Selection into medicine: the predictive validity of an outcome-based procedure
BMC medical education(2018)
摘要
Background Medical schools must select students from a large pool of well-qualified applicants. A challenging issue set forward in the broader literature is that of which cognitive and (inter)personal qualities should be measured to predict diverse later performance. To address this gap, we designed a ‘backward chaining’ approach to selection, based on the competences of a ‘good doctor’. Our aim was to examine if this outcome-based selection procedure was predictive of study success in a medical bachelor program. Methods We designed a multi-tool selection procedure, blueprinted to the CanMEDS competency framework. The relationship between performance at selection and later study success across a three-year bachelor program was examined in three cohorts. Study results were compared between selection-positive and selection-negative (i.e. primarily rejected) students. Results Selection-positive students outperformed their selection-negative counterparts throughout the entire bachelor program on assessments measuring cognitive (e.g. written exams), (inter)personal and combined outcomes (i.e. OSCEs). Of the 30 outcome variables, selection-positive students scored significantly higher in 11 cases. Fifteen other, non-significant between-group differences were also in favor of the selection-positives. An overall comparison using a sign test indicated a significant difference between both groups ( p < 0.001), despite equal pre-university GPAs. Conclusions The use of an outcome-based selection approach seems to address some of the predictive validity limitations of commonly-used selection tools. Selection-positive students significantly outperformed their selection-negative counterparts across a range of cognitive, (inter)personal, and mixed outcomes throughout the entire three-year bachelor in medicine.
更多查看译文
关键词
Selection,Backward chaining,Outcome-based,Predictive validity
AI 理解论文
溯源树
样例
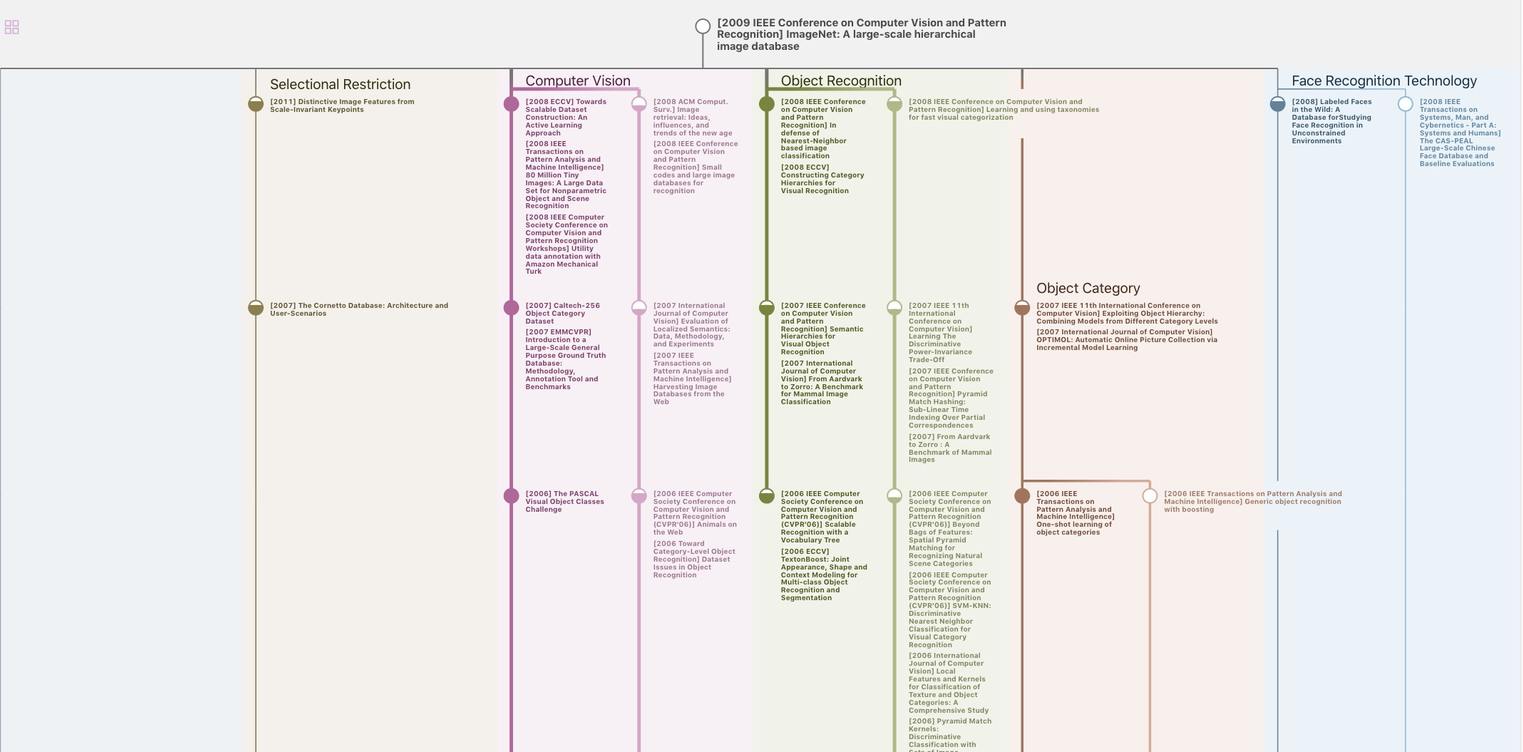
生成溯源树,研究论文发展脉络
Chat Paper
正在生成论文摘要