Hyperspectral Tissue Image Segmentation using Semi-Supervised NMF and Hierarchical Clustering.
IEEE transactions on medical imaging(2019)
摘要
Hyperspectral imaging (HSI) of tissue samples in the mid-infrared (mid-IR) range provides spectro-chemical and tissue structure information at sub-cellular spatial resolution. Disease-states can be directly assessed by analyzing the mid- IR spectra of different cell-types (e.g. epithelial cells) and sub cellular components (e.g. nuclei), provided we can accurately classify the pixels belonging to these components. The challenge is to extract information from hundreds of noisy mid-IR bands at each pixel, where each band is not very informative in itself, making annotations of unstained tissue HSI images particularly tricky. Because the tissue structure is not necessarily identical between the two sections, only a few regions in unstained HSI image can be annotated with high confidence, even when serial (or adjacent) H&E stained section is used as a visual guide. In order to completely use both labeled and unlabeled pixels in training images, we have developed an HSI pixel classification method that uses semi-supervised learning for both spectral dimension reduction and hierarchical pixel clustering. Compared to supervised classifiers, the proposed method was able to account for the vast differences in spectra of sub-cellular components of the same cell-type and achieve an F1-score of 71.18% on two fold cross-validation across 20 tissue images. To generate further interest in this promising modality we have released our source code and also showed that disease classification is straightforward after HSI image segmentation.
更多查看译文
关键词
Image segmentation,Imaging,Diseases,Spatial resolution,Chemicals,Biological tissues,Quantum cascade lasers
AI 理解论文
溯源树
样例
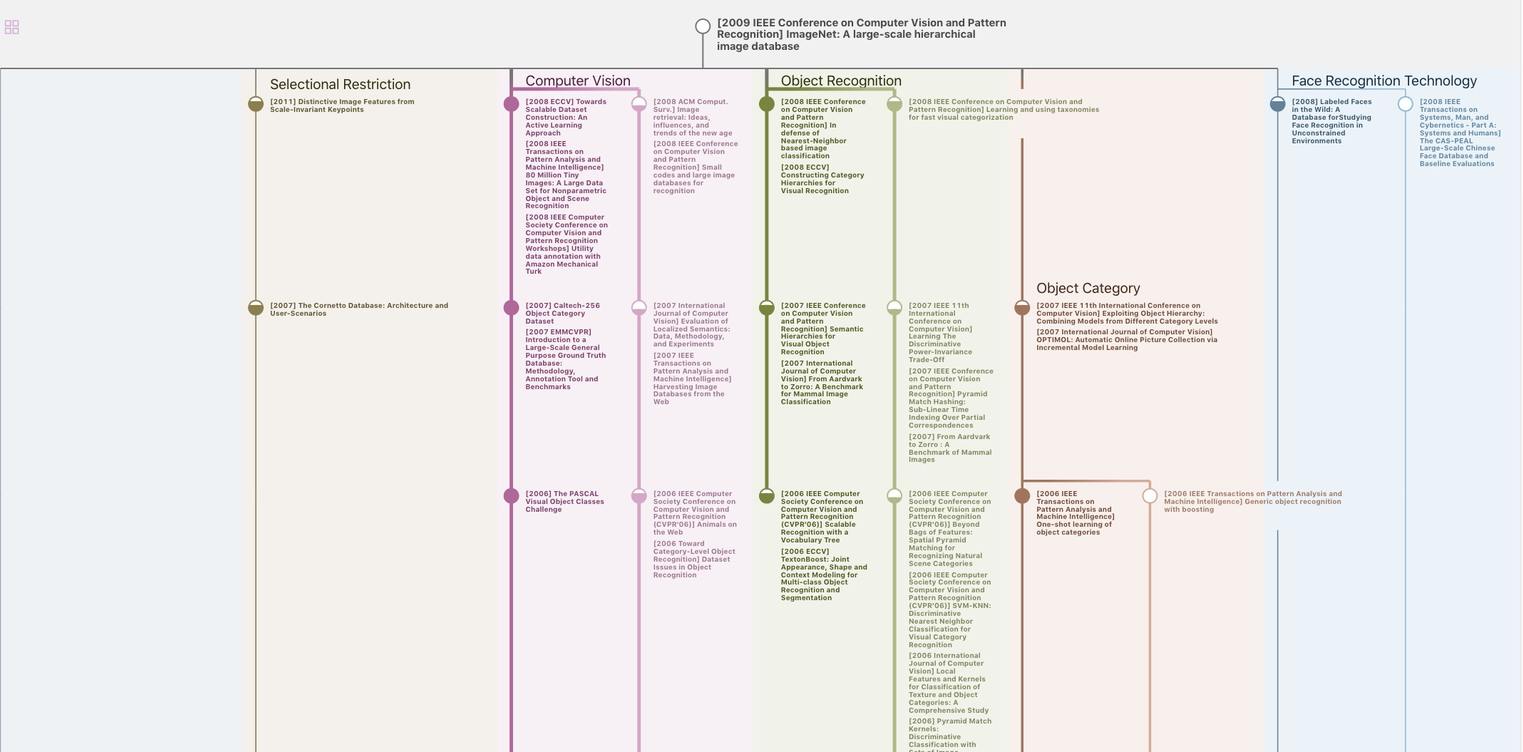
生成溯源树,研究论文发展脉络
Chat Paper
正在生成论文摘要