Semi-supervised Domain Adaptation by Covariance Matching.
IEEE transactions on pattern analysis and machine intelligence(2019)
摘要
Transferring knowledge from a source domain to a target domain by domain adaptation has been an interesting and challenging problem in many machine learning applications. The key problem is how to match the data distributions of the two heterogeneous domains in a proper way such that they can be treated indifferently for learning. We propose a covariance matching approach DACoM for semi-supervised domain adaptation.The DACoM embeds the original samples into a common latent space linearly such that the covariance mismatch of the two mapped distributions is minimized, and the local geometric structure and discriminative information are preserved simultaneously. The KKT conditions of DACoM model are given as a nonlinear eigenvalue equation. We show that the KKT conditions could at least ensure local optimality. An efficient eigen-updating algorithm is then given for solving the nonlinear eigenvalue problem, whose convergence is guaranteed conditionally. To deal with the case when homogeneous information could only be matched nonlinearly, a kernel version of DACoM is further considered.We also analyze the generalization bound for our domain adaptation approaches. Numerical experiments are given to demonstrate the effectiveness and efficiency of the proposed approach. The experiments show that our method outperforms other methods for homogeneous and heterogeneous cases.
更多查看译文
关键词
Kernel,Convergence,Adaptation models,Mathematical model,Eigenvalues and eigenfunctions,Manifolds
AI 理解论文
溯源树
样例
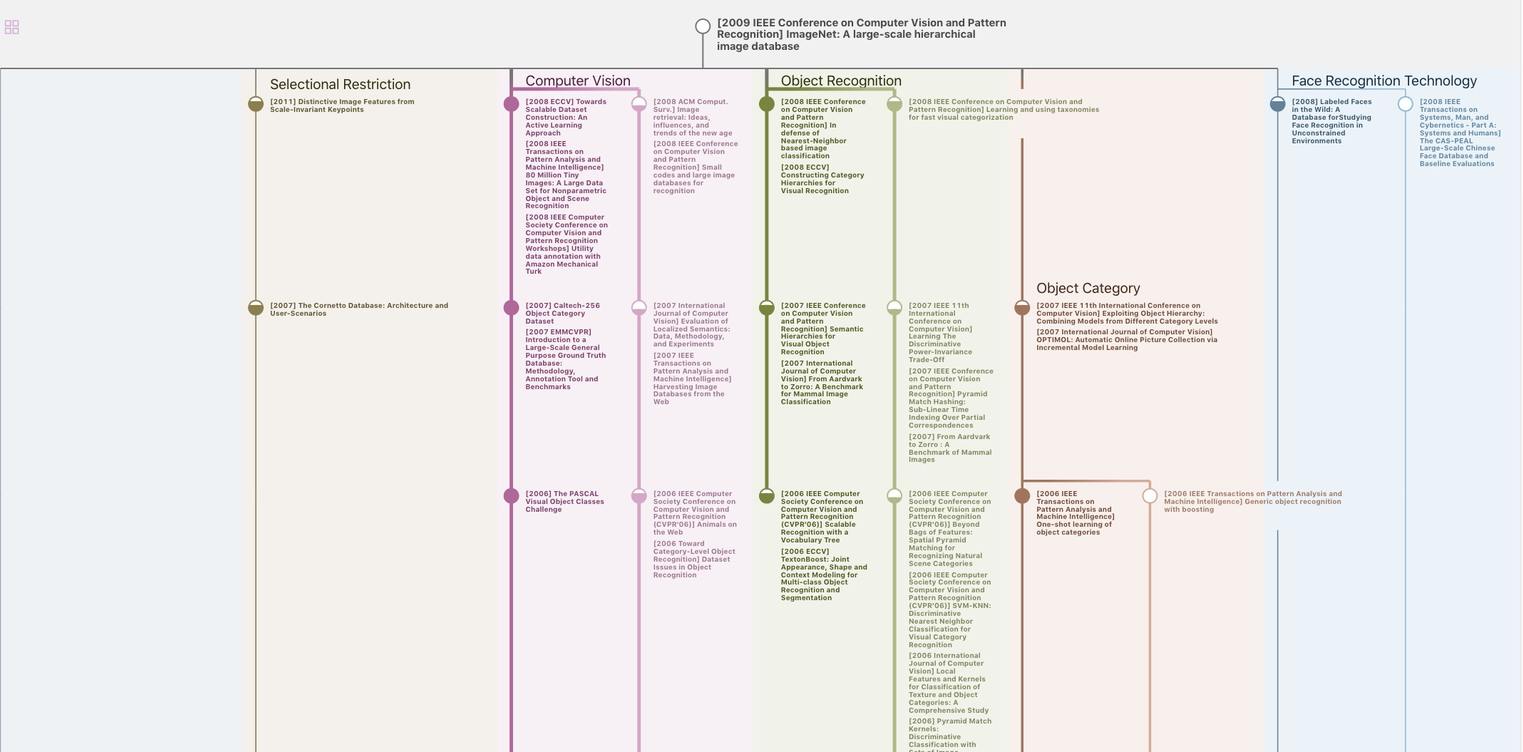
生成溯源树,研究论文发展脉络
Chat Paper
正在生成论文摘要