Kernel-based microfluidic constriction assay for tumor sample identification.
ACS sensors(2018)
摘要
A high-throughput multi-constriction microfluidic channels device can distinguish human breast cancer cell lines (MDA-MB-231, HCC-1806, MCF-7) from immortalized breast cells (MCF-10A) with a confidence level of ~81-85% at a rate of 50-70 cells/min based on velocity increment differences through multi-constriction channels aligned in series. The results are likely related to the deformability differences between non-malignant and malignant breast cells. The data were analyzed by the methods/algorithms of Ridge, nonnegative garrote on kernel machine (NGK), and Lasso using high-dimensional variables, including the cell sizes, velocities and velocity increments. In kernel learning based methods, the prediction values of 10-fold cross-validations are used to represent the difference between two groups of data, where a value of 100% indicates the two groups are completely distinct and identifiable. The prediction value is used to represent the difference between two groups using the established algorithm classifier from high-dimensional variables. These methods were applied to heterogeneous cell populations prepared using primary tumor and adjacent normal tissue obtained from two patients. Primary breast cancer cells were distinguished from patient-matched adjacent normal cells with a prediction ratio of 70.07%-75.96% by NGK method. Thus, this high-throughput multi-constriction microfluidic device together with the kernel learning method can be used to perturb and analyze the biomechanical status of cells obtained from small primary tumor biopsy samples. The resultant biomechanical velocity signatures identify malignancy and provide a new marker for evaluation in risk assessment.
更多查看译文
关键词
breast cancer cells,kernel learning,multiconstriction microfluidic channels,machine learning,variable selection,patient primary tumor cells
AI 理解论文
溯源树
样例
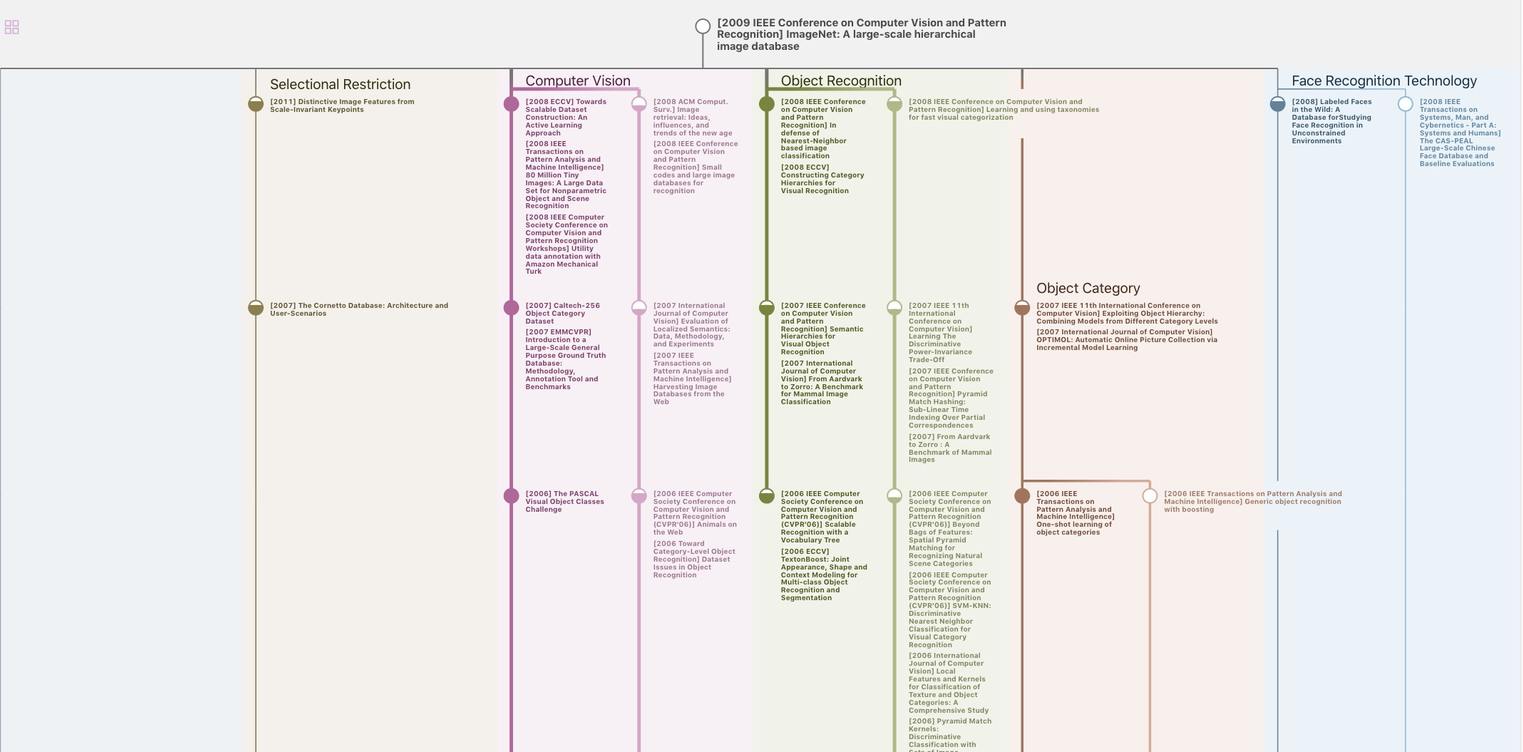
生成溯源树,研究论文发展脉络
Chat Paper
正在生成论文摘要