Sequence-based bacterial small RNAs prediction using ensemble learning strategies
BMC Bioinformatics(2018)
摘要
Background Bacterial small non-coding RNAs (sRNAs) have emerged as important elements in diverse physiological processes, including growth, development, cell proliferation, differentiation, metabolic reactions and carbon metabolism, and attract great attention. Accurate prediction of sRNAs is important and challenging, and helps to explore functions and mechanism of sRNAs. Results In this paper, we utilize a variety of sRNA sequence-derived features to develop ensemble learning methods for the sRNA prediction. First, we compile a balanced dataset and four imbalanced datasets. Then, we investigate various sRNA sequence-derived features, such as spectrum profile, mismatch profile, reverse compliment k-mer and pseudo nucleotide composition. Finally, we consider two ensemble learning strategies to integrate all features for building ensemble learning models for the sRNA prediction. One is the weighted average ensemble method (WAEM), which uses the linear weighted sum of outputs from the individual feature-based predictors to predict sRNAs. The other is the neural network ensemble method (NNEM), which trains a deep neural network by combining diverse features. In the computational experiments, we evaluate our methods on these five datasets by using 5-fold cross validation. WAEM and NNEM can produce better results than existing state-of-the-art sRNA prediction methods. Conclusions WAEM and NNEM have great potential for the sRNA prediction, and are helpful for understanding the biological mechanism of bacteria.
更多查看译文
关键词
Small RNA prediction,Sequence-derived feature,Ensemble learning,Neural network
AI 理解论文
溯源树
样例
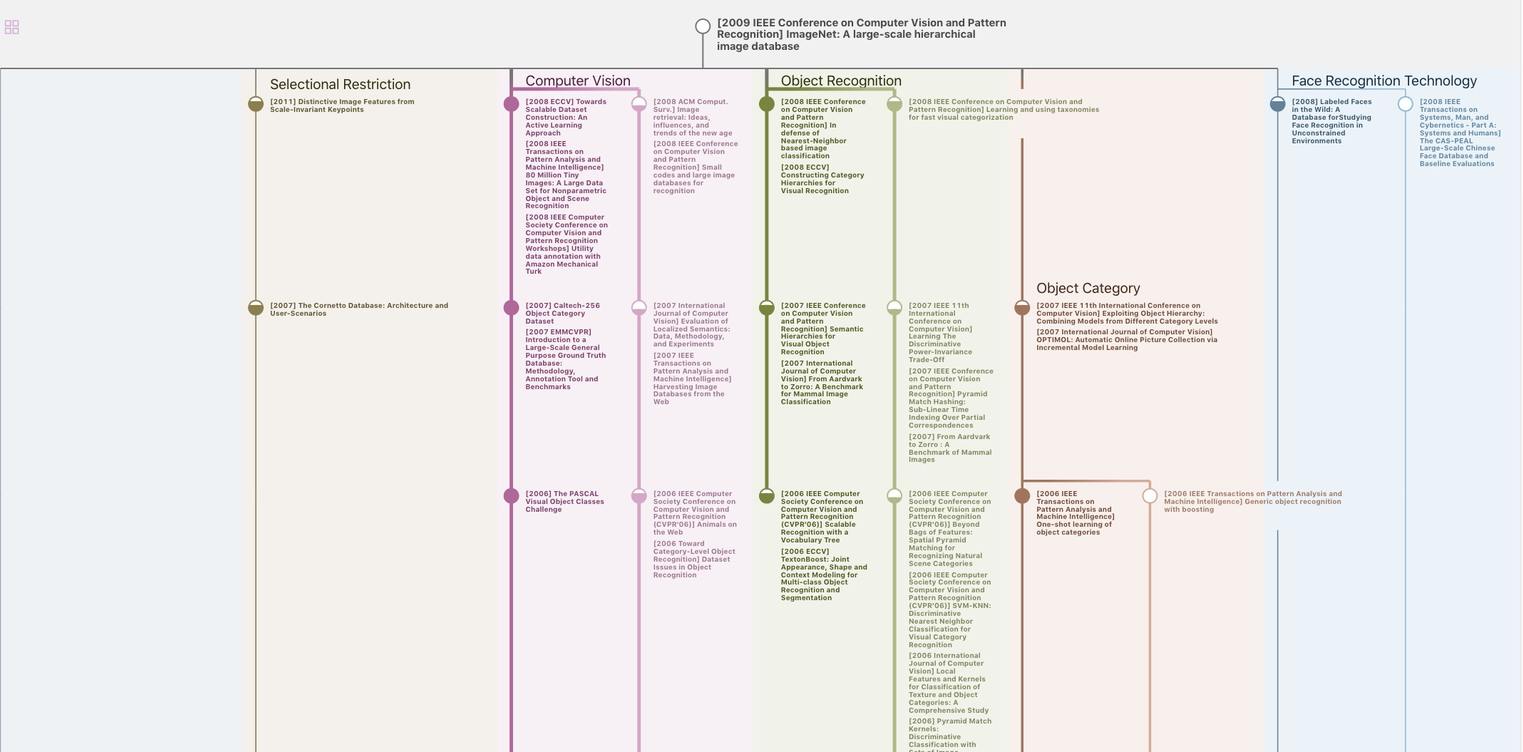
生成溯源树,研究论文发展脉络
Chat Paper
正在生成论文摘要