Remote Optimization Of An Ultracold Atoms Experiment By Experts And Citizen Scientists
PROCEEDINGS OF THE NATIONAL ACADEMY OF SCIENCES OF THE UNITED STATES OF AMERICA(2018)
摘要
We introduce a remote interface to control and optimize the experimental production of Bose-Einstein condensates (BECs) and find improved solutions using two distinct implementations. First, a team of theoreticians used a remote version of their dressed chopped random basis optimization algorithm (RedCRAB), and second, a gamified interface allowed 600 citizen scientists from around the world to participate in real-time optimization. Quantitative studies of player search behavior demonstrated that they collectively engage in a combination of local and global searches. This form of multiagent adaptive search prevents premature convergence by the explorative behavior of low-performing players while high-performing players locally refine their solutions. In addition, many successful citizen science games have relied on a problem representation that directly engaged the visual or experiential intuition of the players. Here we demonstrate that citizen scientists can also be successful in an entirely abstract problem visualization. This is encouraging because a much wider range of challenges could potentially be opened to gamification in the future.
更多查看译文
关键词
citizen science, optimal control, ultracold atoms, human problem solving, closed-loop optimization
AI 理解论文
溯源树
样例
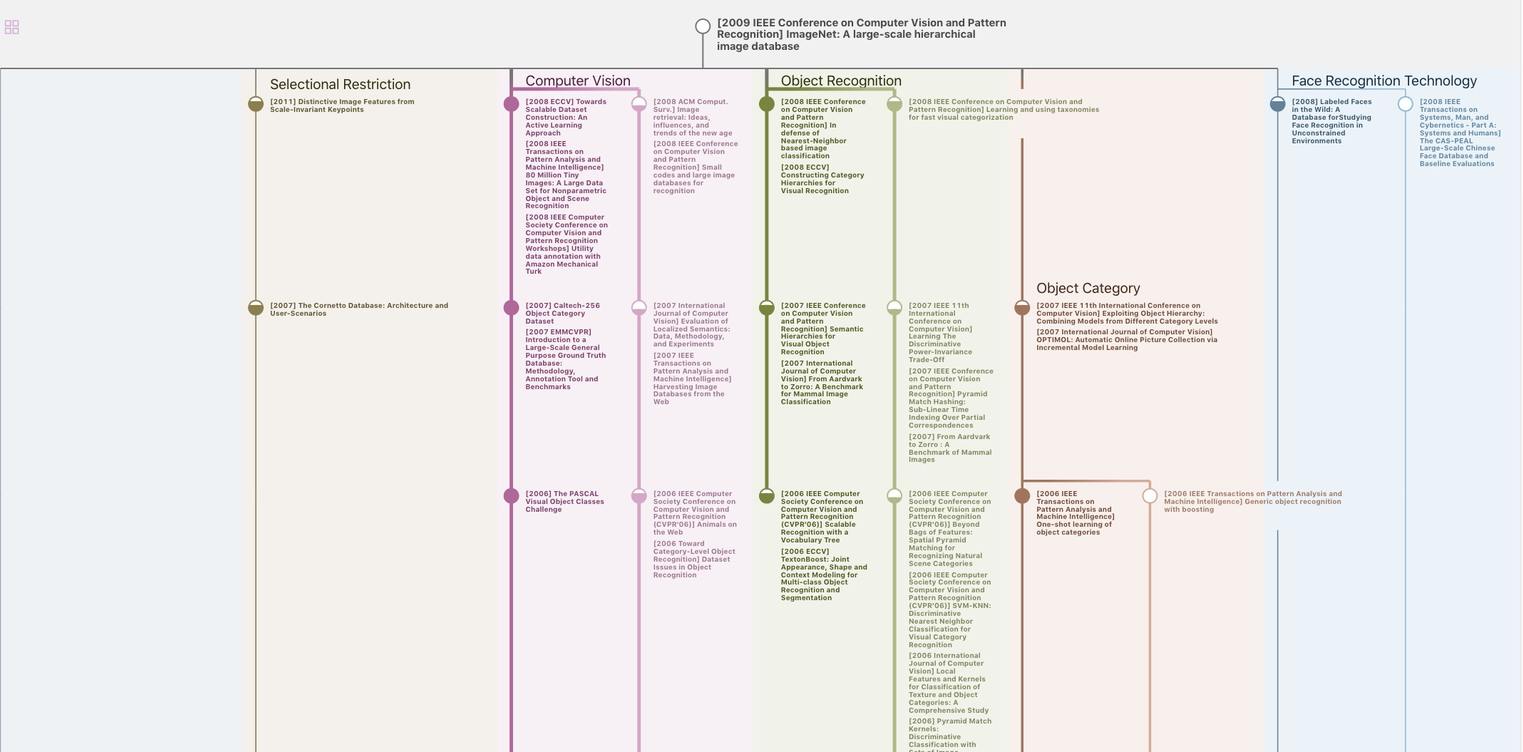
生成溯源树,研究论文发展脉络
Chat Paper
正在生成论文摘要