Relations Between Epidermal Barrier Dysregulation and Staphylococcus Species-Dominated Microbiome Dysbiosis in Patients with Atopic Dermatitis
Journal of Allergy and Clinical Immunology(2018)
摘要
Atopic dermatitis (AD) is an inflammatory skin disease that affects 25% of children and 10% of adults,1Brown S.J. Molecular mechanisms in atopic eczema: insights gained from genetic studies.J Pathol. 2017; 241: 140-145Crossref PubMed Scopus (26) Google Scholar with an increasing prevalence in industrialized countries and a higher risk of allergic rhinitis and asthma later in life.2Spergel J.M. Epidemiology of atopic dermatitis and atopic march in children.Immunol Allergy Clin North Am. 2010; 30: 269-280Abstract Full Text Full Text PDF PubMed Scopus (190) Google Scholar Genetic factors, such as filaggrin-null mutations, as well as environmental factors, such as the skin microbiota, have been described as risk factors for AD.3Werfel T. Allam J.P. Biedermann T. Eyerich K. Gilles S. Guttman-Yassky E. et al.Cellular and molecular immunologic mechanisms in patients with atopic dermatitis.J Allergy Clin Immunol. 2016; 138: 336-349Abstract Full Text Full Text PDF PubMed Scopus (381) Google Scholar, 4Czarnowicki T. Krueger J.G. Guttman-Yassky E. Novel concepts of prevention and treatment of atopic dermatitis through barrier and immune manipulations with implications for the atopic march.J Allergy Clin Immunol. 2017; 139: 1723-1734Abstract Full Text Full Text PDF PubMed Scopus (165) Google Scholar Reduction of microbiome diversity, accompanied by an increase in Staphylococcus aureus abundance, as well as S aureus enterotoxins and exotoxins, have been shown to correlate with disease severity.5Gong J.Q. Lin L. Lin T. Hao F. Zeng F.Q. Bi Z.G. et al.Skin colonization by Staphylococcus aureus in patients with eczema and atopic dermatitis and relevant combined topical therapy: a double-blind multicentre randomized controlled trial.Br J Dermatol. 2006; 155: 680-687Crossref PubMed Scopus (235) Google Scholar Furthermore, epidermal barrier dysfunction is an essential factor related to the pathogenesis of AD. Previous studies of skin of patients with AD have shown disruption of the skin barrier (eg, filaggrin, claudin-1, claudin-4, and claudin-23) in terms of both gene expression and protein localization.6Esaki H. Ewald D.A. Ungar B. Rozenblit M. Zheng X. Xu H. et al.Identification of novel immune and barrier genes in atopic dermatitis by means of laser capture microdissection.J Allergy Clin Immunol. 2015; 135: 153-163Abstract Full Text Full Text PDF PubMed Scopus (139) Google Scholar In this study we pursued a “joint-omics” approach, aiming to uncover possible correlations between the skin microbiome and the skin transcriptome in the context of AD. Gene expression (RNA sequencing [RNAseq]) analysis was performed on matched lesional and nonlesional skin tissue biopsy specimens from patients with AD and healthy subjects. In parallel, 16S DNA sequencing and microbiome analysis were performed on skin swabs collected from the same place in the same patients with AD and healthy subjects (see workflow and detailed patient and sample information in Fig E1 and Table E1 in this article's Online Repository at www.jacionline.org). Tissue biopsy specimens from patients with AD were taken from an upper-arm lesion and a nonlesional site located in close proximity but at least 10 cm away from the lesion and from the upper arm and/or lower back region from healthy subjects. β-Diversity analysis (multidimensional scaling) of the global skin microbiome shows the healthy microbiome to cluster separately from the AD microbiome, with no significant difference between lesional and nonlesional skin (Fig 1, A). On the other hand, β-diversity analysis (principal component analysis [PCA]) of the global skin transcriptome shows that skin samples from patients with lesional AD cluster separately compared with samples from patients with nonlesional AD and those from healthy skin (Fig 1, B). Neither the microbiome nor transcriptomic differences were dependent on sex or age variables. More detailed hierarchical clustering and 3-dimensional PCA and nonmetric multidimensional scaling (NMDS) analyses of both data sets are shown in Fig E2 in this article's Online Repository at www.jacionline.org. Global analysis of microbiome distribution reveals that Staphylococcaceae is the only family with a significantly increased frequency in patients with AD compared with healthy subjects and a significantly greater frequency in lesional compared with nonlesional skin (Fig 1, C). S aureus is the most abundant operational taxonomic unit (OTU) in AD skin and is significantly greater in lesional compared with nonlesional skin. However, S aureus is found in only 40% of the healthy samples with a significantly lower frequency. In contrast, Staphylococcus epidermidis has significantly greater frequency in nonlesional compared with lesional skin and a lower frequency in healthy skin samples. We observed that S aureus and S epidermidis are significantly negatively correlated with each other in lesional skin and for all AD samples (see Fig E3, C, in this article's Online Repository at www.jacionline.org). In general, in the samples from patients with AD, S aureus is significantly negatively correlated with the 4 other most abundant Staphylococcus species, which show positive correlation with each other (see Fig E3, D). Analysis of the 7 most abundant Staphylococcus species frequencies in skin is shown in Fig E3, Table E2, and the Results section in this article's Online Repository at www.jacionline.org. Global skin transcriptome Gene Ontology and Kyoto Encyclopedia of Genes and Genomes analyses based on the 2000 most significant lesional versus nonlesional differentially expressed genes, revealed that cell adhesion, cadherin signaling, and keratinization are among the most differentially expressed gene groups in patients with AD (Fig 1, D, and see Table E3 in this article's Online Repository at www.jacionline.org). Therefore we analyzed differential expression of the 5 skin barrier gene families (see Figs E4 and E5 and the Results section in this article's Online Repository at www.jacionline.org): tight junctions (TJs), stratum corneum (SC), gap junctions (GJs), desmosomes, and adherens junctions (AJs). Overall, the data show a significant downregulation of major barrier molecules in AD lesions together with initiation of a healing process for certain keratins, GJs, desmosomes, and AJs (details in Figs E4 and E5). Finally, we correlated the frequency of the various Staphylococcus species on the skin with expression levels of the various skin barrier genes in each sample. To avoid false discovery of correlations caused by the large number of tests performed, we used a shuffling bootstrap approach to test the robustness of the correlations (see details in the Methods section in this article's Online Repository at www.jacionline.org). Only correlations with ρ = 1 and P < .017 had probability of greater than 99% to be real according to the bootstrap results and were defined here as multiple testing corrected (MTC) correlations. Of the 135 skin barrier genes tested, only 2 TJ genes were proved to have MTC correlations with more than 1 Staphylococcus species. Both CLDN4 and TJP1 gene expression levels were negatively MTC correlated with S aureus and positively MTC correlated with S epidermidis in lesional samples but not in nonlesional samples (Fig 2, A-D). In addition, an MTC-positive correlation was also found between Staphylococcus haemolyticus and CLDN4 and TJP1 and between Staphylococcus hominis and CLDN5 in lesional samples (Fig 2, E-G). Interestingly, all 4 major Staphylococcus species were correlated (S aureus negatively and S epidermidis, S hominis, and S haemolyticus positively) with 2 couples of related genes (CLDN4 and CLDN5; TJP1 and TJP2) either by the abovementioned MTC correlations or by a non-MTC significant correlation (ρ > 0.8 and P ≤ .05: Fig 2, E-H). Lastly, 6 additional MTC correlations were identified between single Staphylococcus species and other TJ genes (see Table E4 in this article's Online Repository at www.jacionline.org). The main result of this study is the correlation between the Staphylococcus species–dominated dysbiosis in the skin microbiome with dysregulation of the skin barrier transcriptome in patients with AD. In particular, there was a correlation in lesional skin, but not nonlesional skin, among all 4 major Staphylococcus species (S aureus negatively and S epidermidis, S hominis, and S haemolyticus positively) and only 4 TJ genes (CLDN4, CLDN5, TJP1, and TJP2). Because correlations do not necessarily imply a causative relation, we cannot make definitive conclusions on whether the microbiome dysbiosis is the cause for or result of the skin barrier defect.7Williams M.R. Gallo R.L. The role of the skin microbiome in atopic dermatitis.Curr Allergy Asthma Rep. 2015; 15: 65Crossref PubMed Scopus (149) Google Scholar All 5 skin barrier gene families show significant expression changes in lesional and nonlesional AD skin, which is consistent with previous publications.8Suarez-Farinas M. Ungar B. Correa da Rosa J. Ewald D.A. Rozenblit M. Gonzalez J. et al.RNA sequencing atopic dermatitis transcriptome profiling provides insights into novel disease mechanisms with potential therapeutic implications.J Allergy Clin Immunol. 2015; 135: 1218-1227Abstract Full Text Full Text PDF PubMed Scopus (178) Google Scholar However, we find a correlation with staphylococci abundance with RNA expression of only 4 TJ genes, possibly because claudin-4 and zonula occludens-1 (ZO-1) (the protein product of TJP1) are expressed at the surface of the top epidermis layer.6Esaki H. Ewald D.A. Ungar B. Rozenblit M. Zheng X. Xu H. et al.Identification of novel immune and barrier genes in atopic dermatitis by means of laser capture microdissection.J Allergy Clin Immunol. 2015; 135: 153-163Abstract Full Text Full Text PDF PubMed Scopus (139) Google Scholar An alternative hypothesis would be that changes in the most highly expressed proteins (eg, CLDN1 and OCLN) could still leave sufficient levels of that specific protein, whereas changes in levels of proteins with medium-level expression (eg, claudin-4/5 and ZO-1/2) could produce relatively low protein levels, thus giving rise to a significant relation with staphylococci abundance. The last possible explanation is that S aureus has a specific relation with these 4 TJ proteins, as observed for claudin-4.9Clark R.T. Hope A. Lopez-Fraga M. Schiller N. Lo D.D. Bacterial particle endocytosis by epithelial cells is selective and enhanced by tumor necrosis factor receptor ligands.Clin Vaccine Immunol. 2009; 16: 397-407Crossref PubMed Scopus (12) Google Scholar In summary, this study allowed us to better characterize concomitantly the state of microbiome and epidermal barrier dysregulation in AD skin. We identified 4 TJ genes with expression that is robustly correlated to the microbiome dysbiosis in patients with AD. A larger validation study is needed to confirm our results, and further proteomics and functional studies are needed to elucidate the role these genes play in relation to changes in Staphylococcus species and skin barrier dysfunction in general. As a general conclusion, we postulate that the explanation for AD flare development and exacerbation is a complex interaction between the skin microbiota, barrier, and immune system. Interaction points between different components of human skin might be the key to development of therapeutic approaches to allergic skin diseases. The Ethical Committee of the Technical University of Munich approved all human subject recruitment and sample collection. Exclusion criteria included self-reported antibiotic treatment (oral or systemic) 6 months before enrollment. Subjects were included only if no topical steroids or other treatment was used in the last 4 weeks before sampling. Additionally, patients were instructed not to use hand sanitizers, antimicrobial soaps, and skin care products and not to shower at least 12 hours before the sample collection appointment. A total of 13 chronic-phase patients with AD with a lesion in the upper arm (mean SCORAD score, 57.3; range, 32-99.5) and 7 healthy control subjects were included in the study (Fig E1 and see characteristics in Table E1). Both swabs for microbiome analysis and 4-mm biopsy specimens for transcriptomics analysis were taken from the middle of the lesion in the area of the upper arm area and from an adjacent nonlesion area with at least 10 cm distance to the outer shape of the visible lesion. Swabs and biopsy specimens from healthy donors were taken from the upper arm and/or lower back region, with no significant difference in staphylococci frequencies. Cutaneous swabs (Sigma-Swab, Medical Wire; Corsham, Wiltshire, United Kingdom) were collected, as described previously,E1Kong H.H. Andersson B. Clavel T. Common J.E. Jackson S.A. Olson N.D. et al.Performing skin microbiome research: a method to the madness.J Invest Dermatol. 2017; 137: 561-568Abstract Full Text Full Text PDF PubMed Scopus (123) Google Scholar, E2Grice E.A. Kong H.H. Conlan S. Deming C.B. Davis J. Young A.C. et al.Topographical and temporal diversity of the human skin microbiome.Science. 2009; 324: 1190-1192Crossref PubMed Scopus (1840) Google Scholar and stored at −80°C. For DNA extraction, swabs were transferred to a bead-beating tube with 500 μg of 0.1-mm zirconium glass beads (Carl Roth, Karlsruhe, Germany), and the QIAamp UCP Pathogen Kit (Qiagen, Hilden, Germany) was used. The original tube was rinsed with 650 μL of lysis buffer, which was then transferred to the swab tube. Samples were incubated for 10 minutes at 56°C with continuous shaking and mechanically lysed with FastPrep (MP Biomedicals, Santa Ana, Calif) twice for 20 seconds at 4.0 m/s, with a 1-minute break between runs. The lysate was processed according to the QIAamp UCP Pathogen Mini Kit preparation protocol. DNA samples were amplified by using variable regions 1 to 3 of the 16S RNA gene to be able to separate different Staphylococcus species.E3Conlan S. Kong H.H. Segre J.A. Species-level analysis of DNA sequence data from the NIH Human Microbiome Project.PLoS One. 2012; 7: e47075Crossref PubMed Scopus (118) Google Scholar All samples were equimolarly pooled into one library by using 2 multiplexing barcodes per samples. Bidirectional sequencing with a MiSeq Nano v3 (Illumina, San Diego, Calif) resulted in 2× 300-bp paired-end reads. At each sampling, swab controls without any skin contact were processed parallel to experimental samples. No significant background contamination was observed from either reagents, collection procedures, or both. Primary microbiome data analysis, including signal processing, demultiplexing, and trimming of adapter sequences, was performed by using MiSeq Reporter 2.5.0.5 software. Thereafter, the bioinformatics software tool CLC Genomics Workbench 8.5.1 with the Microbial Genomics Module (Qiagen) was used for quality trimming, merging, and clustering. OTUs were clustered at a 97% identity threshold, according to the taxonomy of the SILVA 16S rRNA sequence database (www.arb-silva.de). OTUs of interest were reidentified by using EzBioCloud for similarity-based searches against quality-controlled databases of 16S rRNA sequences to identify specific species, especially Staphylococcus species.E4Yoon S.H. Ha S.M. Kwon S. Lim J. Kim Y. Seo H. et al.Introducing EzBioCloud: a taxonomically united database of 16S rRNA and whole genome assemblies.Int J Syst Evol Microbiol. 2017; 67: 1613-1617Crossref PubMed Scopus (4302) Google Scholar Punch biopsy specimens (4 mm) were immediately transferred in RNAlater solution (Life Technologies, Carlsbad, Calif) at 4°C overnight and thereafter stored at −80°C. Total RNA was prepared from whole skin tissue by using the RNeasy Mini Kit (Qiagen). Quantity and quality of the isolated RNAs were determined with a Qubit (1.0) Fluorometer (Life Technologies) and a 2100 Bioanalyzer (Agilent, Waldbronn, Germany), and samples with RNA integrity numbers of greater than 7.0 were chosen for sequencing. Library preparation for RNAseq was performed with the TruSeq Stranded mRNA Sample Prep Kit (Illumina), and the Illumina HiSeq 2500 single end 126 or 101 bp (Illumina) was used for sequencing. All samples were amplified with the Primers 8F-YM (5′-AGAGTTTGATYMTGGCTCAG-3′) and 517R (5′-ATTACCGCGGCTGCTGG-3′).E5Li B. Dewey C.N. RSEM: accurate transcript quantification from RNA-Seq data with or without a reference genome.BMC Bioinformatics. 2011; 12: 323Crossref PubMed Scopus (10803) Google Scholar The amplicons cover the variable regions 1 to 3 of the 16S RNA gene. Each amplicon was purified separately, applying solid-phase reversible immobilization paramagnetic bead-based technology (AMPure XP beads; Beckman Coulter, Fullerton, Calif) with a bead/DNA ratio of 0.7:1 (vol/vol), according to the manufacturer's instructions. Normalization of each amplification product to approximately 1 ng/μL DNA was achieved by using the SequalPrep Kit (Life Technologies). A library was generated containing each of the amplicons from the 16S PCRs. Additionally, 2 positive controls and 2 negative controls were also added to the pool. All samples except the controls were pooled equimolarly to form one library. The High Sensitivity DNA LabChip Kit (Agilent Technologies) was used on the 2100 Bioanalyzer (Agilent Technologies) to analyze the quality of the purified amplicon library. Bidirectional sequencing with an MiSeq Reagent Kit 600-cycles Nano v3 (Illumina) results in reads of 300 bases in both directions, totaling 600 bases of sequence information in 2× 300-bp paired-end reads. The Illumina software MiSeq Reporter 2.5.0.5 on the MiSeq system and the Illumina Sequence Analysis Viewer 1.9.1 were used for imaging and evaluation of the sequencing run performance. Primary microbiome data analysis, including signal processing, demultiplexing, and trimming of adapter sequences of the 2 read files was performed with the MiSeq inherited MiSeq Reporter 2.5.0.5 software. Furthermore, data were imported in the bioinformatics software tool CLC Genomics Workbench 8.5.1 (Qiagen) for quality trimming, merging of reads 1 and 2 from each cluster, and quality control report generation. Clustering (OTU definition) was also performed with the CLC plugin Microbial Genomics Module. Demultiplexed raw data (reads 1 and 2 per sample) were imported into CLC Genomics Workbench as paired-end (forward-reverse) reads. A possible distance between 1 and 10,000 bases was allowed. Failed reads were removed from the data set during this process. As a first step, read 1 and 2 sequences from every sample were merged by using the overlapping sequence information and taking the minimum overlap, potential mismatches, gaps, and unaligned ends into account. The 2 reads were merged, including a minimum of 20-bp overlaps without mismatch and maximum unaligned end mismatches of 2 bp. Read trimming was performed according to primer sequences, base quality, and read length, whereby a probability quality limit of .05 was applied. Sequences of less than 400 bp were discarded to guarantee similarity and a sufficiently high level of sequence information for phylogenetic classification, and longer sequences were trimmed down to this length. The remaining sequences were clustered at a 97% identity threshold defining OTUs according to the taxonomy of the SILVA 16S rRNA sequence database (www.arb-silva.de). Chimeric sequences, representing PCR and sequencing artifacts, were filtered out and discarded during this step. Library preparation for RNAseq was performed by using the TruSeq Stranded mRNA Sample Prep Kit (Illumina). Total RNA samples (1 μg) were ribosome depleted and then reverse transcribed into double-stranded cDNA, with actinomycin added during first-strand synthesis. The cDNA samples were fragmented, end-repaired, and polyadenylated before ligation of TruSeq adapters. The adapters contain the index for multiplexing. Fragments containing TruSeq adapters on both ends were selectively enriched with PCR. The quality and quantity of the enriched libraries were validated by using the Qubit (1.0) Fluorometer and the Bioanalyzer 2100 (Agilent). The product is a smear with an average fragment size of approximately 360 bp. Libraries were normalized to 10 nmol/L in 10 mmol/L Tris-Cl, pH 8.5, with 0.1% Tween 20. The TruSeq SR-/TruSeq PE Cluster Kit v4-cBot-HS (Illumina) was used for cluster generation by using 8 pmol/L of pooled normalized libraries on the cBOT. Sequencing was performed on the Illumina HiSeq 2500 paired end at 2× 126 bp or single end 1at 26 bp with the TruSeq SBS Kit v4-HS (Illumina). Sequencing images were transformed with Illumina Basecaller software to bcl files and demultiplexed to FASTQ files with CASAVA v1.8.2 software (Illumina). Quality check on the reads was performed with FastQC (version 0.10.0; Babraham Institute, Cambridge, United Kingdom). Raw sequencing reads were mapped to the Homo sapiens genome (GRCh38.p7 build) by using RSEM (v1.2.12)E6Klindworth A. Pruesse E. Schweer T. Peplies J. Quast C. Horn M. et al.Evaluation of general 16S ribosomal RNA gene PCR primers for classical and next-generation sequencing-based diversity studies.Nucleic Acids Res. 2013; 41: e1Crossref PubMed Scopus (4407) Google Scholar implementation of Bowtie software (version 1.0.0)E7Langmead B. Trapnell C. Pop M. Salzberg S.L. Ultrafast and memory-efficient alignment of short DNA sequences to the human genome.Genome Biol. 2009; 10: R25Crossref PubMed Scopus (14997) Google Scholar alignment program with the Ensemble annotation (version 75). A number of microbiome samples were excluded (see details in Table E1 and Fig E1) from analysis caused by technical problems or low sequencing depth (<1000 reads), resulting in 20 samples in the analysis that show no significant difference in sequencing depth between the 3 skin groups and no dependence of the number of OTUs observed as a function on sequencing depth (Fig E4). After a normalized OTU table was obtained, the α-diversity of the global microbiome was assessed for each sample by using a number of methods: richness, inverse Simpson index, and normalized Shannon entropy. In addition, we analyzed the frequencies of the most abundant families and species and of specific species of interest (eg, staphylococci). For each OTU, species, and family, we also characterized the span (percentage detectable samples) over all the samples and, more importantly, over each subgroup of samples (ie, lesional, nonlesional, and healthy). β-Diversity analysis of the global microbiome was performed by using multidimensional scaling and hierarchical trees to look for differences between subgroups of patients using the RHEA R-script package.E8Lagkouvardos I. Fischer S. Kumar N. Clavel T. Rhea: a transparent and modular R pipeline for microbial profiling based on 16S rRNA gene amplicons.PeerJ. 2017; 5: 2836https://doi.org/10.7717/peerj.2836Crossref PubMed Scopus (187) Google Scholar The more detailed analysis of Staphylococcaceae and their correlation with the transcriptome was performed on 22 (of 54) Staphylococcus species–annotated OTUs that had a minimum total abundance of 30 reads and minimum span of 25% over all samples. A number of transcriptome samples were excluded (see Table E1 and Fig E1) from analysis because of technical problems or low RNA yield, resulting in 28 samples in the analysis, which show no quality control problems. Gene- and isoform-level abundances were quantified as RPKM values. Clustering analyses were performed with the “ward.D2” clustering algorithm implemented in the “hclust” function of the R statistics package. Heat map plots were performed with the function “heatmap.2” implemented in the gplots R package. Differential expression analysis between groups was performed with the edger R bioconductor package.E9Robinson M.D. McCarthy D.J. Smyth G.K. edgeR: a Bioconductor package for differential expression analysis of digital gene expression data.Bioinformatics. 2010; 26: 139-140Crossref PubMed Scopus (20839) Google Scholar More detailed analysis and correlations tested with the microbiome for the 135 skin barrier genes that were differentially expressed between nonlesional versus lesional skin or between healthy versus nonlesional skin with a false discovery rate of less than 0.01. Because of the extensive multiple testing involved in correlating microbiome data with transcriptome data, we hook a bootstrap approach to correct for false discovery of correlations. Even after focusing on the Staphylococcus species (n = 7) and skin barrier genes (n = 135), 945 correlation tests were performed for each skin group. We identified 63 (6.7%) potentially significant (P < .05) correlations, most of them between sporadic Staphylococcus species and sporadic genes. To verify these correlations, we used a bootstrap approach, in which the transcriptome results were randomly shuffled across samples to create 100 random sets of data in which less than 20% of the gene expression levels corresponded to the appropriate microbiome frequency of the same original sample. This process was performed separately for each combination of data sets being correlated (eg, correlating Staphylococcus species with TJ genes and with GJ genes) to maintain the original structure of the data. This approach revealed that an average of 71 (7.5%) correlations in the bootstrap set was seemingly significant (P < .05) but actually arose randomly in the shuffled bootstrap sets because of multiple testing. Only correlations with ρ = 1 and P < .017 had probability of greater than 99% to be real according to the bootstrap results and were defined as MTC correlations. Only 13 MTC correlations were found (of the initial 63), and these were found only in lesional skin and only from the TJ family (Table E4). Indeed, most of the sporadic correlations were found to be non-MTC, whereas 7 (54%) MTC correlations were found to be associated with 3 genes that had correlation with a number of Staphylococcus species (Table E4). The nonparametric Mann-Whitney (or Kruskal-Wallis) test was used to verify the statistical significance of differences in continuous variables between 2 (or 3) subgroups of samples. The statistical significance of differences in proportions of discrete data between subgroups of samples was tested by using the Fisher exact test. Correlations between continuous variables were assessed by using the nonparametric Spearman test. Significant P values were considered at the 2-tailed level of less than .05 unless correction for multiple testing was needed in which case the false discovery rate results were used or a bootstrap method was used, as described above. In addition to S aureus and S epidermidis, which are the most frequent Staphylococcus species (Table E2), S hominis frequency is similar between samples from patients with nonlesional AD and healthy subjects, which are both significantly greater than those in lesional skin (Fig E3, A). Staphylococcus caprae shows a similar pattern like S hominis, as can be seen by the differential abundance patterns in the heatmap (Fig E3, B). S haemolyticus has the same range of abundance over the 3 skin groups, with a somewhat greater span in samples from healthy subjects versus patients with AD. A significant difference is found also for Staphylococcus warneri, but its abundance is very low. We did not observe a correlation between Staphylococcus species frequencies and either IgE levels or SCORAD scores of the patients. The majority of highly expressed TJ genes are downregulated in lesional skin, among them claudins 1, 3 to 5, 7 to 11, 16, 19, and 23 and CLDND1 (Fig E4). Furthermore, claudin-1, F11r, and occludin are significantly downregulated in nonlesional and lesional skin compared with healthy skin. Conversely, expression of TJ adaptor molecules (eg, MARVELD1, MARVELD2, ZO-1 [TJP-1] and ZO-2 [TJP-2]), which mediate the connection of intercellular junctions to cytoskeletal components, is significantly upregulated in lesional compared with nonlesional skin. From SC genes, filaggrin-1 is significantly downregulated in AD skin, and filaggrin-2 is significantly downregulated in lesional skin (Fig E4). Furthermore, low-level keratins in nail beds and hair follicles (eg keratin 16) were overall upregulated in skin of patients with AD, and keratins of the oral mucosa and esophagus were also upregulated in lesional skin (eg, keratins 6A, 6B, and 6C; Fig E5). Similar expression signature changes were also observed in the GJ and desmosome families, where genes that are expressed at very low levels or constitutively not expressed in healthy skin, such as GJB2, GJB6, DSC2, and DSG3, are significantly upregulated in lesions. However, genes expressed at high levels in healthy skin, such as GJB4, GJC3, and DSG2, are significantly downregulated in nonlesional AD skin, with further downregulation in lesional skin (Fig E5). We did not observe any clustering or dependence of differentially expressed genes as a function of either IgE levels or SCORAD scores of the patients.Fig E2β-Diversity of global skin microbiome and transcriptome. A and B, NMDS clustering of the skin microbiome (Fig E2, A) separates healthy skin samples from those from patients with AD (both lesional and nonlesional AD) and, to a lesser extent, lesional from nonlesional samples, whereas 3-component PCA clustering (Fig E2, B) of skin transcriptome differentiates between all 3 skin groups. PC, Principal component. C-E, Hierarchical clustering of the global skin microbiome (Fig E2, C) separates AD lesional and nonlesional samples from healthy skin, with one exception, whereas for transcriptomics, the clustering of all genes (Fig E2, D) separates lesional samples from the others, but clustering of only the top 100 genes (Fig E2, E) separates all 3 skin groups. HE, Healthy; LS, lesional AD; NL, nonlesional AD.View Large Image Figure ViewerDownload Hi-res image Download (PPT)Fig E3Different frequencies of Staphylococcus species. A and B, Frequencies of Staphylococcus species show different abundance patterns in healthy, lesional AD, and nonlesional AD skin, with only S aureus most abundant in AD lesional samples, whereas S epidermidis is most abundant in nonlesional skin, and the other species are most abundant in healthy skin. C and D, S aureus frequency is negatively correlated with that of S epidermidis and all other staphylococci, which are positively correlated with each other, in lesional skin. Heat map of fold change expression values (FCH) is scaled from blue to red in Fig E3, B, and heat map of correlation coefficients (R) is scaled from orange to green in Fig E3, D. HE, Healthy; LS, lesional AD; NL, nonlesional AD.View Large Image Figure ViewerDownload Hi-res image Download (PPT)Fig E4Differential expression of skin barrier gene families. Epidermal barrier genes are the most significant differentially expressed Kyoto Encyclopedia of Genes and Genomes pathway group in the AD-related transcriptome (Table E3). A and C, Heat map clustering of TJ (Fig E4, A) and SC barrier (Fig E4, C) gene expression shows different patterns of differential expression. B, Most highly expressed TJ genes are significantly downregulated in lesional skin, whereas some TJ genes expressed at lower levels and in particular TJ adaptor genes (eg, TJP1) are upregulated in lesions. D, Among the SC genes, filaggrins are downregulated in AD skin compared with healthy skin and in particular in lesional skin, whereas the keratin genes show a mix of upregulation and downregulation in AD and lesional skin. HE, Healthy; LS, lesional AD; NL, nonlesional AD.View Large Image Figure ViewerDownload Hi-res image Download (PPT)Fig E5Differential expression of skin barrier gene families. A-C, Heat map clustering of gene expression of GJ (Fig E5, A), desmosome (Fig E5, B), and AJ skin barrier genes (Fig E5, C) show a mixed pattern of differential expression. HE, Healthy; LS, lesional AD; NL, nonlesional AD.View Large Image Figure ViewerDownload Hi-res image Download (PPT)Table E1Patients' characteristics and sample availabilityPatientSexAge (y)BMI (kg/m2)SCORAD scoreTotalIgE (KU/l)MicrobiomeTranscriptomeLSNLLSNLAD02M2325.2402240011AD03M3423.850.899831111AD04F4920.4704351101AD05M6719.87966.661111AD06F4645.76617.41111AD07M4615.853272871111AD08M6121.6329.71011AD09F6223.234.37.380111AD10M1822.799.54600111AD11M2824.14963981100AD12M8624.851ND0011AD13F5227.4801840010AD14F2124.84025.50011Total781111Paired LS and NL610Paired microbiome and transcriptome5757HealthySexAge (y)BMI (kg/m2)SCORAD scoreTotalIgEMicrobiomeTranscriptomeHEHEHL01F50310ND01HL02F26220ND01HL03F48340ND11HL04F5023.1017.910HL05F2625.202.7911HL06F3620.8046.811HL07M3130023311Total56Paired microbiome and transcriptome44F, Female; HE, healthy; LS, lesional AD; M, male; ND, no data; NL, nonlesional AD. Open table in a new tab Table E2Staphylococcus species frequencies per AD statusStaphylococcus speciesAll (n = 20)HE (n = 5)AD NL (n = 8; paired = 6)AD LS (n = 7; paired = 6)LS vs NLAD vs HEMean ± SEM frequencySpanMean ± SEM frequencySpanMean ± SEM frequencySpanMean ± SEM frequencySpanPatients with frequency LS > NL (%)§Percentage of patients in which the frequency of the respective Staphylococcus species is greater in the lesional versus nonlesional site.Mean ratio (LS/NL)‖Mean of the ratio of Staphylococcus species abundance in lesional versus nonlesional paired samples.P value,†Wilcoxon signed-rank test (paired). LS vs NLP value,‡Mann-Whitney U test (independent). LS vs HEP value,‡Mann-Whitney U test (independent). NL vs HES aureus38.9% ± 6.7%85%0.5% ± 0.5%40%39.8% ± 7.0%100%65.2% ± 7.5%100%100%2.2.028∗Significant (P < .05)..004∗Significant (P < .05)..003∗Significant (P < .05).S epidermidis5.4% ± 1.0%100%2.8% ± 0.6%100%7.6% ± 1.9%100%4.7% ± 1.7%100%17%0.6.046∗Significant (P < .05)..93.1S hominis2.5% ± 0.8%95%3.8% ± 1.3%100%3.4% ± 1.7%88%0.5% ± 0.2%100%50%0.8.23.018∗Significant (P < .05)..34S haemolyticus1.6% ± 0.5%65%1.8% ± 0.8%80%1.5% ± 1.0%63%1.5% ± 1.0%57%17%0.7.47.41.41S caprae1.0% ± 0.4%80%1.3% ± 0.8%80%1.2% ± 0.6%75%0.6% ± 0.5%86%33%0.7.23.26.77S warneri0.3% ± 0.2%55%1.0% ± 0.7%100%0.1% ± 0.1%50%0%29%17%—.29.003∗Significant (P < .05)..03∗Significant (P < .05).S xylosus0.1% ± 0.1%25%0%20%0.2% ± 0.1%50%0%0%——.18.24.4HE, Healthy; LS, lesional AD; NL, nonlesional AD.∗ Significant (P < .05).† Wilcoxon signed-rank test (paired).‡ Mann-Whitney U test (independent).§ Percentage of patients in which the frequency of the respective Staphylococcus species is greater in the lesional versus nonlesional site.‖ Mean of the ratio of Staphylococcus species abundance in lesional versus nonlesional paired samples. Open table in a new tab Table E3Kyoto Encyclopedia of Genes and Genomes analysis of differentially lesional/nonlesional expressed skin transcriptome pathways and gene familiesTermP valueAdjusted P valueOverlapz ScoreCombined scoreCell adhesion molecules: Homo sapiens–hsa045147.85571 × 10−112.30172 × 10−889/142 (62.6%)−1.72579399630.35157747Cytokine—cytokine receptor interaction— Homo sapiens–hsa040606.38294 × 10−109.351 × 10−8144/265 (54.3%)−1.85309147429.99265139Pathways in cancer—Homo sapiens–hsa052001.13005 × 10−91.10368 × 10−7201/397 (50.6%)−2.05639234232.94226989Chemokine signaling pathway–Homo sapiens–hsa040622.26831 × 10−91.66154 × 10−7107/187 (57.2%)−1.85035184628.88464178Rheumatoid arthritis–Homo sapiens–hsa053231.13405 × 10−76.64551 × 10−657/90 (63.3%)−1.6601897319.7920659AGE-RAGE signaling pathway in diabetic complication–Homo sapiens–hsa049331.69444 × 10−77.03706 × 10−662.101 (61.3%)−1.89055643122.4301672Viral myocarditis–Homo sapiens–hsa054161.63765 × 10−77.03706 × 10−641/59 (69.5%)−1.72583601520.47587141Inflammatory bowel disease–Homo sapiens–hsa053211.92138 × 10−77.03706 × 10−644/65 (67.7%)−1.70281388620.20272949Apoptosis–Homo sapiens–hsa042105.92328 × 10−71.92836 × 10−579/140 (56.4%)−1.75563299919.059604494Focal adhesion–Homo sapiens–hsa045101.06875 × 10−63.13143 × 10−5106/202 (52.4%)−1.69569799117.58682117 Open table in a new tab Table E4List of MTC correlations and their association with other correlations within barrier genes analyzedGeneMTC correlations (R = 1, P = .0167)Correlates withStaphylococcus speciesDirectionStaphylococcus speciesDirectionRP valueCLDN4S aureusNegativeCLDN4S epidermidisPositiveCLDN4S haemolyticusPositiveCLDN4S hominisPositive0.8.13CLDN5S aureusNegative−0.8.13CLDN5S epidermidisPositive0.8.13CLDN5S haemolyticusPositive0.872.05CLDN5S hominisPositiveTJP1S aureusNegativeTJP1S epidermidisPositiveTJP1S haemolyticusPositiveTJP1S hominisPositive0.8.13TJP2S aureusNegative−0.916.03TJP2S epidermidisPositive0.8.13TJP2S haemolyticusPositive0.972.05TJP2S hominisPositive0.872.05AMOTL2S capraePositiveCLDN2S hominisPositiveCLDND2S haemolyticusNegativeJAM2S hominisPositiveJAM3S capraePositiveMPDZS haemolyticusPositive Open table in a new tab F, Female; HE, healthy; LS, lesional AD; M, male; ND, no data; NL, nonlesional AD. HE, Healthy; LS, lesional AD; NL, nonlesional AD.
更多查看译文
关键词
Skin Microbiome,Eczema
AI 理解论文
溯源树
样例
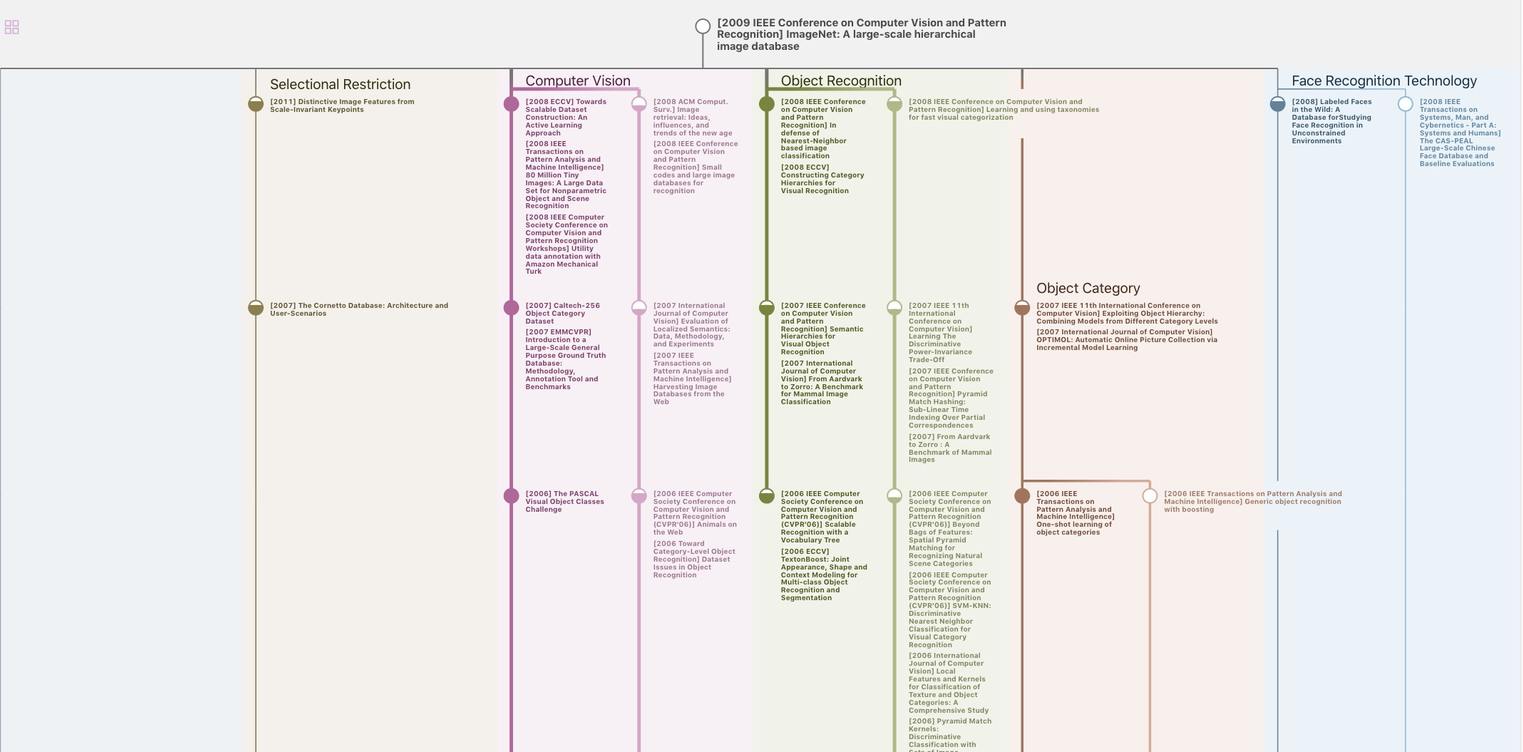
生成溯源树,研究论文发展脉络
Chat Paper
正在生成论文摘要