Gene Network Reconstruction Using Global-Local Shrinkage Priors
Annals of applied statistics/The annals of applied statistics(2017)
摘要
Reconstructing a gene network from high-throughput molecular data is an important but challenging task, as the number of parameters to estimate easily is much larger than the sample size. A conventional remedy is to regularize or penalize the model likelihood. In network models, this is often done locally in the neighbourhood of each node or gene. However, estimation of the many regularization parameters is often difficult and can result in large statistical uncertainties. In this paper we propose to combine local regularization with global shrinkage of the regularization parameters to borrow strength between genes and improve inference. We employ a simple Bayesian model with non-sparse, conjugate priors to facilitate the use of fast variational approximations to posteriors. We discuss empirical Bayes estimation of hyper-parameters of the priors, and propose a novel approach to rank-based posterior thresholding. Using extensive model- and data-based simulations, we demonstrate that the proposed inference strategy outperforms popular (sparse) methods, yields more stable edges, and is more reproducible. The proposed method, termed ShrinkNet, is then applied to Glioblastoma to investigate the interactions between genes associated with patient survival.
更多查看译文
关键词
Undirected gene network,Bayesian inference,shrinkage,variational approximation,empirical Bayes
AI 理解论文
溯源树
样例
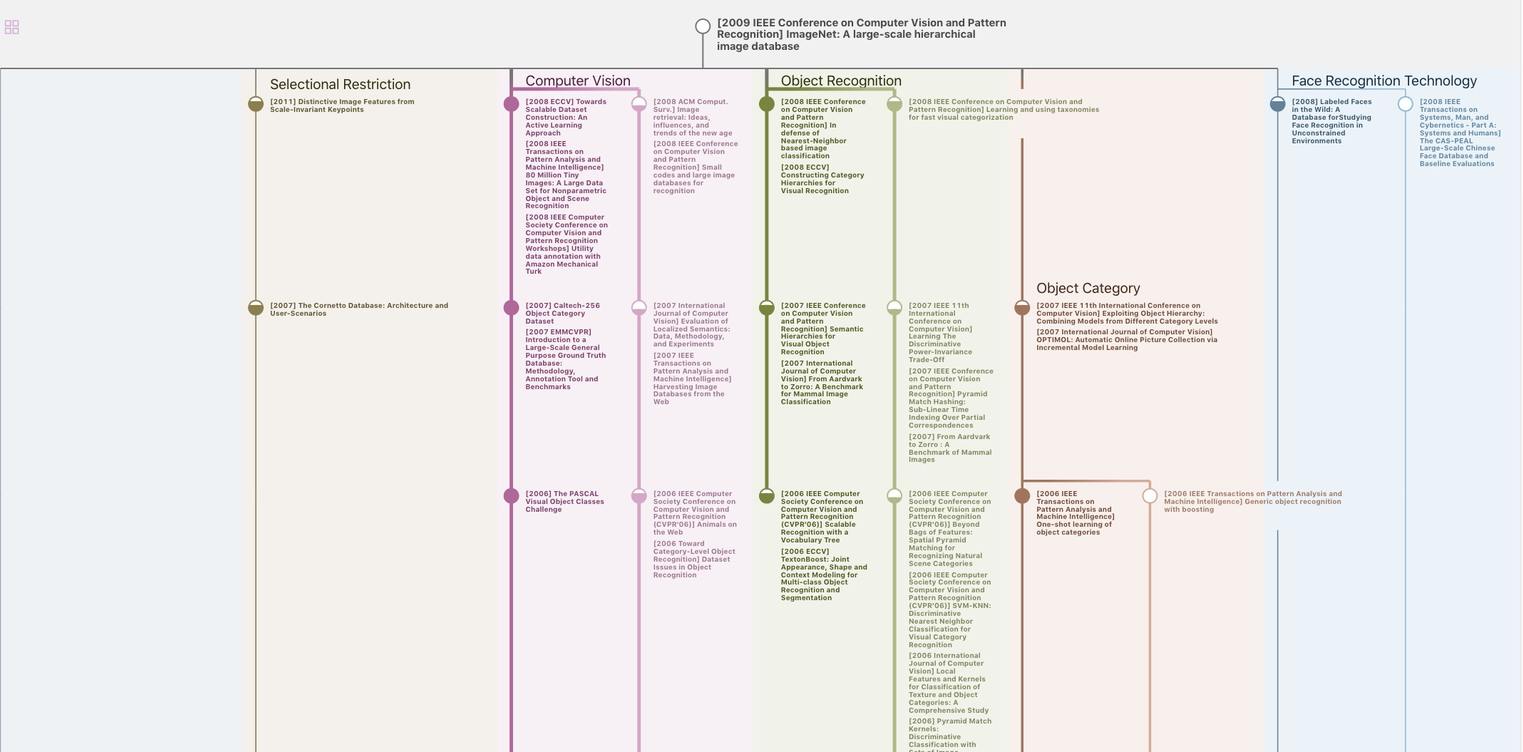
生成溯源树,研究论文发展脉络
Chat Paper
正在生成论文摘要