Numerical Quadrature for Probabilistic Policy Search.
IEEE transactions on pattern analysis and machine intelligence(2020)
摘要
Learning control policies has become an appealing alternative to the derivation of control laws based on classic control theory. Model-based approaches have proven an outstanding data efficiency, especially when combined with probabilistic models to eliminate model bias. However, a major difficulty for these methods is that multi-step-ahead predictions typically become intractable for larger planning horizons and can only poorly be approximated. In this paper, we propose the use of numerical quadrature to overcome this drawback and provide significantly more accurate multi-step-ahead predictions. As a result, our approach increases data efficiency and enhances the quality of learned policies. Furthermore, policy learning is not restricted to optimizing locally around one trajectory, as numerical quadrature provides a principled approach to extend optimization to all trajectories starting in a specified starting state region. Thus, manual effort, such as choosing informative starting points for simultaneous policy optimization, is significantly decreased. Furthermore, learning is highly robust to the choice of initial policy and, thus, interaction time with the system is minimized. Empirical evaluations on simulated benchmark problems show the efficiency of the proposed approach and support our theoretical results.
更多查看译文
关键词
Gaussian processes,System dynamics,Data models,Numerical models,Computational modeling,Predictive models,Uncertainty
AI 理解论文
溯源树
样例
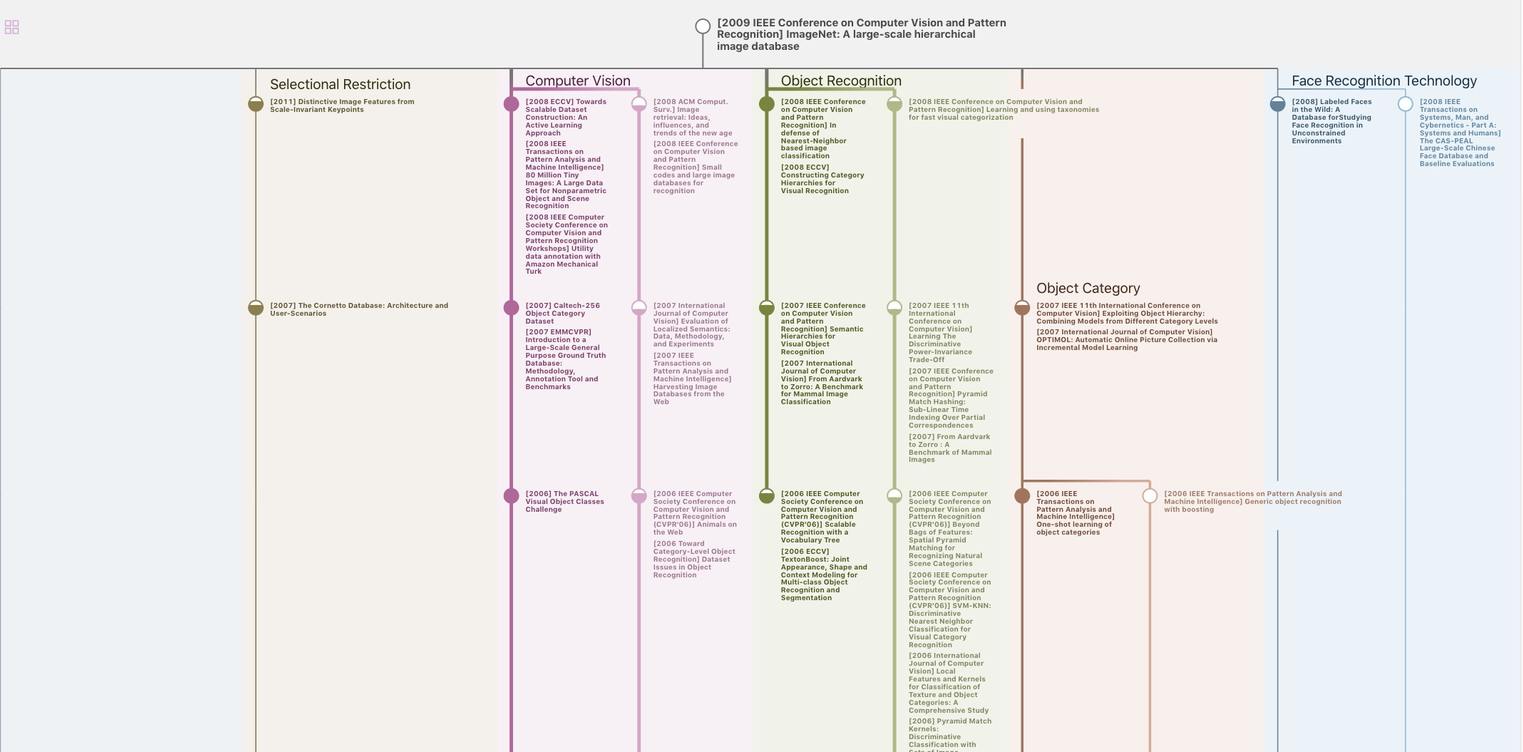
生成溯源树,研究论文发展脉络
Chat Paper
正在生成论文摘要