Comparison of Quantitative Exposure Models for Occupational Exposure to Organic Solvents in Korea.
ANNALS OF WORK EXPOSURES AND HEALTH(2019)
摘要
This study aims to evaluate the accuracy, precision and conservatism of three models (ECETOC TRA 3.1, Stoffenmanager 7.0 and ART 1.5) by comparing model predictions and repeated exposure measurements in Korea. We collected the exposure measurements (n = 390) and detailed contextual information extracted from 10 survey reports published by Ministry of Employment and Labour in the mid-2000s. Using these three models, seven occupational health professionals predicted inhalation exposure to 10 solvents used for cleaning tasks in 51 situations at 33 companies in 15 industries. We applied four comparison approaches previously used by several European studies and calculated the lack of agreement (bias, relative bias, precision), Pearson correlation coefficients (r), level of conservatism, and residuals between the log-transformed predicted estimates and measured exposures on both individual and situation levels. The overall bias and precision were -0.53 +/- 2.11 with ART, -1.32 +/- 5.88 with Stoffenmanager, and -1.03 +/- 8.88 with ECETOC TRA. Pearson correlation coefficients were significantly high in ART (r = 0.95) and Stoffenmanager (r = 0.82), but moderate in ECETOC TRA (r = 0.58). We found ART to be the most accurate model, and Stoffenmanager was the most balanced model in terms of good accuracy, high correlation, and medium conservatism in the model predictions. However, ECETOC TRA showed less accurate outcomes and lower level of conservatism but still had moderate correlations. We observed a systematic tendency to overestimate low exposures and underestimate higher exposures in all models, similar to previous studies. Therefore, our findings suggest that these European models can be used to predict occupational exposure to solvents in Korea.
更多查看译文
关键词
Advanced REACH Tool (ART),ECETOC TRA,exposure modelling,occupational exposure assessment,STOFFENMANAGER (R)
AI 理解论文
溯源树
样例
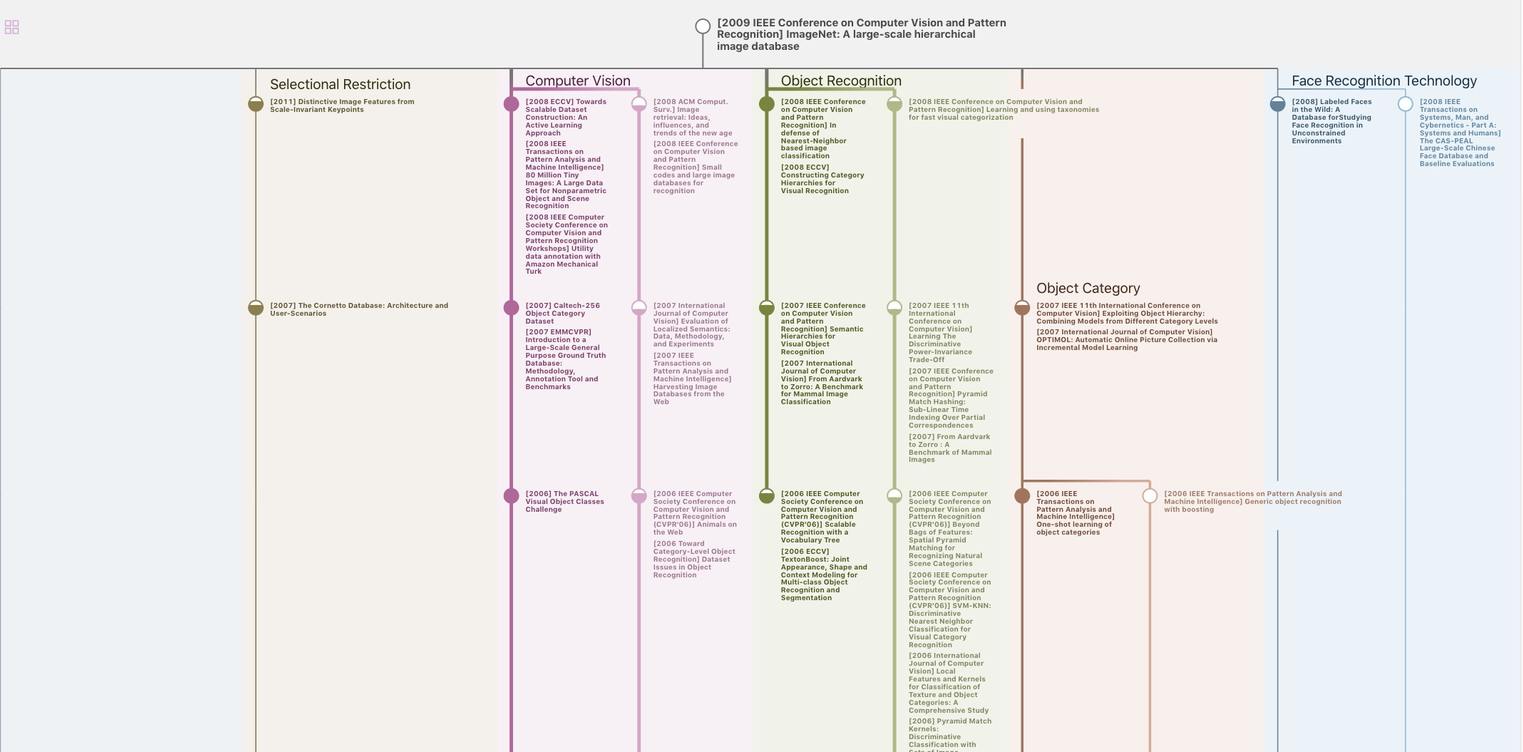
生成溯源树,研究论文发展脉络
Chat Paper
正在生成论文摘要