Compensation of Feature Selection Biases Accompanied with Improved Predictive Performance for Binary Classification by Using a Novel Ensemble Feature Selection Approach.
BioData mining(2016)
摘要
Motivation Biomarker discovery methods are essential to identify a minimal subset of features (e.g., serum markers in predictive medicine) that are relevant to develop prediction models with high accuracy. By now, there exist diverse feature selection methods, which either are embedded, combined, or independent of predictive learning algorithms. Many preceding studies showed the defectiveness of single feature selection results, which cause difficulties for professionals in a variety of fields (e.g., medical practitioners) to analyze and interpret the obtained feature subsets. Whereas each of these methods is highly biased, an ensemble feature selection has the advantage to alleviate and compensate for such biases. Concerning the reliability, validity, and reproducibility of these methods, we examined eight different feature selection methods for binary classification datasets and developed an ensemble feature selection system. Results By using an ensemble of feature selection methods, a quantification of the importance of the features could be obtained. The prediction models that have been trained on the selected features showed improved prediction performance.
更多查看译文
关键词
Machine learning,Feature selection,Ensemble learning,Biomarker discovery,Random forest
AI 理解论文
溯源树
样例
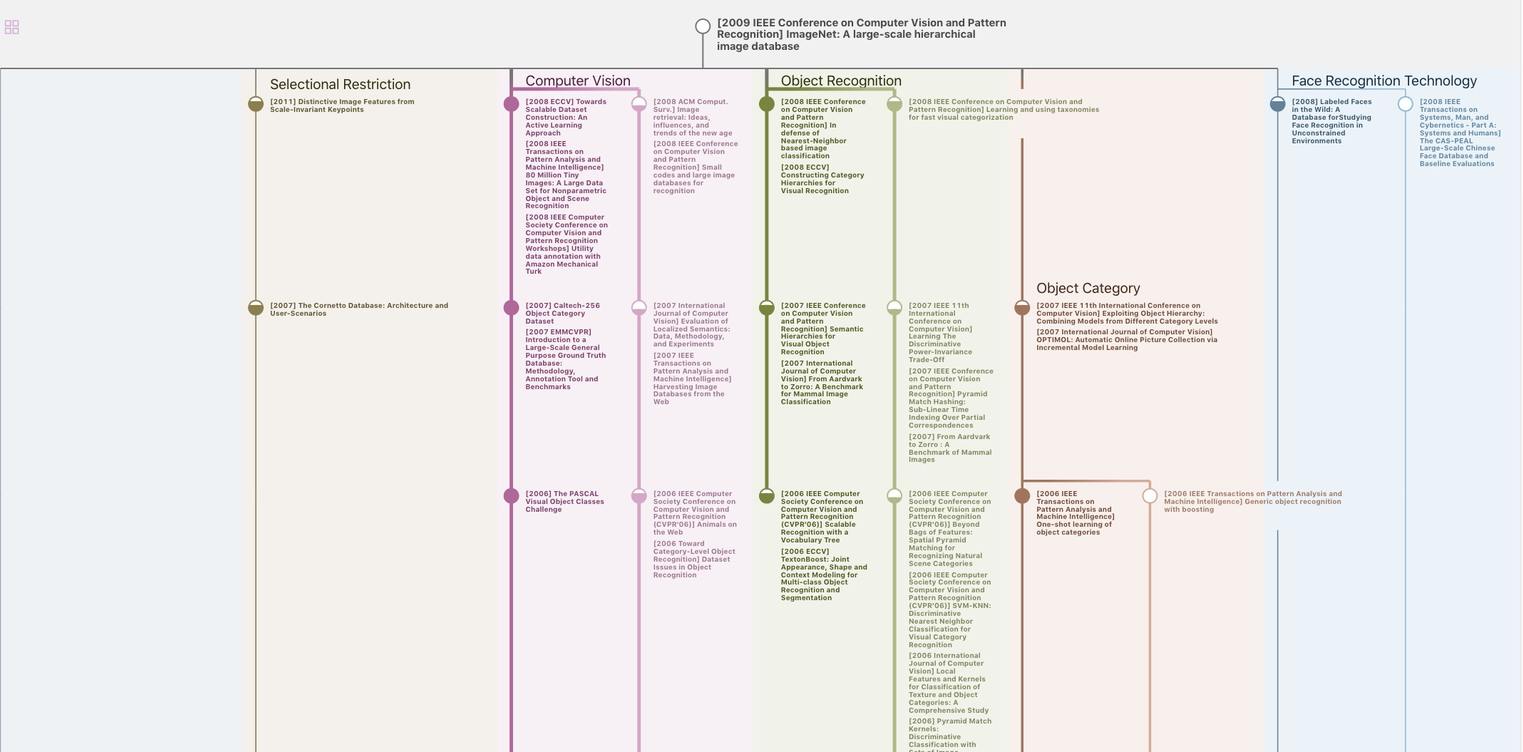
生成溯源树,研究论文发展脉络
Chat Paper
正在生成论文摘要