Extracting Grain Orientations from EBSD Patterns of Polycrystalline Materials Using Convolutional Neural Networks.
MICROSCOPY AND MICROANALYSIS(2018)
摘要
We present a deep learning approach to the indexing of electron backscatter diffraction (EBSD) patterns. We design and implement a deep convolutional neural network architecture to predict crystal orientation from the EBSD patterns. We design a differentiable approximation to the disorientation function between the predicted crystal orientation and the ground truth; the deep learning model optimizes for the mean disorientation error between the predicted crystal orientation and the ground truth using stochastic gradient descent. The deep learning model is trained using 374,852 EBSD patterns of polycrystalline nickel from simulation and evaluated using 1,000 experimental EBSD patterns of polycrystalline nickel. The deep learning model results in a mean disorientation error of 0.548 degrees compared to 0.652 degrees using dictionary based indexing.
更多查看译文
关键词
electron backscatter diffraction,EBSD,deep learning,convolutional neural networks
AI 理解论文
溯源树
样例
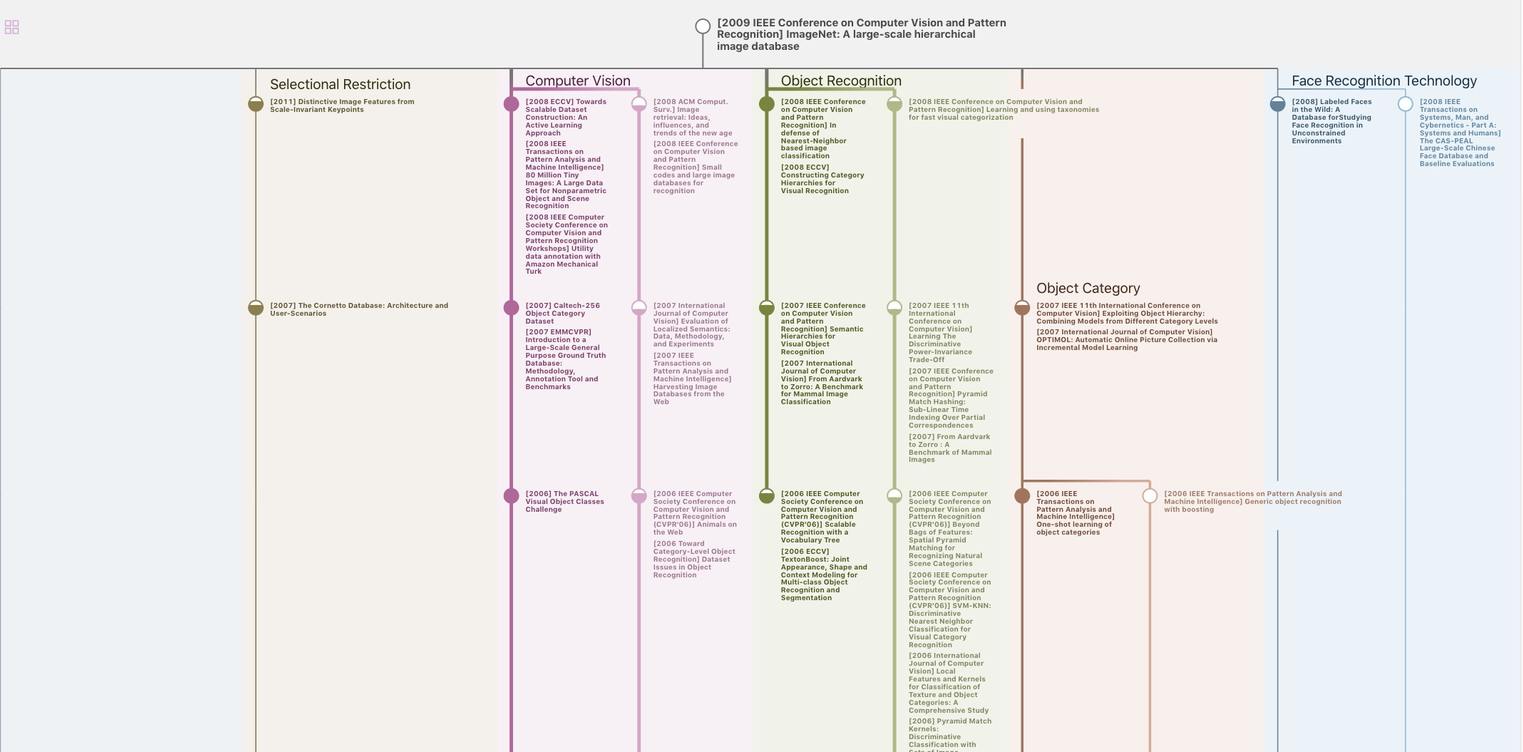
生成溯源树,研究论文发展脉络
Chat Paper
正在生成论文摘要