Effects of Health Level 7 Messaging on Data Quality in New York City's Immunization Information System, 2014.
Public health reports (Washington, D.C. : 1974)(2016)
摘要
OBJECTIVE:We compared the quality of data reported to New York City's immunization information system, the Citywide Immunization Registry (CIR), through its real-time Health Level 7 (HL7) Web service from electronic health records (EHRs), with data submitted through other methods.
METHODS:We stratified immunizations administered and reported to the CIR in 2014 for patients aged 0-18 years by reporting method: (1) sending HL7 messages from EHRs through the Web service, (2) manual data entry, and (3) upload of a non-standard flat file from EHRs. We assessed completeness of reporting by measuring the percentage of immunizations reported with lot number, manufacturer, and Vaccines for Children (VFC) program eligibility. We assessed timeliness of reporting by determining the number of days from date of administration to date entered into the CIR.
RESULTS:HL7 reporting accounted for the largest percentage (46.3%) of the 3.8 million immunizations reported in 2014. Of immunizations reported using HL7, 97.9% included the lot number and 92.6% included the manufacturer, compared with 50.4% and 48.0% for manual entry, and 65.9% and 48.8% for non-standard flat file, respectively. VFC eligibility was 96.9% complete when reported by manual data entry, 95.3% complete for HL7 reporting, and 87.2% complete for non-standard flat file reporting. Of the three reporting methods, HL7 was the most timely: 77.6% of immunizations were reported by HL7 in <1 day, compared with 53.6% of immunizations reported through manual data entry and 18.1% of immunizations reported through non-standard flat file.
CONCLUSION:HL7 reporting from EHRs resulted in more complete and timely data in the CIR compared with other reporting methods. Providing resources to facilitate HL7 reporting from EHRs to immunization information systems to increase data quality should be a priority for public health.
更多查看译文
AI 理解论文
溯源树
样例
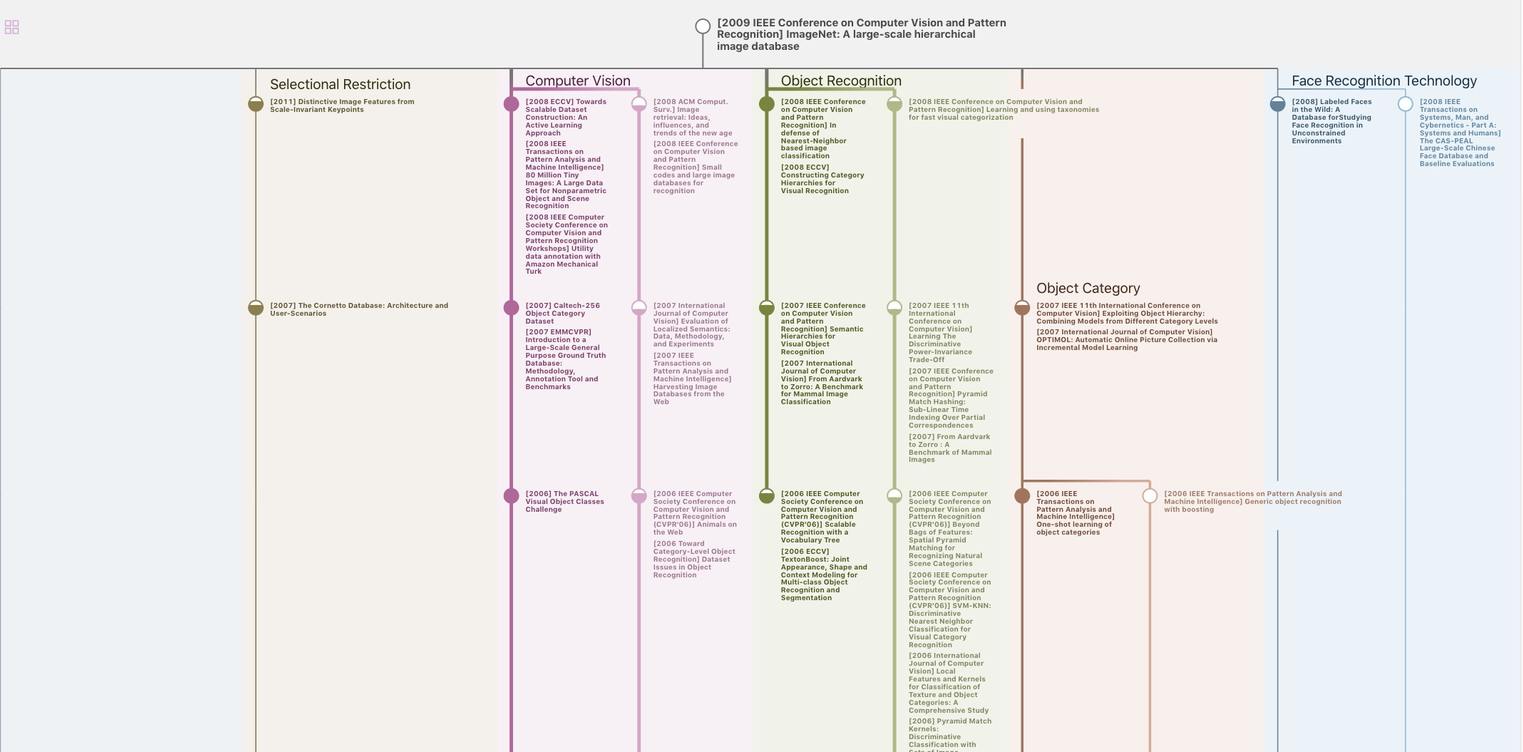
生成溯源树,研究论文发展脉络
Chat Paper
正在生成论文摘要