The Added Value of Diffusion-Weighted MRI-Derived Structural Connectome in Evaluating Mild Cognitive Impairment: A Multi-Cohort Validation1.
Journal of Alzheimer's disease : JAD(2018)
Abstract
T1-weighted MRI has been extensively used to extract imaging biomarkers and build classification models for differentiating Alzheimer's disease (AD) patients from healthy controls, but only recently have brain connectome networks derived from diffusion-weighted MRI been used to model AD progression and various stages of disease such as mild cognitive impairment (MCI). MCI, as a possible prodromal stage of AD, has gained intense interest recently, since it may be used to assess risk factors for AD. Little work has been done to combine information from both white matter and gray matter, and it is unknown how much classification power the diffusion-weighted MRI-derived structural connectome could provide beyond information available from T1-weighted MRI. In this paper, we focused on investigating whether diffusion-weighted MRI-derived structural connectome can improve differentiating healthy controls subjects from those with MCI. Specifically, we proposed a novel feature-ranking method to build classification models using the most highly ranked feature variables to classify MCI with healthy controls. We verified our method on two independent cohorts including the second stage of Alzheimer's Disease Neuroimaging Initiative (ADNI2) database and the National Alzheimer's Coordinating Center (NACC) database. Our results indicated that 1) diffusion-weighted MRI-derived structural connectome can complement T1-weighted MRI in the classification task; 2) the feature-rank method is effective because of the identified consistent T1-weighted MRI and network feature variables on ADNI2 and NACC. Furthermore, by comparing the top-ranked feature variables from ADNI2, NACC, and combined dataset, we concluded that cross-validation using independent cohorts is necessary and highly recommended.
MoreTranslated text
AI Read Science
Must-Reading Tree
Example
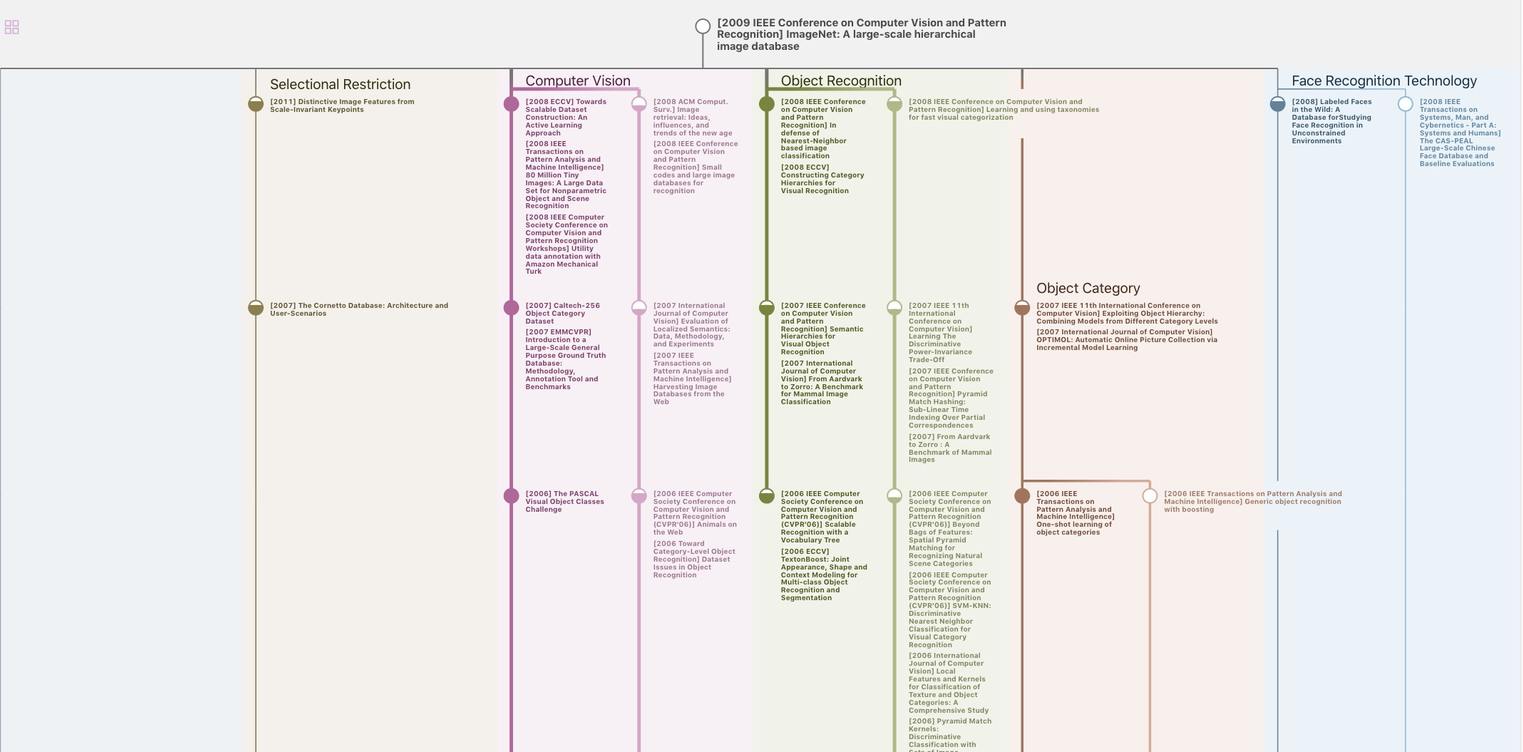
Generate MRT to find the research sequence of this paper
Chat Paper
Summary is being generated by the instructions you defined