Unobtrusive Activity Recognition of Elderly People Living Alone Using Anonymous Binary Sensors and DCNN.
IEEE journal of biomedical and health informatics(2019)
摘要
Elderly population (over the age of 60) is predicted to be 1.2 billion by 2025. Most of the elderly people would like to stay alone in their own house due to the high eldercare cost and privacy invasion. Unobtrusive activity recognition is the most preferred solution for monitoring daily activities of the elderly people living alone rather than the camera and wearable devices based systems. Thus, we propose an unobtrusive activity recognition classifier using Deep Convolutional Neural Network (DCNN) and anonymous binary sensors (PIR sensors and door sensors). The real world long-term fully annotated Aruba open dataset collected by binary sensors has been employed for the training and evaluation of the classifier. First, ten basic daily activities, namely: Bed_to_Toilet, Eating, Meal_Preparation, Relax, Sleeping, Work, Housekeeping, Wash_Dishes, Enter_Home, and Leave_Home are segmented with different sliding window sizes, and then converted into binary activity images. Next, the activity images are employed for training the DCNN classifiers with different parameters. Finally, the trained classifiers are evaluated with the 10-fold cross validation method, and the results show that the best DCNN classifier gives 0.79 and 0.951 of F1-score for all 10 activities and eight activities (excluding Leave_Home and Wash_Dishes), respectively.
更多查看译文
关键词
Activity recognition,Senior citizens,Magnetic sensors,Hidden Markov models,Monitoring,Feature extraction
AI 理解论文
溯源树
样例
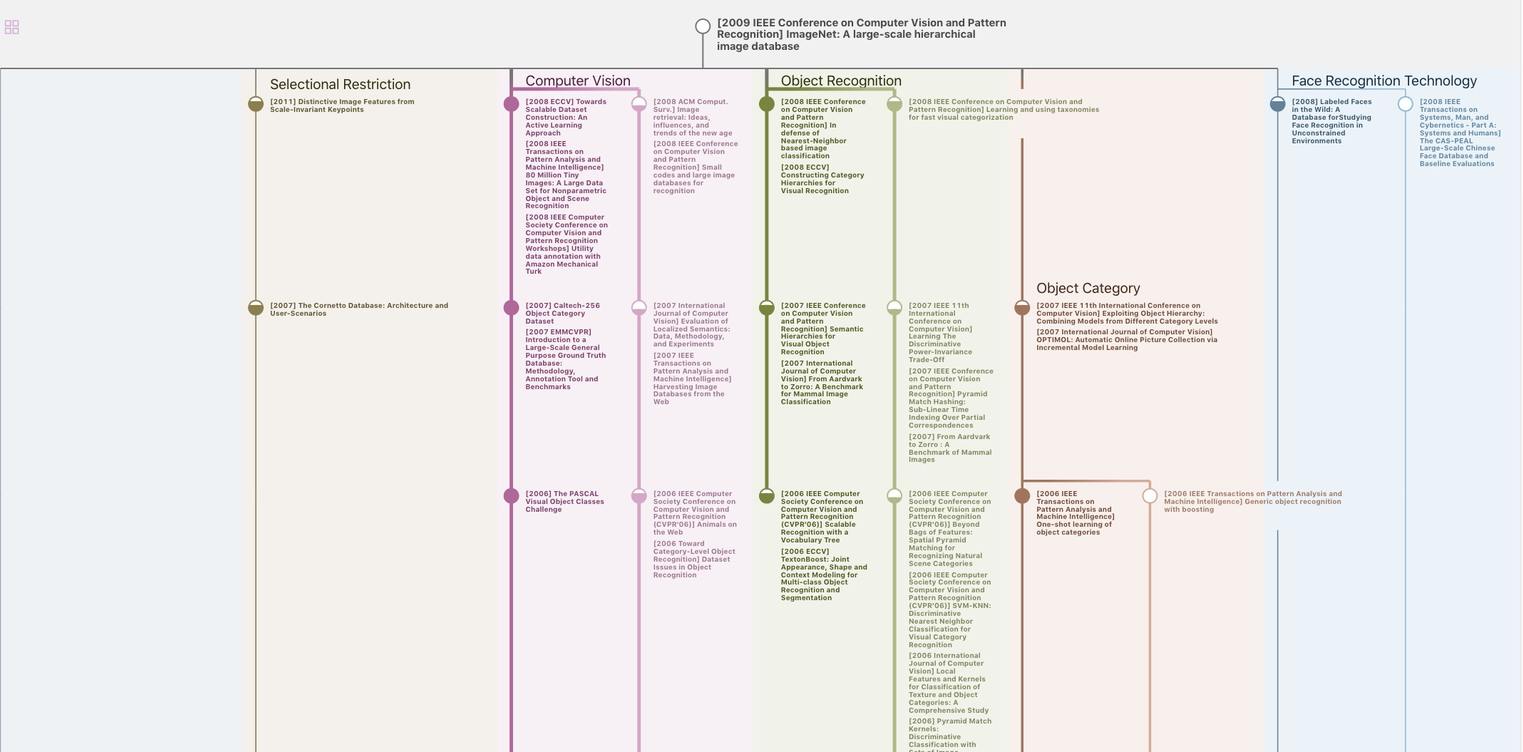
生成溯源树,研究论文发展脉络
Chat Paper
正在生成论文摘要