The Effect on Network Flows-Based Features and Training Set Size on Malware Detection
2018 IEEE 17th International Symposium on Network Computing and Applications (NCA)(2018)
摘要
Although network flows have been used in areas such as network traffic analysis and botnet detection, not many works have used network flows-based features for malware detection. This paper is focused on malware detection based on using features extracted from the network traffic and system logs. We evaluated the performance of four supervised machine learning algorithms (i.e., J48, Random Forest, Naive Bayes, and PART) for malware detection and identified the best learner. Furthermore, we used feature selection based on information gain to identify the smallest number of features needed for classification. In addition, we experimented with training sets of different sizes. The main findings include: (1) Adding network flows-based features improved significantly the performance of malware detection. (2) J48 and PART were the best performing learners, with the highest F-score and G-score values. (3) Using J48, the top five features ranked by information gain attained the same performance as when using all 88 features. In the case of PART, the top fourteen features ranked by information gain led to the same performance as when all 88 features were used. None of the system logs-based features were included in these two models. (4) The classification performance when training on 75% of the data was comparable to training on 90% of the data. As little as 25% of the data can be used for training at an expense of somewhat higher, but not very significant performance degradation (i.e., less than 7% for F-score and 6% for G-score compared to when 90% of the data were used for training).
更多查看译文
关键词
malware detection,network traffic-based features,network flows,system logs-based features,supervised machine learning
AI 理解论文
溯源树
样例
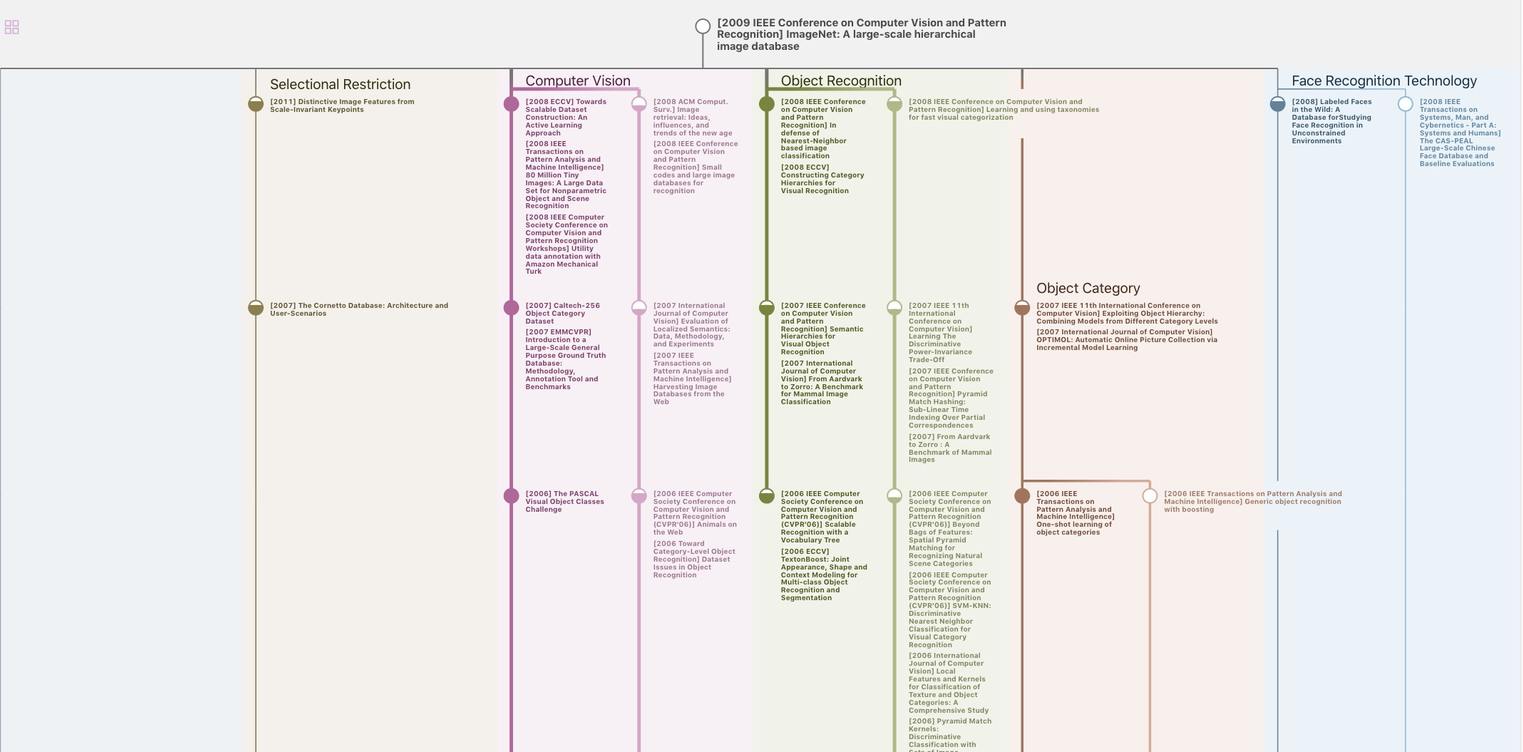
生成溯源树,研究论文发展脉络
Chat Paper
正在生成论文摘要