'Artiphysiology' reveals V4-like shape tuning in a deep network trained for image classification.
ELIFE(2018)
摘要
Deep networks provide a potentially rich interconnection between neuroscientific and artificial approaches to understanding visual intelligence, but the relationship between artificial and neural representations of complex visual form has not been elucidated at the level of single-unit selectivity. Taking the approach of an electrophysiologist to characterizing single CNN units, we found many units exhibit translation-invariant boundary curvature selectivity approaching that of exemplar neurons in the primate mid-level visual area V4. For some V4-like units, particularly in middle layers, the natural images that drove them best were qualitatively consistent with selectivity for object boundaries. Our results identify a novel image-computable model for V4 boundary curvature selectivity and suggest that such a representation may begin to emerge within an artificial network trained for image categorization, even though boundary information was not provided during training. This raises the possibility that single-unit selectivity in CNNs will become a guide for understanding sensory cortex.
更多查看译文
关键词
neuroscience,rhesus macaque
AI 理解论文
溯源树
样例
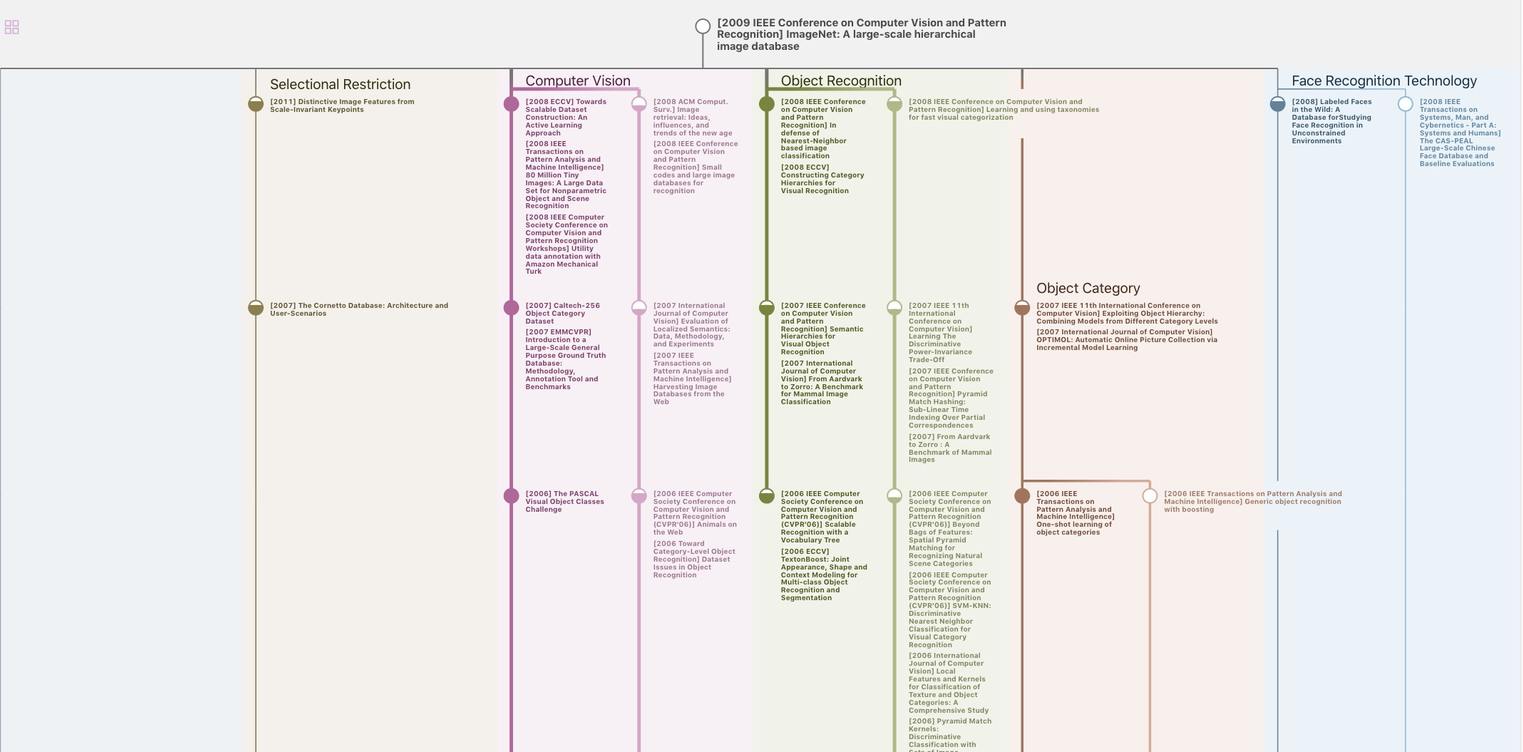
生成溯源树,研究论文发展脉络
Chat Paper
正在生成论文摘要