Automatically Detecting Arrhythmia-related Irregular Patterns using the Temporal and Spectro-Temporal Textures of ECG Signals.
ICPR(2018)
摘要
Arrhythmia is an abnormal heart rhythm that occurs due to the improper operation of the electrical impulses that coordinate the heartbeats. It is one of the most well-known heart conditions (including coronary artery disease, heart failure etc.) that is experienced by millions of people around the world. While there are several types of arrhythmias, not all of them are dangerous or harmful. However, there are arrhythmias that can often lead to death in minutes (e.g, ventricular fibrillation and ventricular tachycardia) even in young people. Thus, the detection of arrhythmia is critical for stopping and reversing its progression and for increasing longevity and life quality. While a doctor can perform different heart-monitoring tests specific to arrhythmias, the electrocardiogram (ECG) is one of the most common ones used either independently or in combination with other tests (to only detect, e.g. echocardiogram, or trigger arrhythmia and, then, detect, e.g. stress test). We propose a machine learning approach that augments the traditional arrhythmia detection approaches via our automatic arrhythmia classification system. It utilizes the texture of the ECG signal in both the temporal and spectro-temporal domains to detect and classify four types of heartbeats. The original ECG signal is first preprocessed, and then, the R-peaks associated with heartbeat estimation are identified. Next, 1D local binary patterns (LBP) in the temporal domain are utilized, while 2D LBPs and texture-based features extracted by a grayscale co-occurrence matrix (GLCM) are utilized in the spectro-temporal domain using the short-time Fourier transform (STFT) and Morse wavelets. Finally, different classifiers, as well as different ECG lead configurations are examined before we determine our proposed time-frequency SVM model, which obtains a maximum accuracy of 99.81%, sensitivity of 98.17%, and specificity of 99.98% when using a 10 cross-validation on the MIT-BIH database. Our approach yields competitive accuracy when compared to other methods discussed in the literature.
更多查看译文
关键词
traditional arrhythmia detection approaches,automatic arrhythmia classification system,spectro-temporal domain,arrhythmia-related irregular patterns,abnormal heart rhythm,heartbeat estimation,ECG signal,heart conditions,heart failure,heart-monitoring tests,ECG lead configurations,spectro-temporal textures,coronary artery disease,electrocardiogram,machine learning approach,1D local binary patterns,texture-based feature extraction,grayscale cooccurrence matrix,short-time Fourier transform,Morse wavelets,time-frequency SVM model,MIT-BIH database
AI 理解论文
溯源树
样例
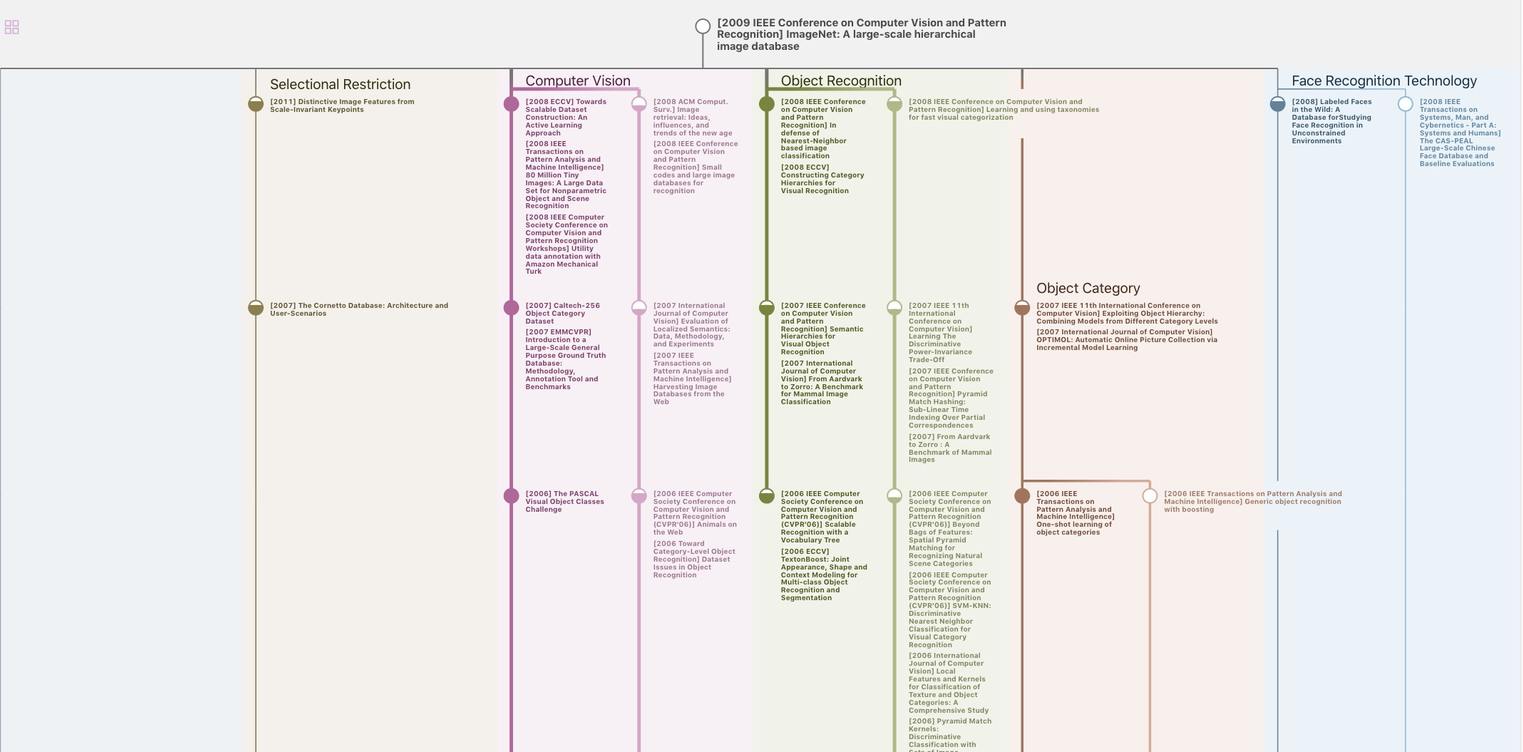
生成溯源树,研究论文发展脉络
Chat Paper
正在生成论文摘要