Investigation of Spectral Clustering for Signed Graph Matrix Representations
2018 IEEE High Performance extreme Computing Conference (HPEC)(2018)
摘要
Signed graphs [11], which allow for both favorable and adverse relationships, are becoming a common model choice for various data analysis applications, e.g., correlation clustering [1] and spectral clustering [9]. Unlike for unsigned graphs, there is no collective agreement of a matrix representation that relates to the unsigned graph Laplacian. There currently exists three proposed matrix representations: [9] proposes a zero row-sum preserving Laplacian (signed Laplacian), [8] proposes a physics preserving Laplacian (Physics Laplacian), and [4] proposes an expansion of the signed Laplacian into an unsigned Laplacian with twice the number of degrees-of-freedom (Gremban's expansion.) We investigate these three proposed matrix representations with respect to the quality of traditional (unsigned graph) spectral clustering concepts. We provide three numerical tests that use a stochastic block model with negative edge weights. We observe that the best matrix representation for spectral clustering depends on the underlying structure of a signed graph, which may be unavailable. However, since the Gremban's expansion matrix provides higher quality clusters for more combinations of inner and outer-connection probabilities for positive and negative valued edges, we conclude that the Gremban's expansion is the most robust representation to use for spectral clustering.
更多查看译文
关键词
signed graphs,Laplacian,spectral embedding,spectral clustering,Gremban's expansion
AI 理解论文
溯源树
样例
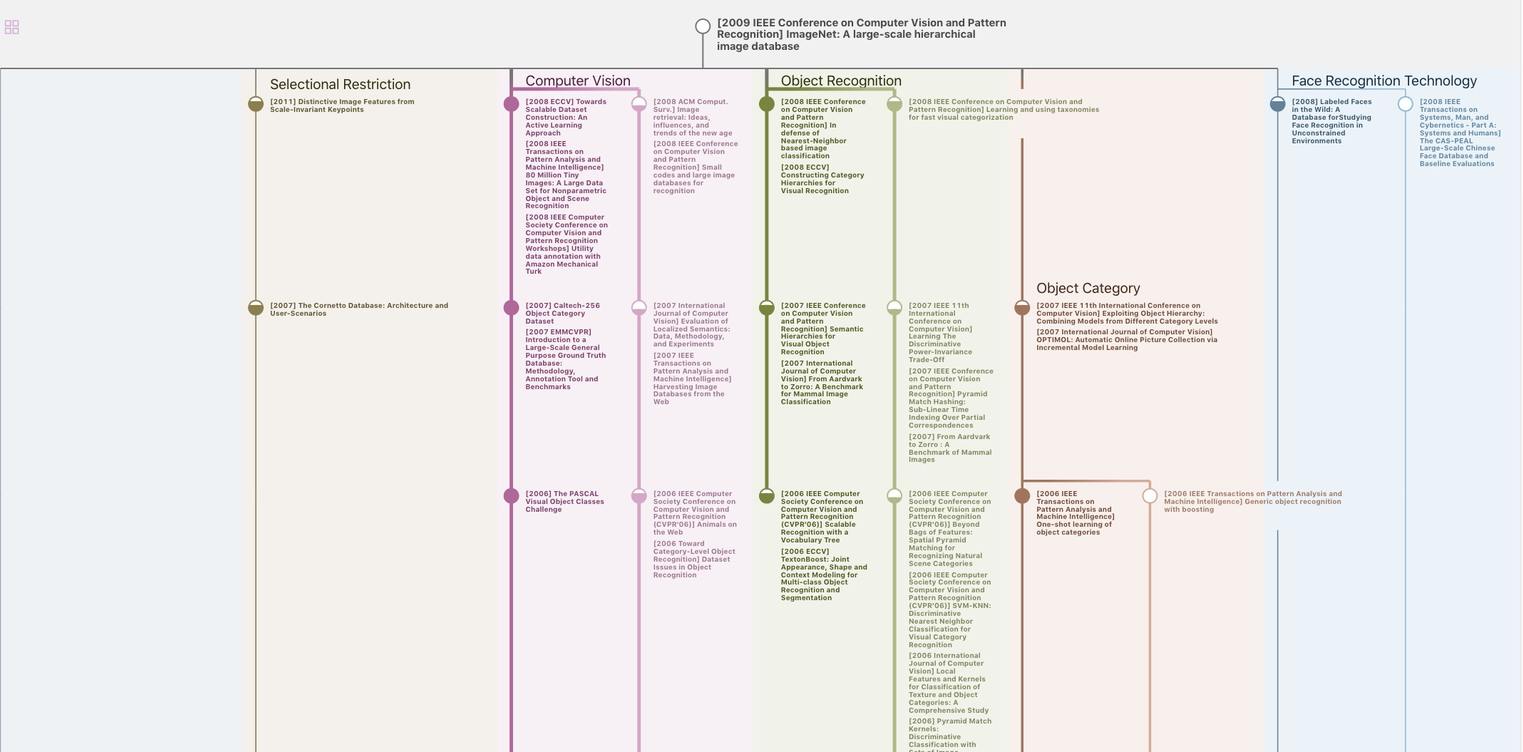
生成溯源树,研究论文发展脉络
Chat Paper
正在生成论文摘要