$\mathcal{DBSDA}$ : Lowering the Bound of Misclassification Rate for Sparse Linear Discriminant Analysis via Model Debiasing
IEEE Transactions on Neural Networks and Learning Systems(2019)
摘要
Linear discriminant analysis (LDA) is a well-known technique for linear classification, feature extraction, and dimension reduction. To improve the accuracy of LDA under the high dimension low sample size (HDLSS) settings, shrunken estimators, such as Graphical Lasso, can be used to strike a balance between biases and variances. Although the estimator with induced sparsity obtains a faster converg...
更多查看译文
关键词
Covariance matrices,Analytical models,Upper bound,Convergence,Estimation,Linear discriminant analysis,Sociology
AI 理解论文
溯源树
样例
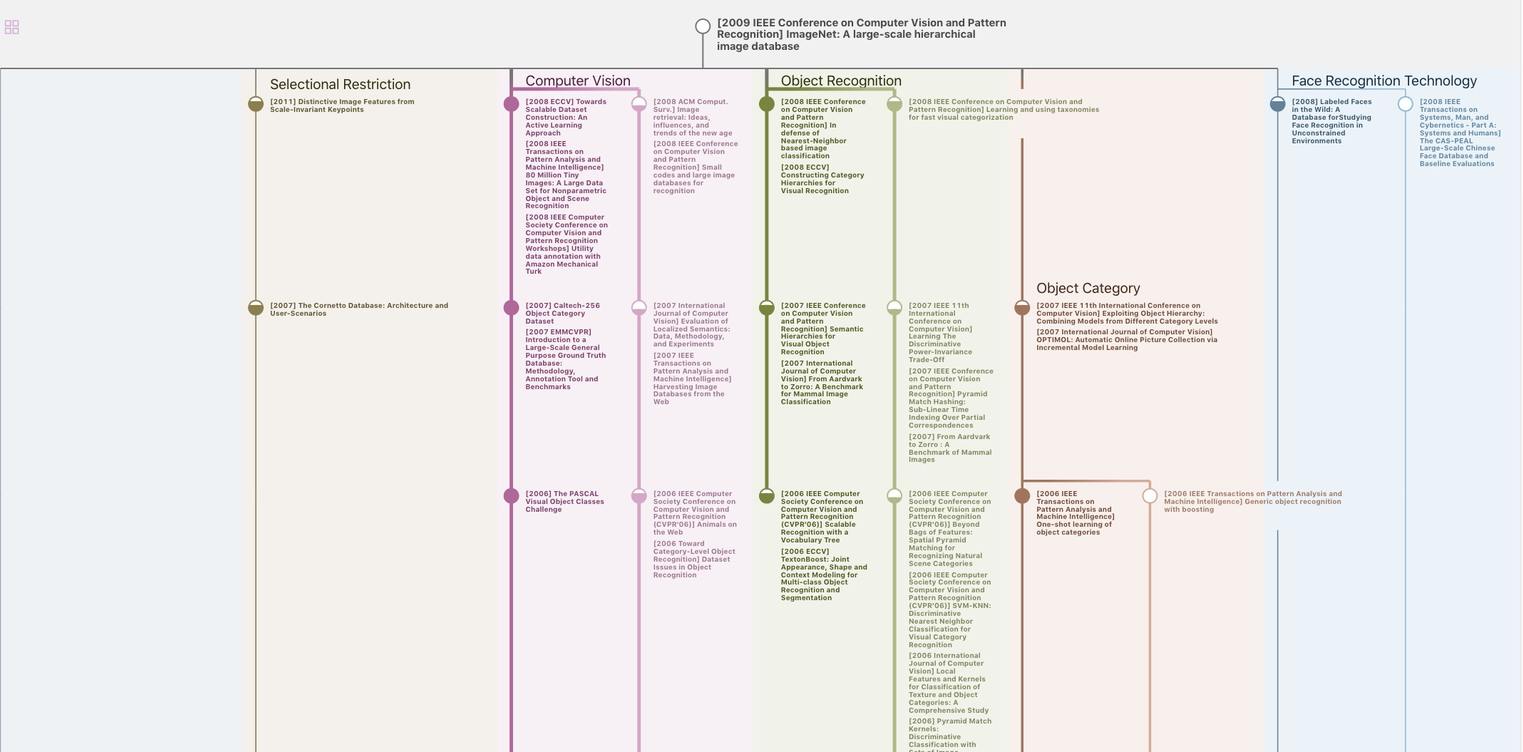
生成溯源树,研究论文发展脉络
Chat Paper
正在生成论文摘要