Maximum Likelihood Estimation-Based Joint Sparse Representation for the Classification of Hyperspectral Remote Sensing Images.
IEEE Transactions on Neural Networks and Learning Systems(2019)
摘要
A joint sparse representation (JSR) method has shown superior performance for the classification of hyperspectral images (HSIs). However, it is prone to be affected by outliers in the HSI spatial neighborhood. In order to improve the robustness of JSR, we propose a maximum likelihood estimation (MLE)-based JSR (MLEJSR) model, which replaces the traditional quadratic loss function with an MLE-like ...
更多查看译文
关键词
Nonhomogeneous media,Maximum likelihood estimation,Testing,Linear programming,Hyperspectral imaging,Training
AI 理解论文
溯源树
样例
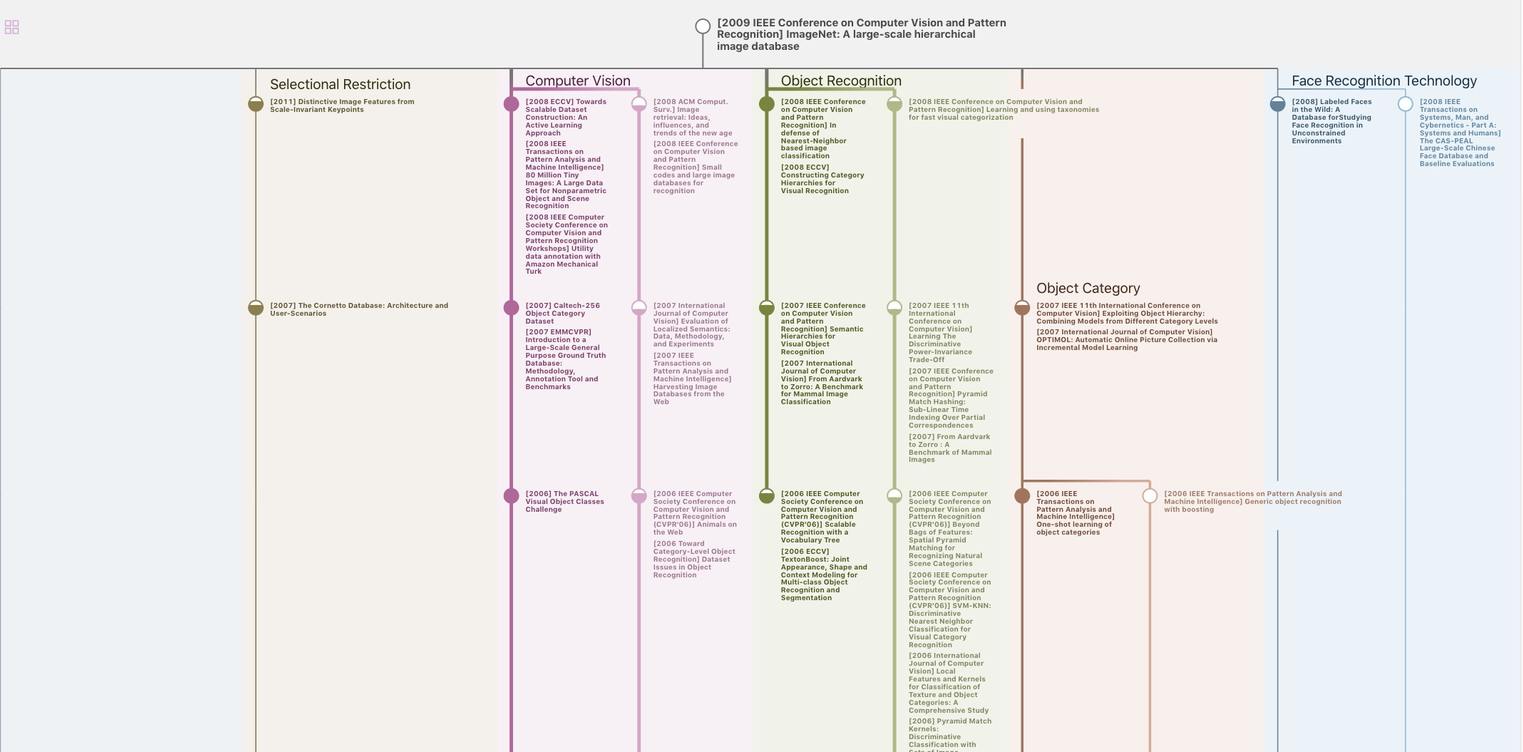
生成溯源树,研究论文发展脉络
Chat Paper
正在生成论文摘要