Predicting Invasive Disease-Free Survival for Early Stage Breast Cancer Patients Using Follow-Up Clinical Data
IEEE transactions on bio-medical engineering/IEEE transactions on biomedical engineering(2019)
摘要
Objective: Chinese women are seriously threatened by breast cancer with high morbidity and mortality. The lack of robust prognosis models results in difficulty for doctors to prepare an appropriate treatment plan that may prolong patient survival time. An alternative prognosis model framework to predict invasive disease-free survival (iDFS) for early stage breast cancer patients, called MP4Ei, is proposed. MP4Ei framework gives an excellent performance to predict the relapse or metastasis breast cancer of Chinese patients in five years. Methods: MP4Ei is built based on statistical theory and gradient boosting decision tree framework. 5246 patients, derived from the clinical research center for breast in West China Hospital of Sichuan University, with early-stage (stage I-III) breast cancer are eligible for inclusion. Stratified feature selection, including statistical and ensemble methods, is adopted to select 23 out of the 89 patient features about the patient' demographics, diagnosis, pathology, and therapy. Then, 23 selected features as the input variables are imported into the XGBoost algorithm, with Bayesian parameter tuning and cross validation, to find out the optimum simplified model for fiveyear iDFS prediction. Results: For eligible data, with 4196 patients (80%) for training, and with 1050 patients (20%) for testing, MP4Ei achieves comparable accuracy with AUC 0.8451, which has a significant advantage (p < 0.05). Conclusion: Thiswork demonstrates the complete iDFS prognosis model with very competitive performance. Significance: The proposed method in this paper could be used in clinical practice to predict patients' prognosis and future surviving state, which may help doctors make treatment plan.
更多查看译文
关键词
Breast cancer,machine learning,feature selection,prognosis prediction,relapse and metastasis cancer,gradient boosting decision tree
AI 理解论文
溯源树
样例
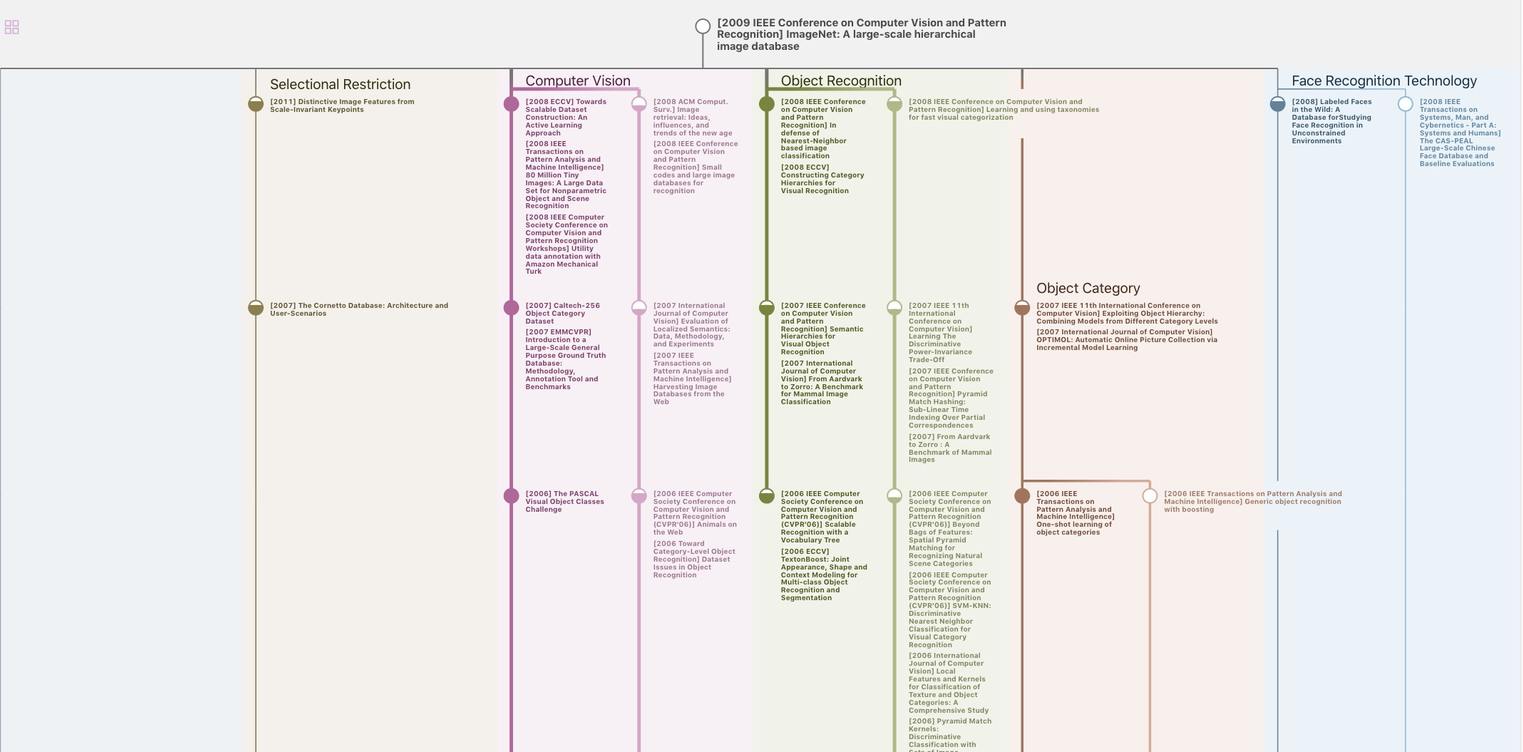
生成溯源树,研究论文发展脉络
Chat Paper
正在生成论文摘要