Urban Dynamic Logistics Pattern Mining with 3D Convolutional Neural Network
2018 IEEE International Conference on Big Data (Big Data)(2018)
摘要
With wide applications of various types of sensors and web-based software, massive location and time related logistics data is available. The massive spatial and temporal data significantly enhances visibility of modern logistics activities, however, integrating continuous time information to explore spatio-temporal pattern is an outstanding challenge. In this research, we develop a data mining method using image-based method to convert massive spatio-temporal information into heatmap based image sequence where spatial data can be embedded into image frames while image sequence represent time domain. By developing architecture of deep learning neural network with three-dimensional (3D) kernels for different convolution layers, 3D convolutional computation can be applied to images with certain temporal depth to extract dynamic spatio-temporal patterns. The method was validated with real taxi data of New York City. Experimental results demonstrated that dynamic logistics patterns could be identified with historical spatio-temporal information. Comparison of clusters generated by different learning methods showed that the proposed method could produce more accurate spatial temporal pattern.
更多查看译文
关键词
Spatio-temporal data mining,deep learning,3D convolutional neural network,dynamic pattern mining,logistics and transportation
AI 理解论文
溯源树
样例
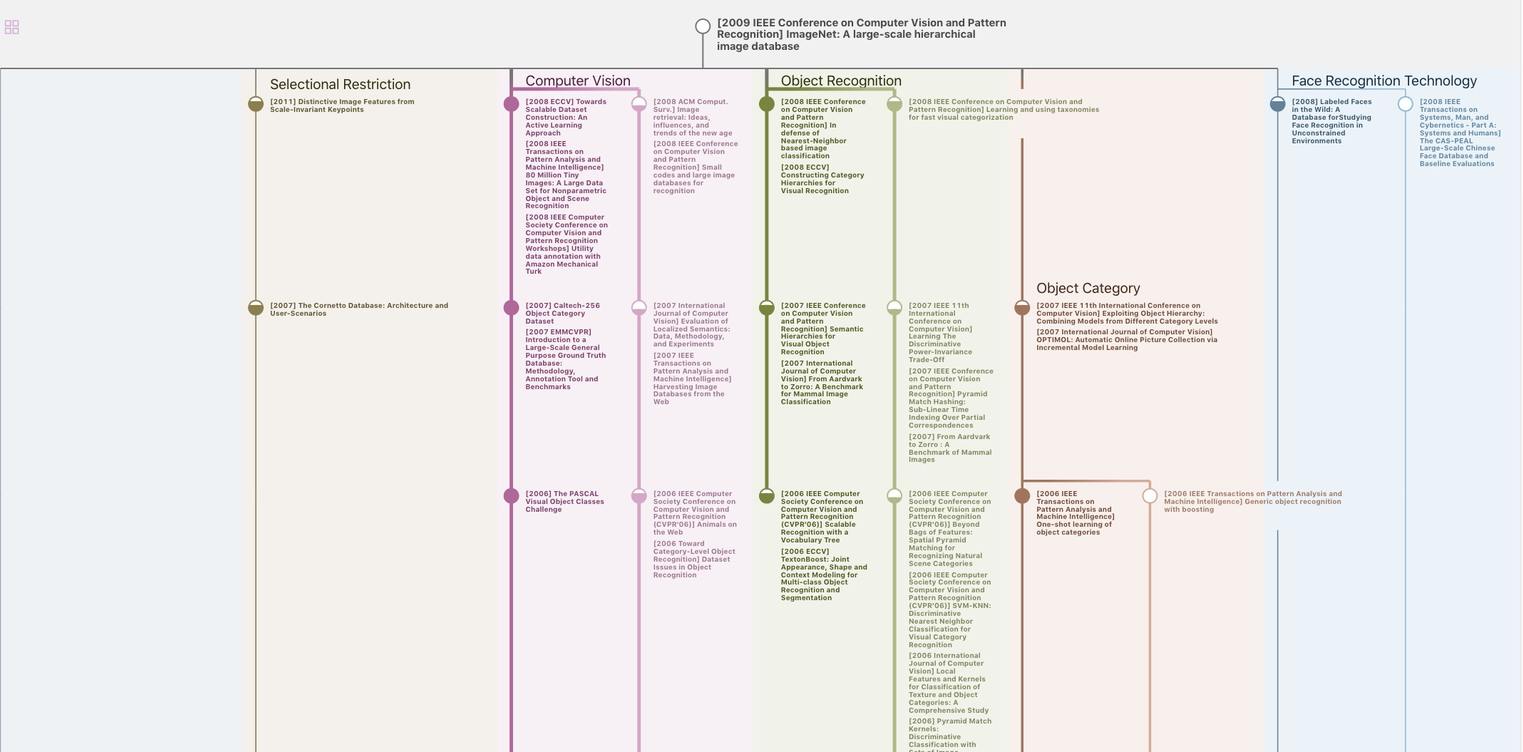
生成溯源树,研究论文发展脉络
Chat Paper
正在生成论文摘要