Detecting Breast Cancers with Mammography: Will AI Succeed Where Traditional CAD Failed?
RADIOLOGY(2019)
摘要
HomeRadiologyVol. 290, No. 2 PreviousNext Reviews and CommentaryFree AccessEditorialDetecting Breast Cancers with Mammography: Will AI Succeed Where Traditional CAD Failed?Manisha Bahl Manisha Bahl Author AffiliationsFrom the Department of Radiology, Division of Breast Imaging, Massachusetts General Hospital, 55 Fruit St, WAC 240, Boston, MA 02114.Address correspondence to the author (e-mail: [email protected]).Manisha Bahl Published Online:Nov 20 2018https://doi.org/10.1148/radiol.2018182404MoreSectionsPDF ToolsImage ViewerAdd to favoritesCiteTrack CitationsPermissionsReprints ShareShare onFacebookTwitterLinked In See also the article by Rodríguez-Ruiz et al in this issue.IntroductionScreening mammography is the most effective method for detecting early breast cancers and has been shown to reduce breast cancer–specific mortality (1). Yet, despite its efficacy, 20% of newly diagnosed cancers and almost one-third of interval cancers are evident—in retrospect—on previous mammograms (2). (Interval cancers are those diagnosed within 1 year of a mammogram reported as normal in an annual screening program or within 2 years of a mammogram reported as normal in a biennial screening program.) The most common reasons for missed breast cancers are misinterpretation of perceived abnormalities and overlooked abnormalities (3).Approved by the U.S. Food and Drug Administration in 1998, computer-aided detection (CAD) systems were developed to help radiologists detect breast cancers by reducing overlooked abnormalities. Although CAD systems can be helpful, the body of evidence in support of CAD is equivocal (4). One of the largest studies to date, which included approximately 500 000 mammograms interpreted by 271 radiologists, found that screening performance was not improved with CAD on any metric (5).Advances in artificial intelligence (AI) have led to techniques that offer promise over traditional CAD systems (6). Traditional CAD algorithms are mathematical models that can determine whether an imaging feature associated with a disease is present. For example, traditional CAD algorithms identify features such as calcifications and determine their presence or absence within a set of images. Although traditional CAD algorithms may be able to detect calcifications on mammograms, such systems cannot differentiate between benign calcifications and those seen in the setting of ductal carcinoma in situ (DCIS), a noninvasive form of breast cancer. In contrast, AI algorithms focus on a specific outcome such as DCIS and, with exposure to data, can learn to identify features or combinations of features that are associated with and predictive of DCIS.Unlike traditional CAD algorithms, the AI approach has the potential to detect useful features and relationships that are imperceptible by humans or currently unknown (6). Until recently, CAD systems relied on handcrafted features, which required consultation with radiologists during development and were thus biased toward human thought processes (7). The introduction of AI led to a shift from a rule-based, problem-specific approach to a more generic method based on learning, of which deep learning is an example. Deep learning, a subfield of AI, is based on representation learning, in which the computer is provided data and then discovers features or combinations of features that are required to classify the data (8). No handcrafted features are entered into the computer, and the computer’s developed algorithms evolve and improve with exposure to more data points. Deep learning uses data to train convolutional neural networks. These are specific types of neural networks that have features useful for image analysis (6). The many layers in deep learning algorithms are used to detect simple features (eg, lines and edges) and complex features (eg, shapes) in a hierarchical structure (8).In this issue of Radiology, Rodríguez-Ruiz and colleagues compare the breast cancer detection performance of radiologists reading screening mammography examinations unaided versus supported by a deep learning–based AI system (9). The system used by the radiologists for support was Transpara (version 1.3.0; ScreenPoint Medical, Nijmegen, the Netherlands), which is compatible with multivendor two-dimensional digital mammography and digital breast tomosynthesis examinations. The AI support provides radiologists with the following decision support tools: (a) interactive decision support (local cancer likelihood score activated by clicking on a specific breast region), (b) traditional lesion markers for computer-detected abnormalities, and (c) a proprietary examination-based cancer likelihood score. The local cancer likelihood score ranges from 1 to 100, with 100 representing the highest level of suspicion. The examination-based cancer likelihood score ranges from 1 to 10, with 10 representing the highest level of suspicion. The examination-based score is calibrated such that the number of mammograms in each category is approximately equal (10% of screening mammograms fall into category 1, 10% in category 2, etc).The retrospective, fully crossed, multireader, multicase study by Rodríguez-Ruiz and colleagues analyzes radiologists’ assessments of 240 two-dimensional digital screening mammography examinations from two different institutions (9). Fourteen radiologists from the United States interpreted the 240 examinations (enriched with 100 cancers), once with and once without AI support. When radiologists used AI support, the authors found that radiologists improved their average area under the receiver operating characteristic curve (AUC) from 0.87 to 0.89 (P = .002). Sensitivity was higher when using AI support (86% vs 83%, P = .046), and specificity also trended toward improvement (79% vs 77%, P = .06). Of note, the improvement in AUC was observed with less-experienced radiologists but not with expert radiologists. Although many of the study’s results are statistically significant, the improvements in performance with AI support are small (ie, AUC increase of 0.02 and sensitivity improvement of 3%), and whether this would actually translate to clinical practice with improved cancer detection rates is uncertain.Most striking about the study, however, was the finding that there was no difference between the stand-alone performance of the AI system and the radiologists’ average performance (AUC of 0.89 vs 0.87, respectively; P = .33) (9). There is marked variation in screening performance metrics among radiologists across the country, and abnormal interpretation rates (previously referred to as recall rates) are higher than the recommended rate for almost half of radiologists (10). The results of the study by Rodríguez-Ruiz and colleagues suggest that integration of AI systems into routine clinical practice could help radiologists with varying levels of training and experience achieve performance benchmarks and therefore improve the quality of mammography across the country. Given their results, Rodríguez-Ruiz and colleagues also suggest that AI systems could serve as stand-alone first or second readers in screening programs (9).Interpretation time is of particular importance to breast imaging radiologists as clinical workloads increase and as practices transition to digital breast tomosynthesis, which takes longer to read than two-dimensional digital mammography. Rodríguez-Ruiz and colleagues report that reading times per examination were similar with and without AI support (149 vs 146 seconds, P = .15) (9). Of note, reading times did vary based on the examination-based cancer likelihood score. Radiologists lowered their average reading time by 11% for low-suspicion examinations (scores 1–5) but increased their average reading time by 2% for high-suspicion examinations (scores 6–10). This suggests that radiologists focus less attention on low-suspicion examinations and more attention on high-suspicion examinations, as expected.A limitation of the study, as the authors point out, is the study design (9). This retrospective study included a set of mammograms enriched with screening-detected breast cancers, and awareness of the high proportion of cancers may have affected radiologists’ performance. A prospective evaluation in the clinical setting would be necessary before the AI tool is deployed for routine use. Also, radiologists need appropriate training to learn the capabilities and limitations of the tool, to take full advantage of its support while avoiding potential pitfalls. As previously mentioned, the improvements in performance with AI support were small (although statistically significant). In the future, sophisticated algorithms are likely to make AI support more powerful—particularly algorithms that incorporate temporal information (ie, comparisons to prior studies).The study by Rodríguez-Ruiz and colleagues suggests that AI algorithms are reaching a performance level that is comparable to that of radiologists with regard to cancer detection rates at screening mammography (9). Yet, before the implementation of AI tools as stand-alone first or second readers in screening programs, thorough validation with larger studies and prospective study design in actual clinical settings is needed. Whereas traditional CAD systems rely on pretaught algorithms based on handcrafted features, emerging AI systems constantly integrate and incorporate data (4). The increased recognition that mammograms contain more information than is appreciated by the human eye, coupled with the adaptive learning of neural networks, offers incredible promise for accurate and robust AI-based decision support tools for mammographic screening.Disclosures of Conflicts of Interest: disclosed no relevant relationships.References1. Independent UK Panel on Breast Cancer Screening. The benefits and harms of breast cancer screening: an independent review. Lancet 2012;380(9855):1778–1786. Crossref, Medline, Google Scholar2. Hoff SR, Samset JH, Abrahamsen AL, Vigeland E, Klepp O, Hofvind S. Missed and true interval and screen-detected breast cancers in a population based screening program. Acad Radiol 2011;18(4):454–460. Crossref, Medline, Google Scholar3. Bird RE, Wallace TW, Yankaskas BC. Analysis of cancers missed at screening mammography. Radiology 1992;184(3):613–617. Link, Google Scholar4. Katzen J, Dodelzon K. A review of computer aided detection in mammography. Clin Imaging 2018;52:305–309. Crossref, Medline, Google Scholar5. Lehman CD, Wellman RD, Buist DS, et al. Diagnostic accuracy of digital screening mammography with and without computer-aided detection. JAMA Intern Med 2015;175(11):1828–1837. Crossref, Medline, Google Scholar6. Kohli M, Prevedello LM, Filice RW, Geis JR. Implementing machine learning in radiology practice and research. AJR Am J Roentgenol 2017;208(4):754–760. Crossref, Medline, Google Scholar7. Kooi T, Litjens G, van Ginneken B, et al. Large scale deep learning for computer aided detection of mammographic lesions. Med Image Anal 2017;35:303–312. Crossref, Medline, Google Scholar8. Tang A, Tam R, Cadrin-Chênevert A, et al. Canadian Association of Radiologists white paper on artificial intelligence in radiology. Can Assoc Radiol J 2018;69(2):120–135. Crossref, Medline, Google Scholar9. Rodríguez-Ruiz A, Krupinski E, Mordang J, et al. Detection of breast cancer with mammography: impact of an artificial intelligence support system. Radiology 2019;290:305–314. Link, Google Scholar10. Lehman CD, Arao RF, Sprague BL, et al. National performance benchmarks for modern screening digital mammography: update from the Breast Cancer Surveillance Consortium. Radiology 2017;283(1):49–58. Link, Google ScholarArticle HistoryReceived: Oct 17 2018Revision requested: Oct 19 2018Revision received: Oct 22 2018Accepted: Oct 24 2018Published online: Nov 20 2018Published in print: Feb 2019 FiguresReferencesRelatedDetailsCited ByHacking and Artificial Intelligence in Radiology: Basic Principles of Data Integrity and SecurityE. RussellRitenour2023 | Contemporary Diagnostic Radiology, Vol. 46, No. 5Exploiting Patch Sizes and Resolutions for Multi-Scale Deep Learning in Mammogram Image ClassificationGonzalo IñakiQuintana, ZhijinLi, LaurenceVancamberg, MathildeMougeot, AgnèsDesolneux, SergeMuller2023 | Bioengineering, Vol. 10, No. 5Symmetry-based regularization in deep breast cancer screeningEduardoCastro, JoseCosta Pereira, Jaime S.Cardoso2023 | Medical Image Analysis, Vol. 83Lecture Notes in Networks and SystemsHoang DucQuy, Nguyen NgocSon, Ho Pham HuyAnh2023 | , Vol. 567A comparative performance study of random‐grid model for hyperparameters selection in detection of abnormalities in digital breast imagesOlaide N.Oyelade, Absalom E.Ezugwu2022 | Concurrency and Computation: Practice and Experience, Vol. 34, No. 13Each Woman’s Menopause: An Evidence Based ResourceMichelleFrankland, TrishBrown2022Mammography-based Radiomics in Breast Cancer: A Scoping Review of Current Knowledge and Future NeedsSomphoneSiviengphanom, ZibaGandomkar, Sarah J.Lewis, Patrick C.Brennan2022 | Academic Radiology, Vol. 29, No. 8Medical Imaging 2022: Computer-Aided DiagnosisYen Nhi T.Vu, BrentMombourquette, Thomas P.Matthews, JasonSu, SadanandSingh, Khan M.Iftekharuddin, KarenDrukker, Maciej A.Mazurowski, HongbingLu, ChisakoMuramatsu, Ravi K.Samala2022Artificial Intelligence (AI) for Screening Mammography, >From the AJR Special Series on AI ApplicationsLeslie R.Lamb, Constance D.Lehman, AimiliaGastounioti, Emily F.Conant, ManishaBahl2022 | American Journal of Roentgenology, Vol. 219, No. 3Leveraging Multi-Task Learning to Cope With Poor and Missing Labels of MammogramsMickaelTardy, DianaMateus2022 | Frontiers in Radiology, Vol. 1Breast cancer screening in average and high-risk womenW. TaniaRahman, Mark A.Helvie2022 | Best Practice & Research Clinical Obstetrics & Gynaecology, Vol. 83Application of deep learning in the detection of breast lesions with four different breast densitiesHongmeiLi, JingYe, HaoLiu, YichuanWang, BinbinShi, JuanChen, AipingKong, QingXu, JunhuiCai2021 | Cancer Medicine, Vol. 10, No. 14Applications of Artificial Intelligence in Breast ImagingMatthew B.Morgan, Jonathan L.Mates2021 | Radiologic Clinics of North America, Vol. 59, No. 1¿Son los sistemas de inteligencia artificial una herramienta útil para los programas de cribado de cáncer de mama?O.Díaz, A.Rodríguez-Ruiz, A.Gubern-Mérida, R.Martí, M.Chevalier2021 | Radiología, Vol. 63, No. 3Are artificial intelligence systems useful in breast cancer screening programmes?O.Díaz, A.Rodríguez-Ruiz, A.Gubern-Mérida, R.Martí, M.Chevalier2021 | Radiología (English Edition), Vol. 63, No. 3Artificial Intelligence for Breast Ultrasound: Will It Impact Radiologists’ Accuracy?ManishaBahl2021 | Journal of Breast Imaging, Vol. 3, No. 3Can artificial intelligence replace ultrasound as a complementary tool to mammogram for the diagnosis of the breast cancer?SaharMansour, RashaKamal, LamiaaHashem, BasmaAlKalaawy2021 | The British Journal of Radiology, Vol. 94, No. 1128Diagnostic Performance of Artificial Intelligence-Based Computer-Aided Diagnosis for Breast Microcalcification on MammographyYoon AhDo, MijungJang, Bo LaYun, Sung UiShin, BohyoungKim, Sun MiKim2021 | Diagnostics, Vol. 11, No. 8Artificial Intelligence: A Primer for Breast Imaging RadiologistsManishaBahl2020 | Journal of Breast Imaging, Vol. 2, No. 4A Deep Learning Model to Triage Screening Mammograms: A Simulation StudyAdam Yala, Tal Schuster, Randy Miles, Regina Barzilay, Constance Lehman, 6 August 2019 | Radiology, Vol. 293, No. 1Accompanying This ArticleDetection of Breast Cancer with Mammography: Effect of an Artificial Intelligence Support SystemNov 20 2018RadiologyRecommended Articles Artificial Intelligence for Mammography and Digital Breast Tomosynthesis: Current Concepts and Future PerspectivesRadiology2019Volume: 293Issue: 2pp. 246-259Consensus Reads: The More Sets of Eyes Interpreting a Mammogram, the Better for WomenRadiology2020Volume: 295Issue: 1pp. 42-43Detection of Breast Cancer with Mammography: Effect of an Artificial Intelligence Support SystemRadiology2018Volume: 290Issue: 2pp. 305-314The Coming of Age of Breast Tomosynthesis in ScreeningRadiology2019Volume: 291Issue: 1pp. 31-33Implementation of Synthesized Two-dimensional Mammography in a Population-based Digital Breast Tomosynthesis Screening ProgramRadiology2016Volume: 281Issue: 3pp. 730-736See More RSNA Education Exhibits Artificial Intelligence in Breast Imaging: Past, Present, and FutureDigital Posters2020New Horizons: Artificial Intelligence (AI) In Digital Breast Tomosynthesis (DBT)Digital Posters2021Artificial Intelligence In Breast Imaging: Whatâs Going On In Real World?Digital Posters2021 RSNA Case Collection DCIS within breast fibroadenomaRSNA Case Collection2020Ductal carcinoma in situRSNA Case Collection2020Ductal Carcinoma In SituRSNA Case Collection2022 Vol. 290, No. 2 Metrics Altmetric Score PDF download
更多查看译文
关键词
mammography,breast cancers,ai
AI 理解论文
溯源树
样例
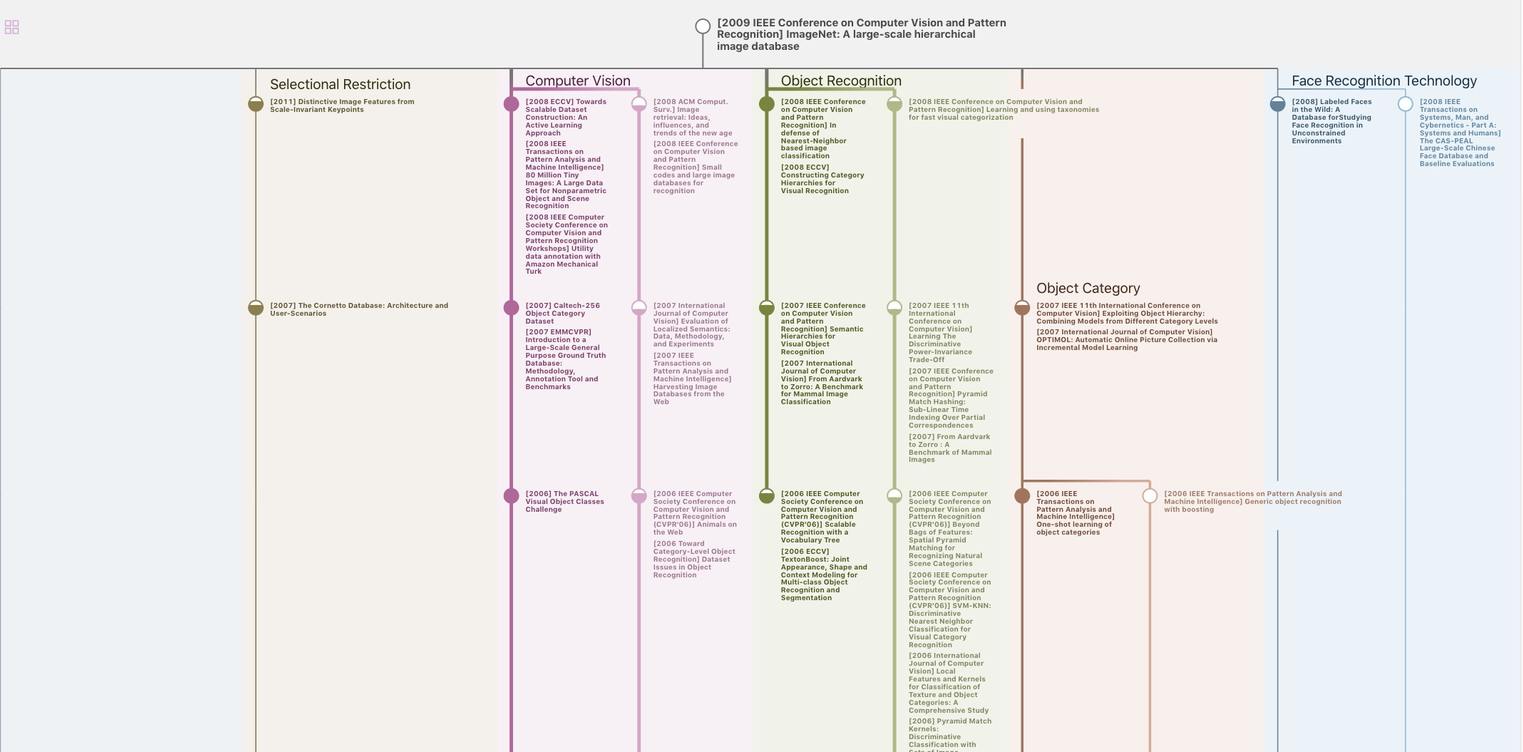
生成溯源树,研究论文发展脉络
Chat Paper
正在生成论文摘要