Natural Language Processing and Its Implications for the Future of Medication Safety: A Narrative Review of Recent Advances and Challenges.
PHARMACOTHERAPY(2018)
摘要
The safety of medication use has been a priority in the United States since the late 1930s. Recently, it has gained prominence due to the increasing amount of data suggesting that a large amount of patient harm is preventable and can be mitigated with effective risk strategies that have not been sufficiently adopted. Adverse events from medications are part of clinical practice, but the ability to identify a patient's risk and to minimize that risk must be a priority. The ability to identify adverse events has been a challenge due to limitations of available data sources, which are often free text. The use of natural language processing (NLP) may help to address these limitations. NLP is the artificial intelligence domain of computer science that uses computers to manipulate unstructured data (i.e., narrative text or speech data) in the context of a specific task. In this narrative review, we illustrate the fundamentals of NLP and discuss NLP's application to medication safety in four data sources: electronic health records, Internet-based data, published literature, and reporting systems. Given the magnitude of available data from these sources, a growing area is the use of computer algorithms to help automatically detect associations between medications and adverse effects. The main benefit of NLP is in the time savings associated with automation of various medication safety tasks such as the medication reconciliation process facilitated by computers, as well as the potential for near-real-time identification of adverse events for postmarketing surveillance such as those posted on social media that would otherwise go unanalyzed. NLP is limited by a lack of data sharing between health care organizations due to insufficient interoperability capabilities, inhibiting large-scale adverse event monitoring across populations. We anticipate that future work in this area will focus on the integration of data sources from different domains to improve the ability to identify potential adverse events more quickly and to improve clinical decision support with regard to a patient's estimated risk for specific adverse events at the time of medication prescription or review.
更多查看译文
关键词
adverse drug reaction reporting systems,electronic health records,medical informatics,natural language processing,patient safety,pharmacovigilance,social media,supervised machine learning
AI 理解论文
溯源树
样例
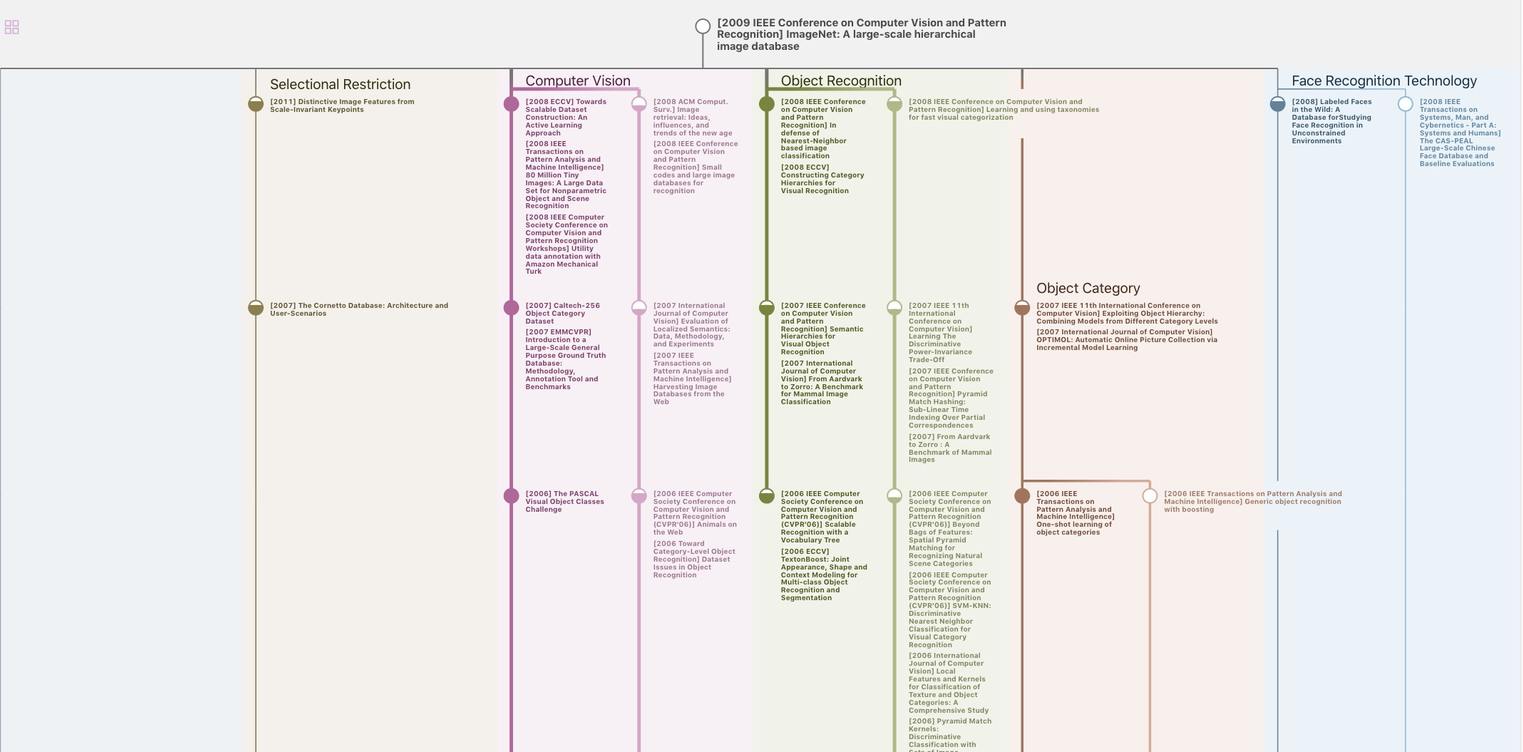
生成溯源树,研究论文发展脉络
Chat Paper
正在生成论文摘要