Empirical investigation of active learning strategies.
Neurocomputing(2019)
摘要
Many predictive tasks require labeled data to induce classification models. The data labeling process may have a high cost. Several strategies have been proposed to optimize the selection of the most relevant examples, a process referred to as active learning. However, a lack of empirical studies comparing different active learning approaches across multiple datasets makes it difficult identifying the most promising strategies, or even assessing the relative gain of active learning over the trivial random selection of instances. In this study, a comprehensive comparison of active learning strategies is presented, with various instance selection criteria, different classification algorithms and a large number of datasets. The experimental results confirm the effectiveness of active learning and provide insights about the relationship between classification algorithms and active learning strategies. Additionally, ranking curves with bands are introduced as a means to summarize in a single chart the performance of each active learning strategy for different classification algorithms and datasets.
更多查看译文
关键词
Active learning,Agnostic active learning,Non-agnostic active learning,Data sampling,Partially labeled data,Data labeling
AI 理解论文
溯源树
样例
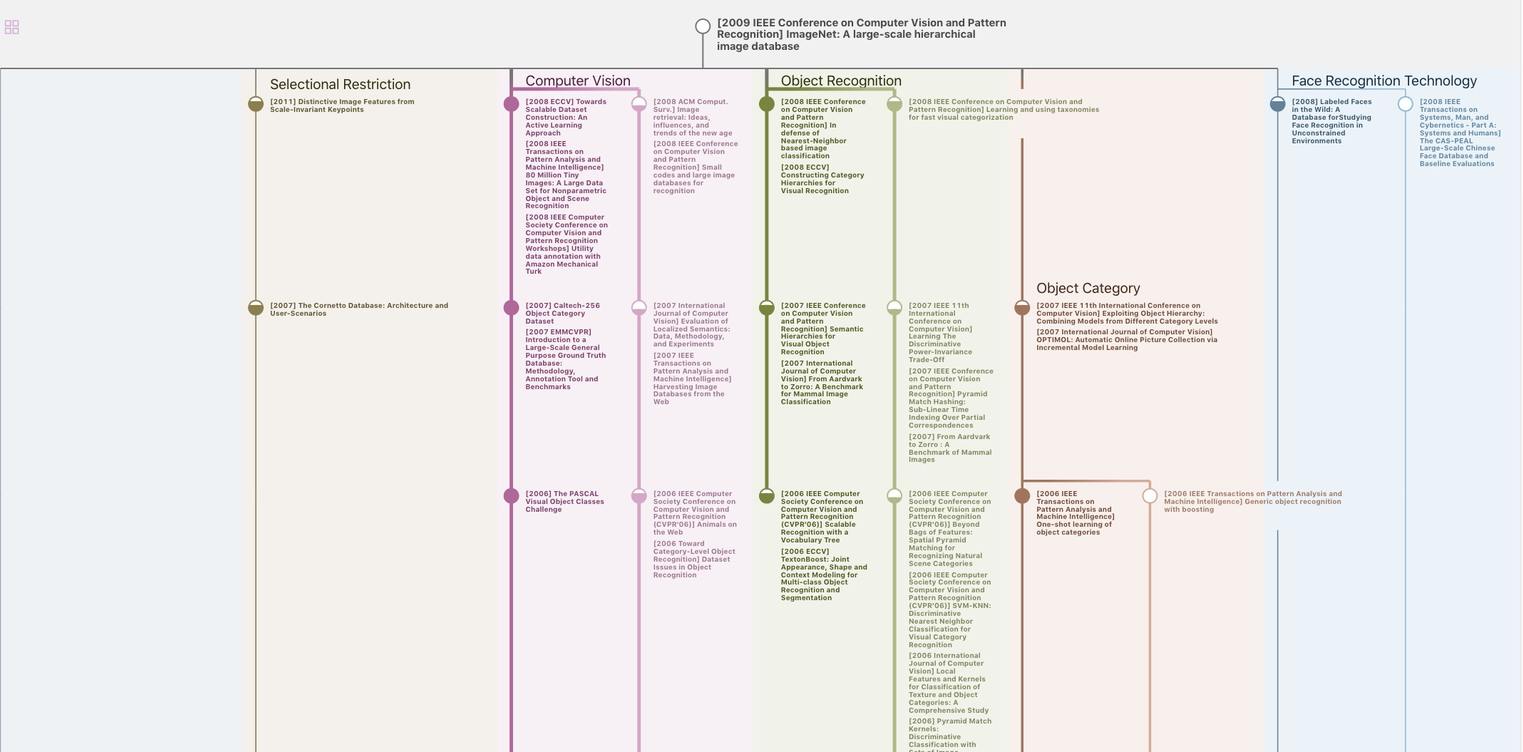
生成溯源树,研究论文发展脉络
Chat Paper
正在生成论文摘要