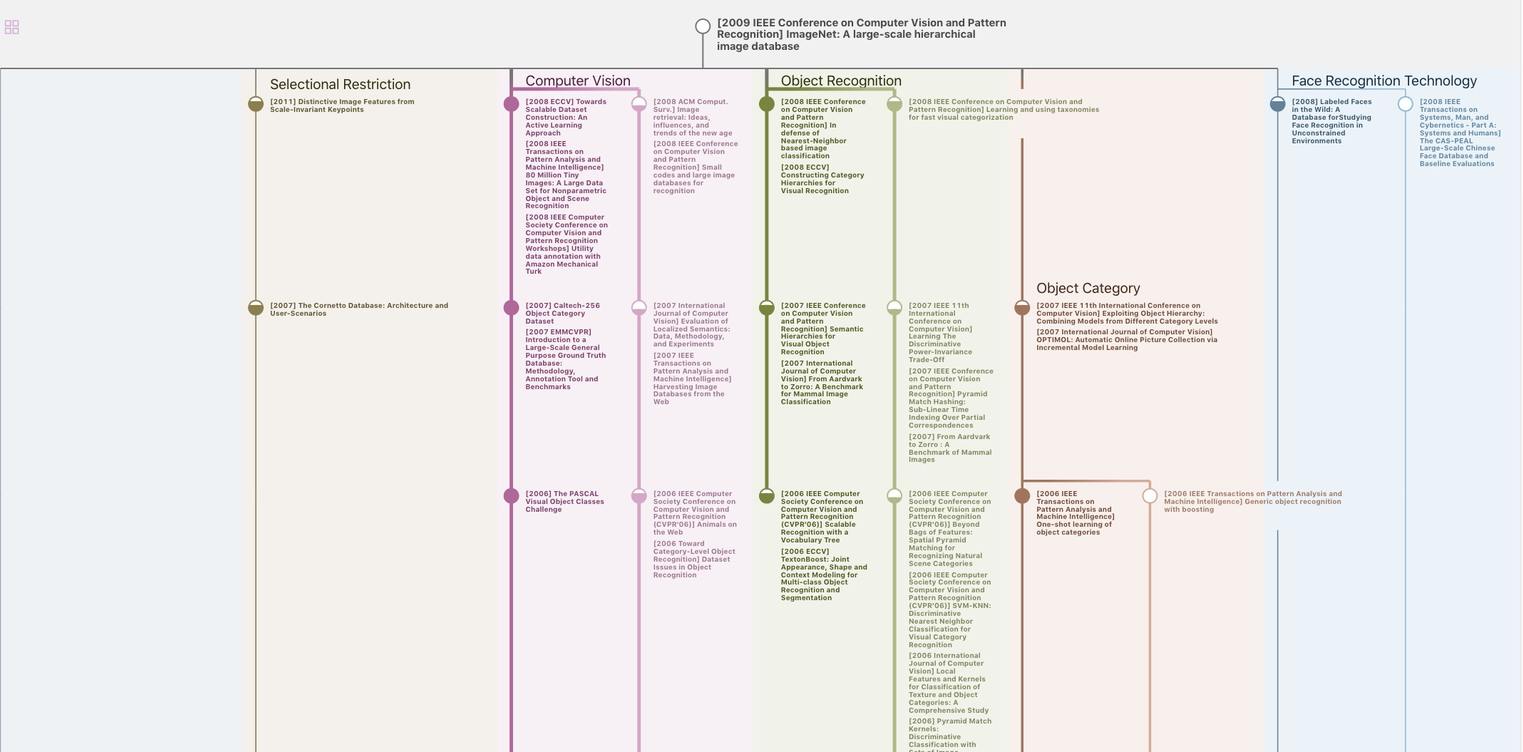
生成溯源树,研究论文发展脉络
Chat Paper
正在生成论文摘要