Search result diversification on attributed networks via nonnegative matrix factorization.
Information Processing & Management(2018)
摘要
Search result diversification is an effective way to tackle query ambiguity and enhance result novelty. In the context of large information networks, diversifying search result is also critical for further design of applications such as link prediction and citation recommendation. In previous work, this problem has mainly been tackled in a way of implicit query intent. To further enhance the performance on attributed networks, we propose a novel search result diversification approach via nonnegative matrix factorization. Our approach encodes latent query intents as well as nodes as representation vectors by a novel nonnegative matrix factorization model, and the diversity of the results accounts for the query relevance and the novelty w.r.t. these vectors. To learn the representation vectors of nodes, we derive the multiplicative updating rules to train the nonnegative matrix factorization model. We perform a comprehensive evaluation on our approach with various baselines. The results show the effectiveness of our proposed solution, and verify that attributes do help improve diversification performance.
更多查看译文
关键词
Graph search,Diversification,Nonnegative matrix factorization,Attributed network
AI 理解论文
溯源树
样例
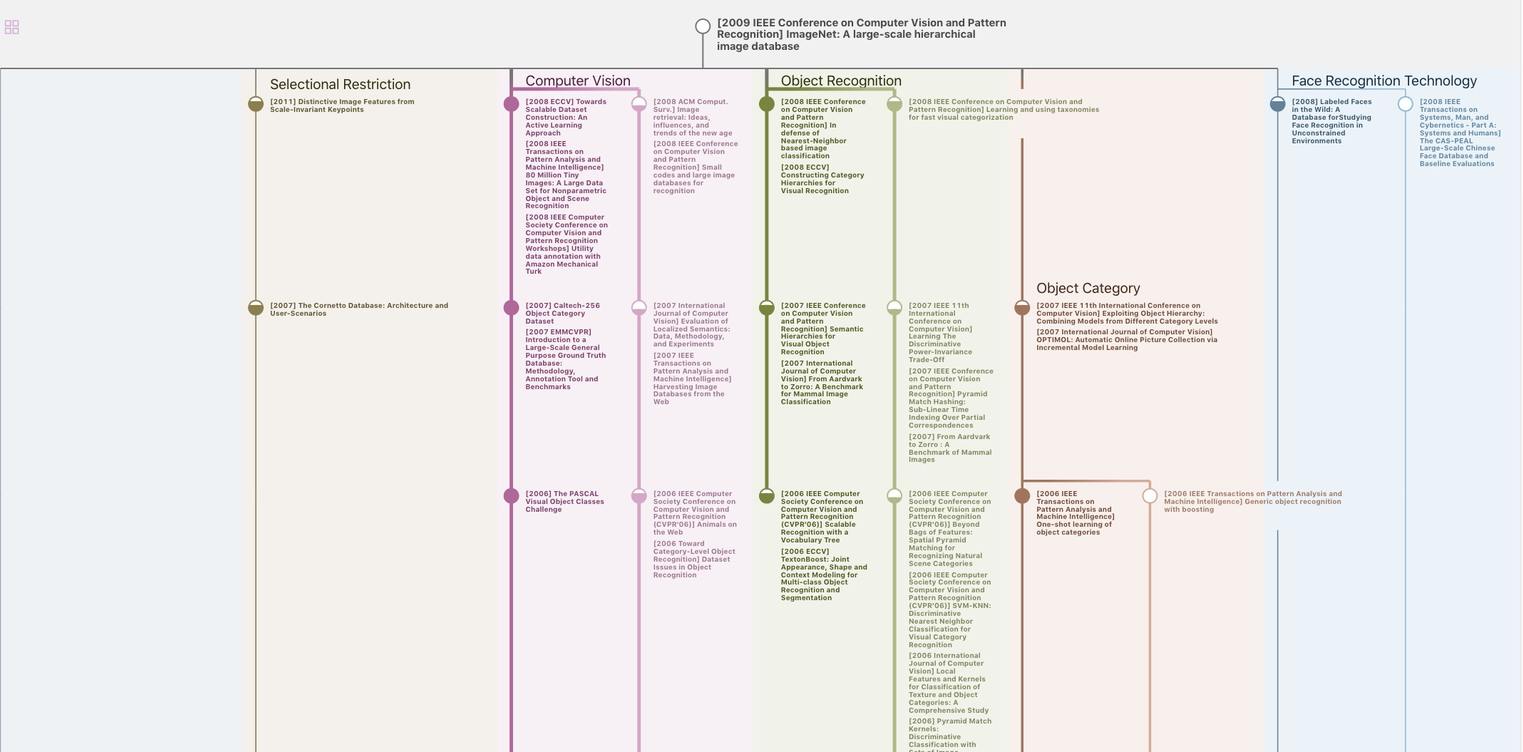
生成溯源树,研究论文发展脉络
Chat Paper
正在生成论文摘要