Pattern to Knowledge: Deep Knowledge-Directed Machine Learning for Residue-Residue Interaction Prediction
SCIENTIFIC REPORTS(2018)
摘要
Residue-residue close contact (R2R-C) data procured from three-dimensional protein-protein interaction (PPI) experiments is currently used for predicting residue-residue interaction (R2R-I) in PPI. However, due to complex physiochemical environments, R2R-I incidences, facilitated by multiple factors, are usually entangled in the source environment and masked in the acquired data. Here we present a novel method, P2K (Pattern to Knowledge), to disentangle R2R-I patterns and render much succinct discriminative information expressed in different specific R2R-I statistical/functional spaces. Since such knowledge is not visible in the data acquired, we refer to it as deep knowledge. Leveraging the deep knowledge discovered to construct machine learning models for sequence-based R2R-I prediction, without trial-and-error combination of the features over external knowledge of sequences, our R2R-I predictor was validated for its effectiveness under stringent leave-one-complex-out-alone cross-validation in a benchmark dataset, and was surprisingly demonstrated to perform better than an existing sequence-based R2R-I predictor by 28% (p: 1.9E-08). P2K is accessible via our web server on https://p2k.uwaterloo.ca .
更多查看译文
关键词
Deep Knowledge,Supplementary Note,Input Protein Sequence,Existing Software Platforms,Random Predictor
AI 理解论文
溯源树
样例
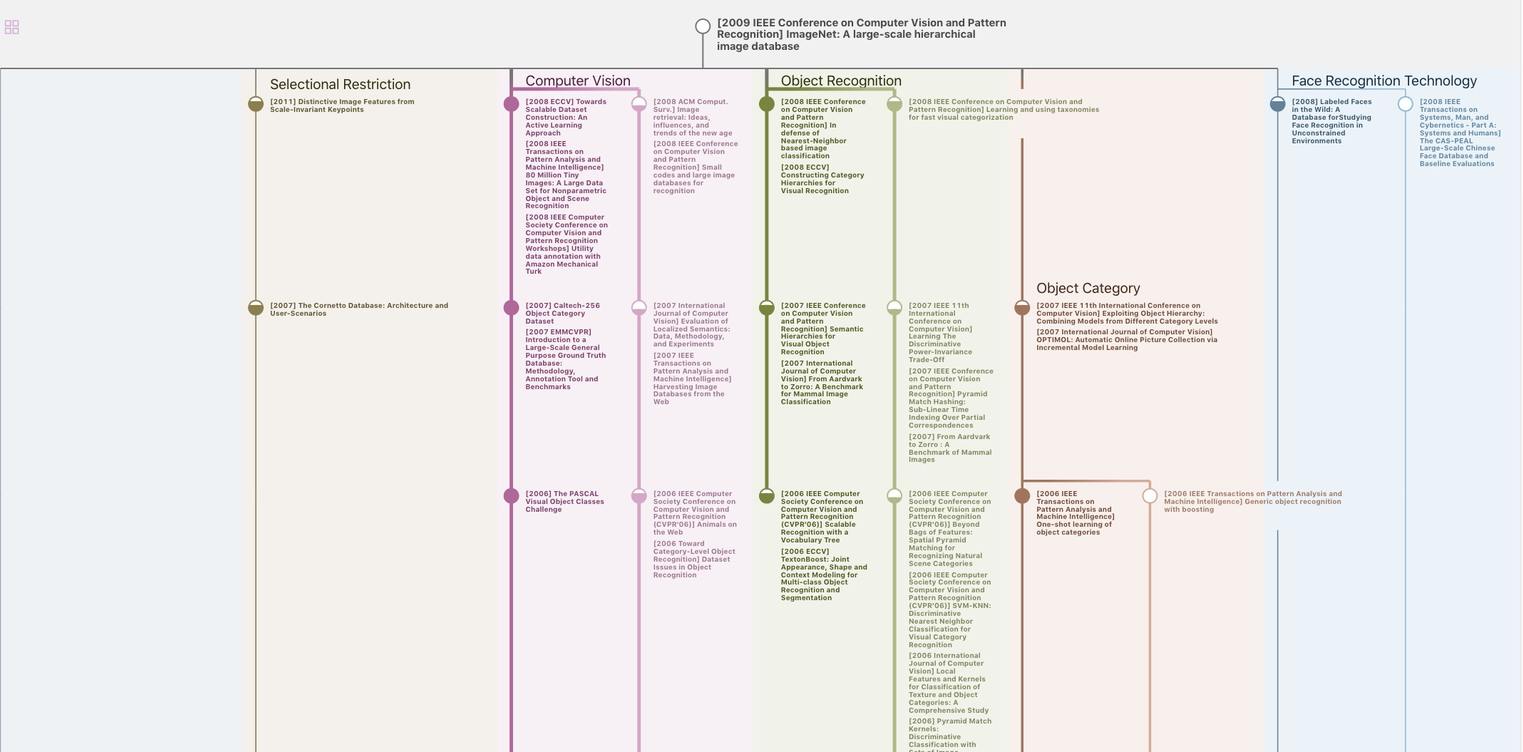
生成溯源树,研究论文发展脉络
Chat Paper
正在生成论文摘要