Deepfake Detection Via Joint Unsupervised Reconstruction and Supervised Classification.
Neurocomputing(2024)
摘要
. Deep learning has enabled realistic face manipulation for malicious purposes (i.e., deepfakes), which poses significant concerns over the integrity of the media in circulation. Most existing deep learning techniques for deepfake detection can achieve promising performance in the intra-dataset evaluation setting (i.e., training and testing on the same dataset), but are unable to perform satisfactorily in the inter-dataset evaluation setting (i.e., training on one dataset and testing on another). Most of the previous methods use the backbone network to extract global features for making predictions and only employ binary supervision (i.e., indicating whether the training instances are fake or authentic) to train the network. Classification merely based on the learning of global features often leads to weak generalizability to deepfakes attributed to unseen manipulation methods. Previous methods also use a reconstruction process to improve the learned representation. However, they did not consider the merit of combining the reconstruction task with the classification task. In this paper, we introduce a novel approach for deepfake detection, which considers the reconstruction and classification tasks simultaneously to address these problems. This method shares the information learned by one task with the other, each focusing on different aspects, and hence boosts the overall performance. In particular, we design a two-branch Convolutional AutoEncoder (CAE), in which the Convolutional Encoder used to compress the feature map into a latent representation is shared by both branches. The latent representation of the input data is then fed to a simple classifier and the unsupervised reconstruction com-ponent simultaneously. Our network is trained end-to-end. Experiments demonstrate that our method achieves state-of-the-art performance on three commonly-used datasets, particularly in the cross-dataset evaluation setting.
更多查看译文
关键词
Deepfake detection,Unsupervised reconstruction,Joint supervision
AI 理解论文
溯源树
样例
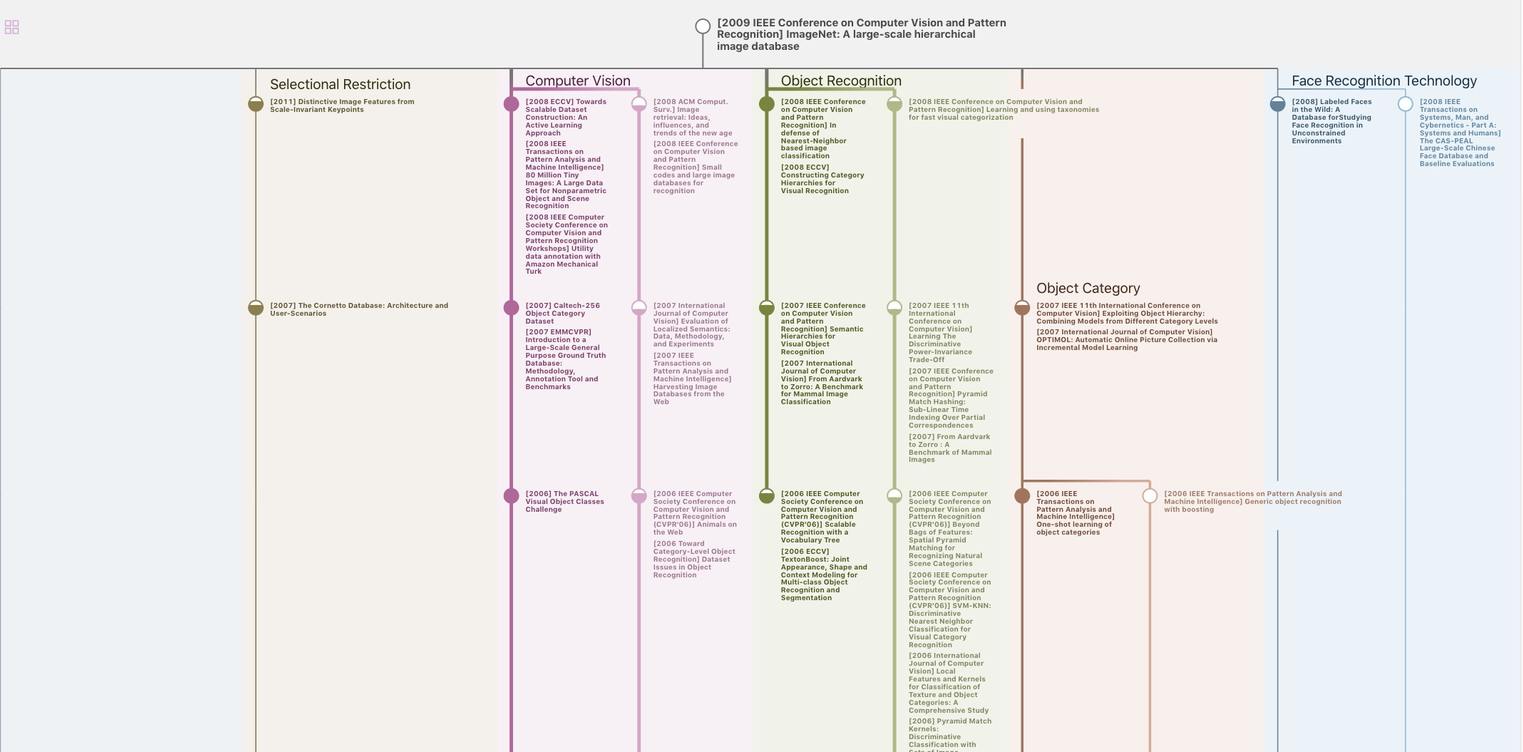
生成溯源树,研究论文发展脉络
Chat Paper
正在生成论文摘要