MLSPD - Machine Learning Based Spam and Phishing Detection
Computational Data and Social Networks Lecture Notes in Computer Science(2018)
关键词
Artificial Intelligence,Phishing,Spam emails,Supervised learning
AI 理解论文
溯源树
样例
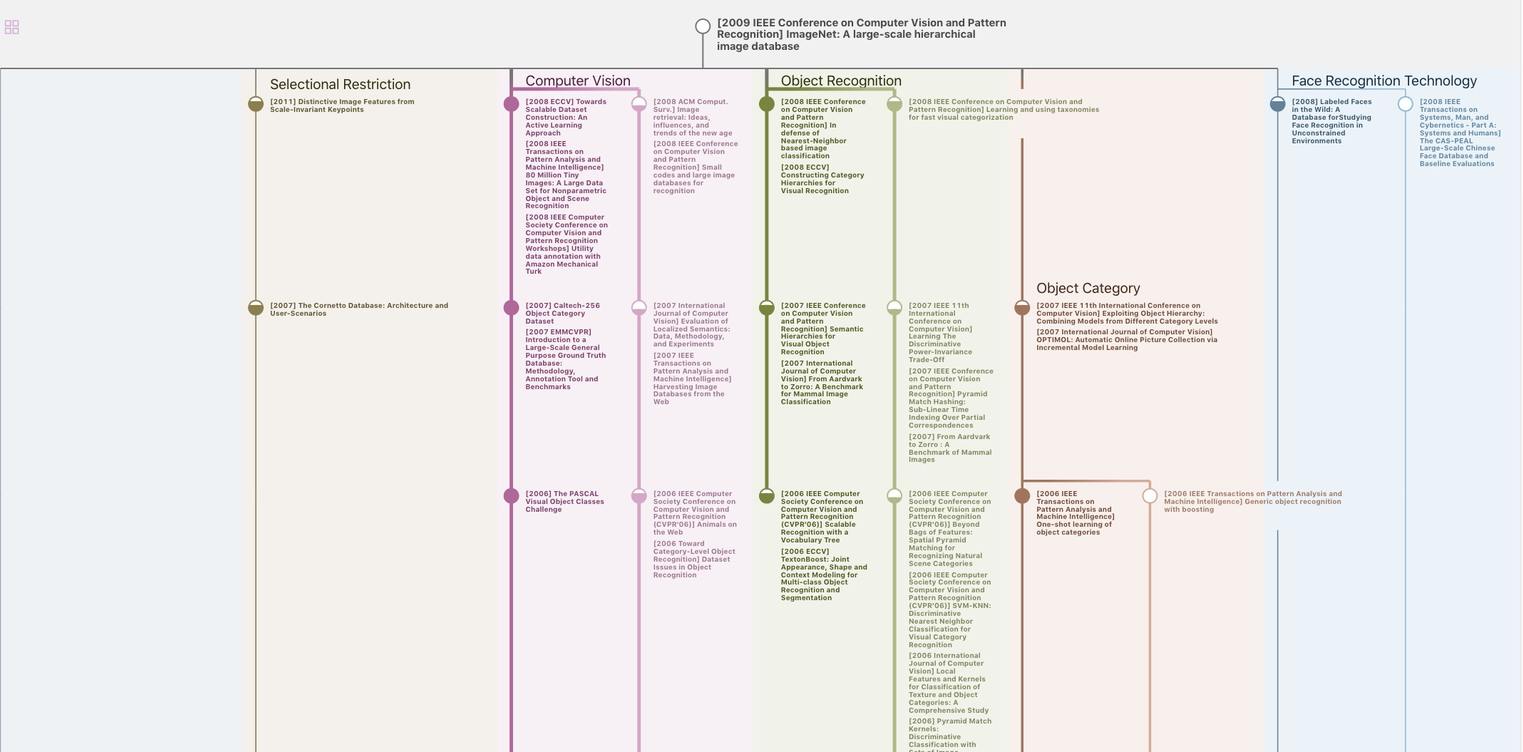
生成溯源树,研究论文发展脉络
Chat Paper
正在生成论文摘要