Hierarchical Population-Based Learning For Optimal Large-Scale Coalition Structure Generation In Smart Grids
AI 2018: ADVANCES IN ARTIFICIAL INTELLIGENCE(2018)
摘要
Large-scale Coalition Structure Generation poses a key challenge in the Cooperative Game Theory and Multi-Agent Systems in regards to its NP-hardness computation complexity. State-of-the-art algorithms, such as Optimal Dynamic Programming, could only solve the problem on a small scale, e.g. 20 agents, with an excessive running time. Our previous study, using population-based learning to deal with the same scale outperforms others and revels an immense potential of efficiency and accuracy. In this study we further advance the problem to large scales, e.g. 80 agents. Firstly, we show that our PBIL-MW algorithm could obtain an approximate optimal solution. Furthermore, we propose an approach of Hierarchical PBIL-MW with a termination scheme that achieves significant efficiency with only small losses in terms of accuracy. It provides an alternative solution, while time restriction is essential in some applications.
更多查看译文
关键词
Coalition Structure Generation, Optimisation Dynamic Programming, Population-Based Incremental Learning, Smart Grids, Hierarchical Structure
AI 理解论文
溯源树
样例
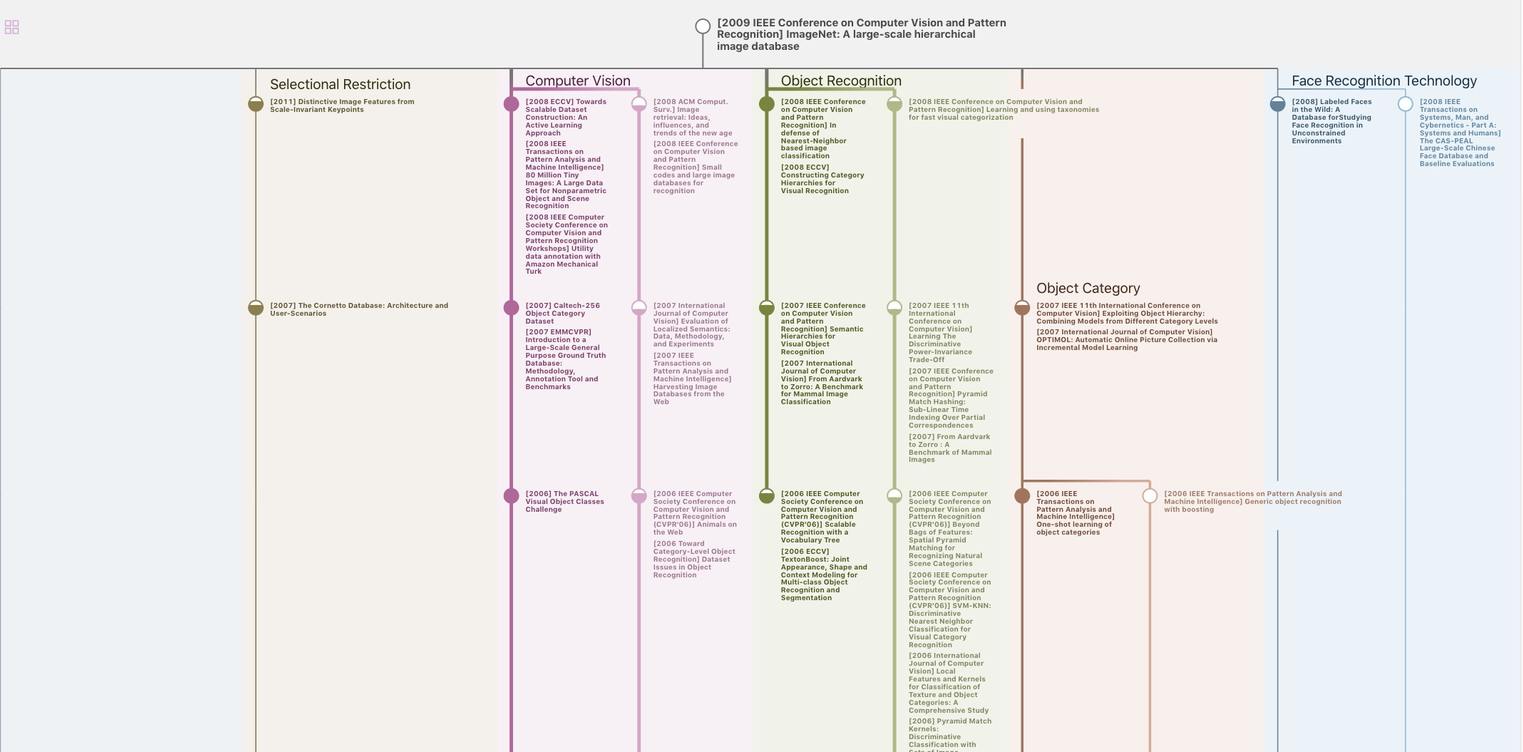
生成溯源树,研究论文发展脉络
Chat Paper
正在生成论文摘要