A Hybrid Approach to Identifying Key Factors in Environmental Health Studies
2018 IEEE International Conference on Big Data (Big Data)(2018)
摘要
In recent years, the availability of data-driven analytics has become a key tool in discovery in public health and environmental science research. As a result, these communities have looked to leverage recent advances in machine learning algorithms. This class of algorithms are able to find hidden patterns and develop new knowledge in complex data, accelerating the rate of discovery in multiple research domains. In this paper, we present our methodology of applying machine learning algorithms to health outcomes, chemical exposures, and social behavior data from expectant mothers, as part of the NIEHS-supported PROTECT Center. The ultimate goal is to determine the dominant factors/features potentially responsible for the high rate of premature births in Puerto Rico. Many commonly-used machine learning algorithms can be used for feature selection. However, given the imbalance in our birth outcome data, with many more term (i.e., 37 weeks or longer) versus preterm pregnancies (i.e., less than 37 weeks), analysis of the PROTECT dataset presents many unique challenges. In addition to outcome imbalance, our database contains both quantitative and categorical data variables, adding some complexity to the analytical methods used. Applying straightforward correlation or regression analysis would be insufficient. Our datasets also contain a significant amount of missing data (incomplete records), providing noisy input to our algorithms. A further challenge is that we are working with a relatively limited set of complex data (only 2000 participants to date), so our models must be able to be built with a relatively small number of data samples. To overcome these challenges, we have implemented a customized end-to-end analytical toolchain which forms a preprocessing pipeline. Our framework performs general data filtering and handles missing data fields using a similarity-based approach. Next, we apply one of a number of different machine learning algorithms, including Linear Correlation, Normalized Mutual Information, Logistic Regression, and Decision Trees. We use these during both feature selection and model performance evaluation. Finally, we present top-ranked features produced by our model as potential key contributors of high preterm birth rates in Puerto Rico, and discuss results across these algorithms.
更多查看译文
关键词
key factors,environmental health studies,data-driven analytics,public health,environmental science research,leverage recent advances,complex data,multiple research domains,health outcomes,social behavior data,NIEHS-supported PROTECT Center,Puerto Rico,feature selection,birth outcome data,outcome imbalance,quantitative data variables,categorical data variables,straightforward correlation,data samples,cus-tomized end-to-end analytical toolchain,framework performs general data filtering,different machine learning algorithms,potential key contributors,high preterm birth rates
AI 理解论文
溯源树
样例
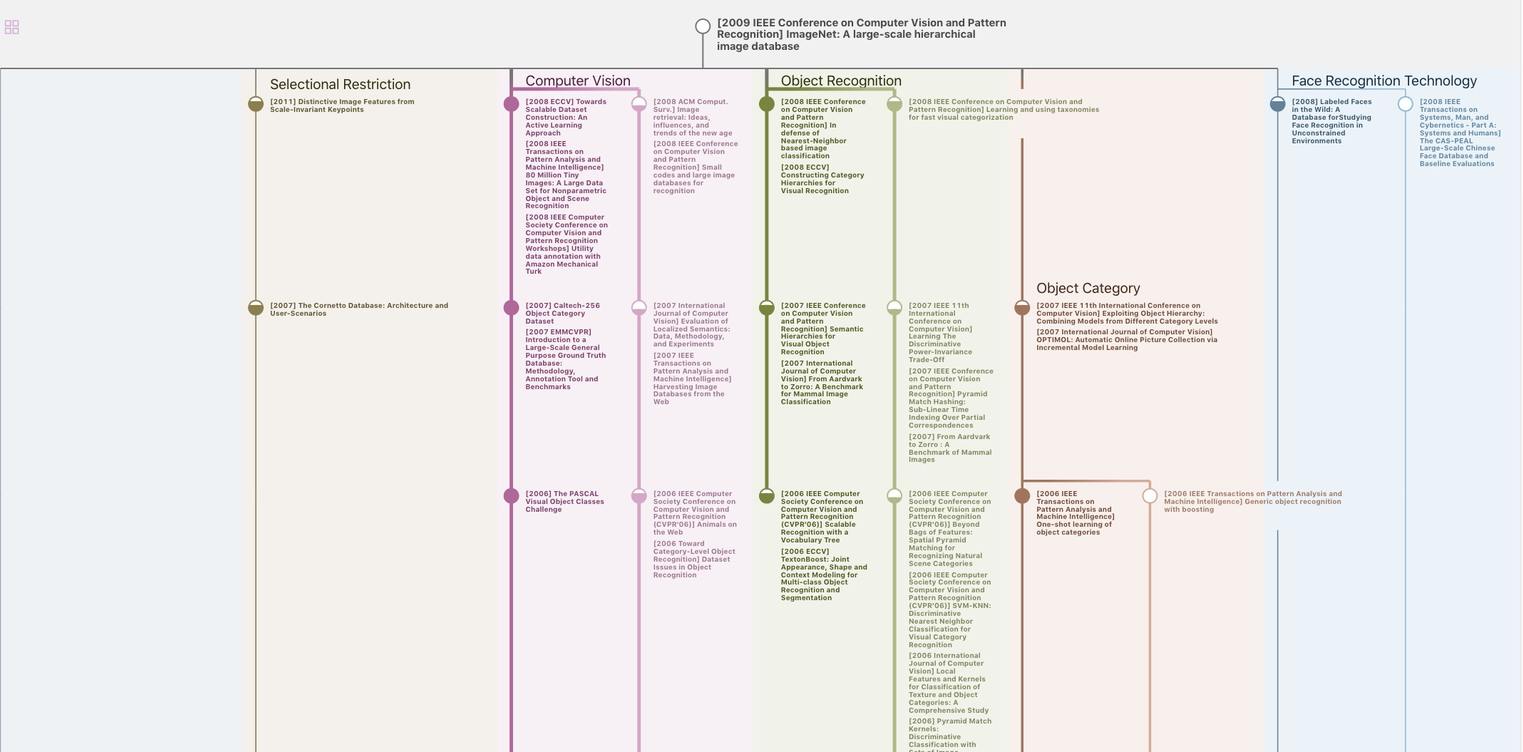
生成溯源树,研究论文发展脉络
Chat Paper
正在生成论文摘要