OFDM sensing based on deep learning
PHYSICAL COMMUNICATION(2023)
摘要
Detection of OFDM signals has received paramount interest especially for spectrum sensing in cognitive radios. We present a deep learning-based OFDM signal detection method, namely OFDM-DetNet, which combines feature learning and classifier in a single densely connected convolutional network (DenseNet) structure. OFDM-DetNet completes the detection in an end-to-end manner and utilizes the raw in-phase (I) and quadrature (Q) components of the received signal as the input and the detection result as the output. In addition, a confidence-based fusion method, namely OFDM-DetNet-F, is proposed to further improve the detection performance under low signal-to-noise ratio (SNR) scenarios without retraining the network. We construct three datasets with simulations and three datasets with real-world signals to evaluate the performance of the proposed methods. Both simulation results and experimental results indicate the superior performance of the proposed OFDM-DetNet compared with two deep learning-based OFDM detection methods, i.e., stacked autoencoder (SAE)-based method and covariance matrix (CM)-based detection method, in terms of probability of detection. Furthermore, the performance can be further improved with our proposed fusion scheme in low SNR region.
更多查看译文
关键词
Signal detection,Cognitive radio,Orthogonal frequency division multiplexing,Deep learning,Convolutional neural network
AI 理解论文
溯源树
样例
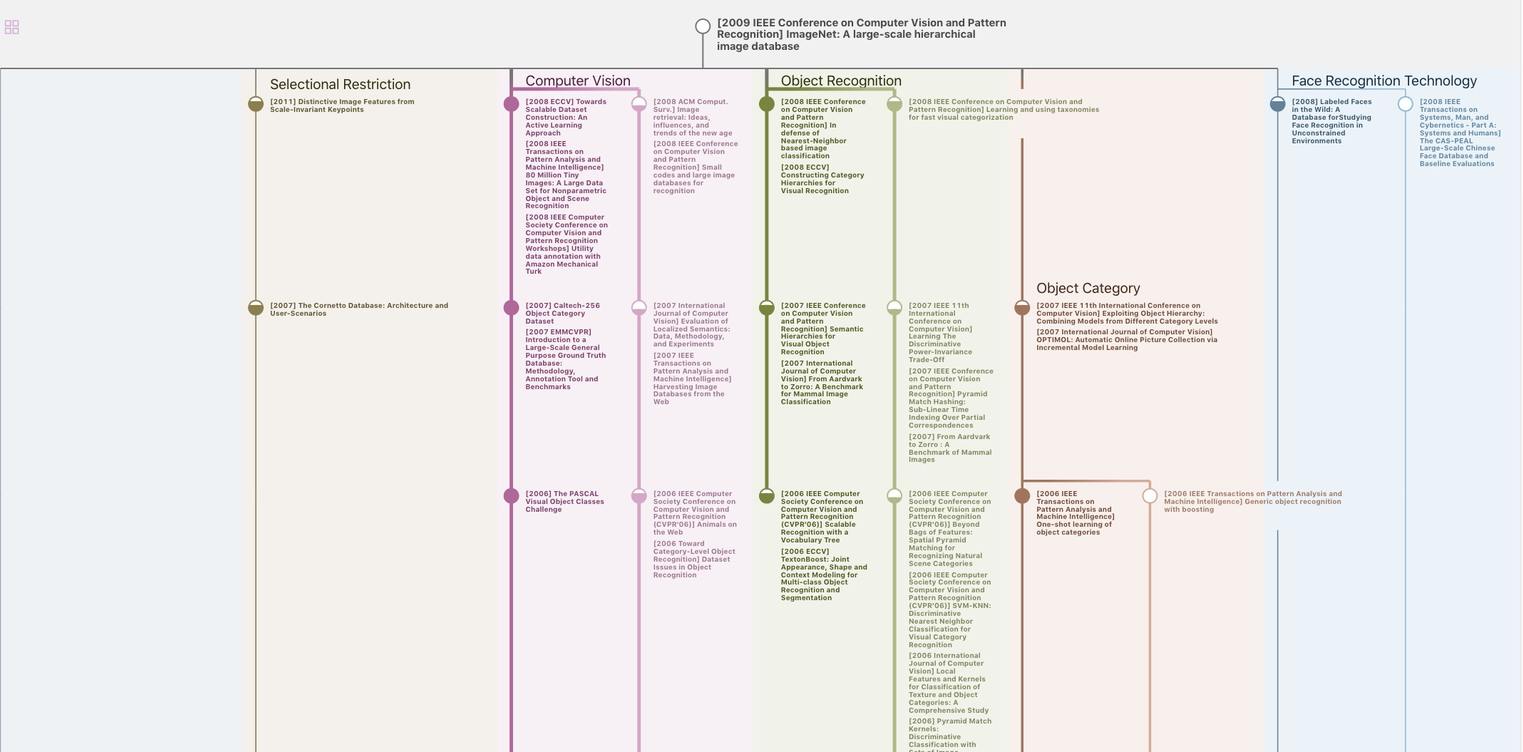
生成溯源树,研究论文发展脉络
Chat Paper
正在生成论文摘要