3D-Aided Dual-Agent GANs for Unconstrained Face Recognition.
IEEE transactions on pattern analysis and machine intelligence(2019)
摘要
In this paper, we propose a Dual-Agent Generative Adversarial Network (DA-GAN) model, which can improve realism of a face simulator's output using unlabeled real faces, while preserving identity information during realism refinement. The dual agents are specifically designed for distinguishing real v.s. fake and identities simultaneously. In particular, we employ an off-the-shelf 3D face model as a simulator to generate profile face images with varying poses. DA-GAN leverages a FCN as the generator and an auto-encoder as the discriminator with dual agents. Besides the novel architecture, we make several key modifications to the standard GAN to preserve pose and texture, preserve identity and stabilize training process: (i) a pose perception loss; (ii) an identity perception loss; (iii) an adversarial loss with a boundary equilibrium regularization term. Experimental results show that DA-GAN not only achieves outstanding perceptual results but also significantly outperforms state-of-the-arts on the challenging NIST IJB-A and CFP unconstrained face recognition benchmarks. In addition, the proposed DA-GAN is also a promising new approach for solving generic transfer learning problems more effectively. DA-GAN is the foundation of our winning in the NIST IJB-A face recognition competition in which we secured the 1st places on the tracks of verification and identification.
更多查看译文
关键词
Face,Face recognition,Gallium nitride,Training,Three-dimensional displays,Generators,Solid modeling
AI 理解论文
溯源树
样例
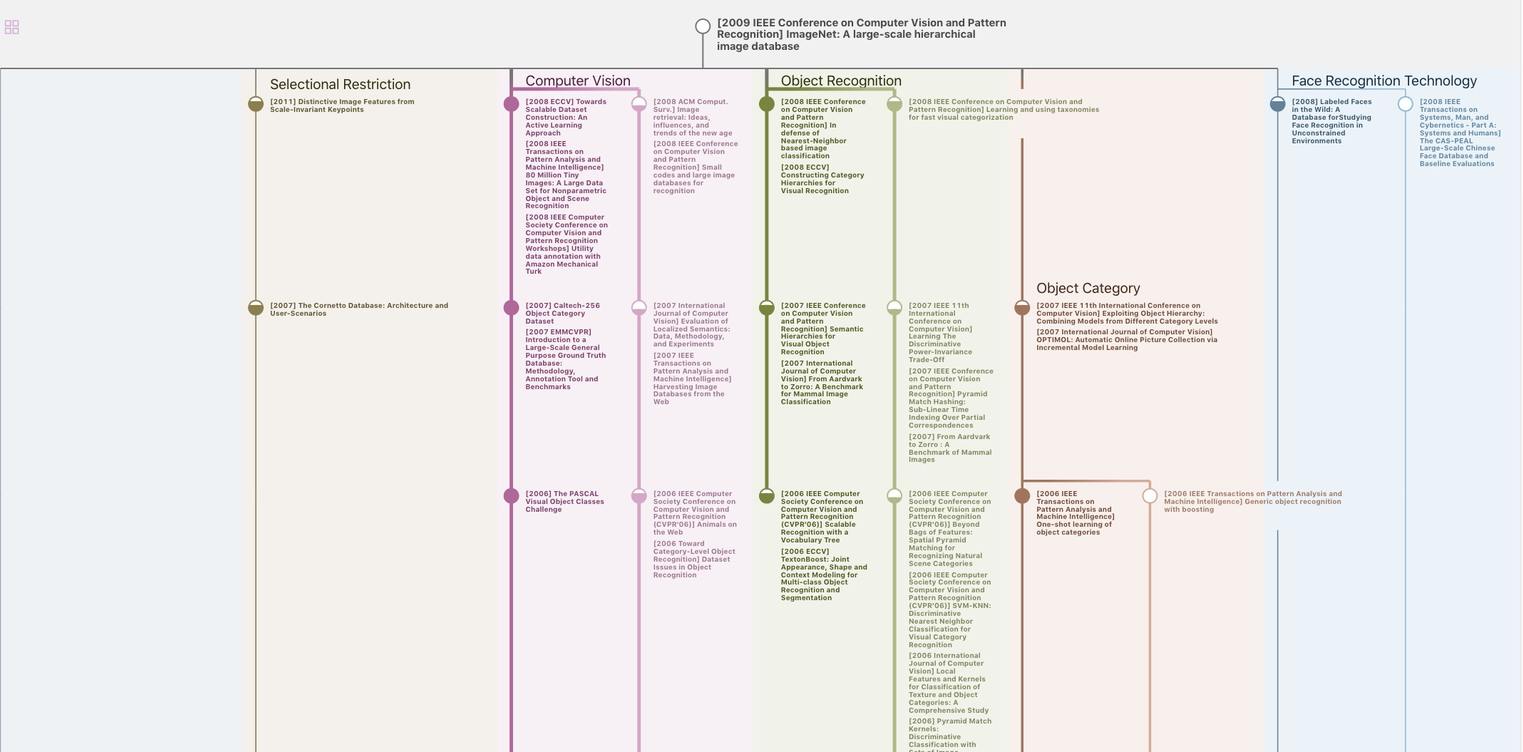
生成溯源树,研究论文发展脉络
Chat Paper
正在生成论文摘要