SAR study on inhibitors of GIIA secreted phospholipase A using machine learning methods.
CHEMICAL BIOLOGY & DRUG DESIGN(2019)
摘要
GIIA secreted phospholipase A(2) (GIIA sPLA(2)) is a potent target for drug discovery. To distinguish the activity level of the inhibitors of GIIA sPLA(2), we built 24 classification models by three machine learning algorithms including support vector machine (SVM), decision tree (DT), and random forest (RF) based on 452 compounds. The molecules were represented by CORINA descriptors, MACCS fingerprints, and ECFP4 fingerprints, respectively. The dataset was split into a training set containing 312 compounds and a test set containing 140 compounds by Kohonen's self-organizing map (SOM) strategy and a random strategy. A recursive feature elimination (RFE) method and an information gain (IG) method were used in the selection of molecular descriptors. Three favorable performing models were obtained. They were built by SVM algorithm with CORINA descriptors (Models 1A and 2A) and ECFP4 fingerprints (Model 10A). In the prediction of test set of Model 10A, the accuracy reached 90.71%, and the Matthews correlation coefficient (MCC) values reached 0.82. In addition, the 452 inhibitors were clustered into eight subsets by K-Means algorithm for analyzing their structural features. It was found that highly active inhibitors mainly contained indole scaffold or indolizine scaffold and four side chains.
更多查看译文
关键词
classification models,decision tree (DT),GIIA sPLA(2) inhibitors,random forest (RF),support vector machine (SVM)
AI 理解论文
溯源树
样例
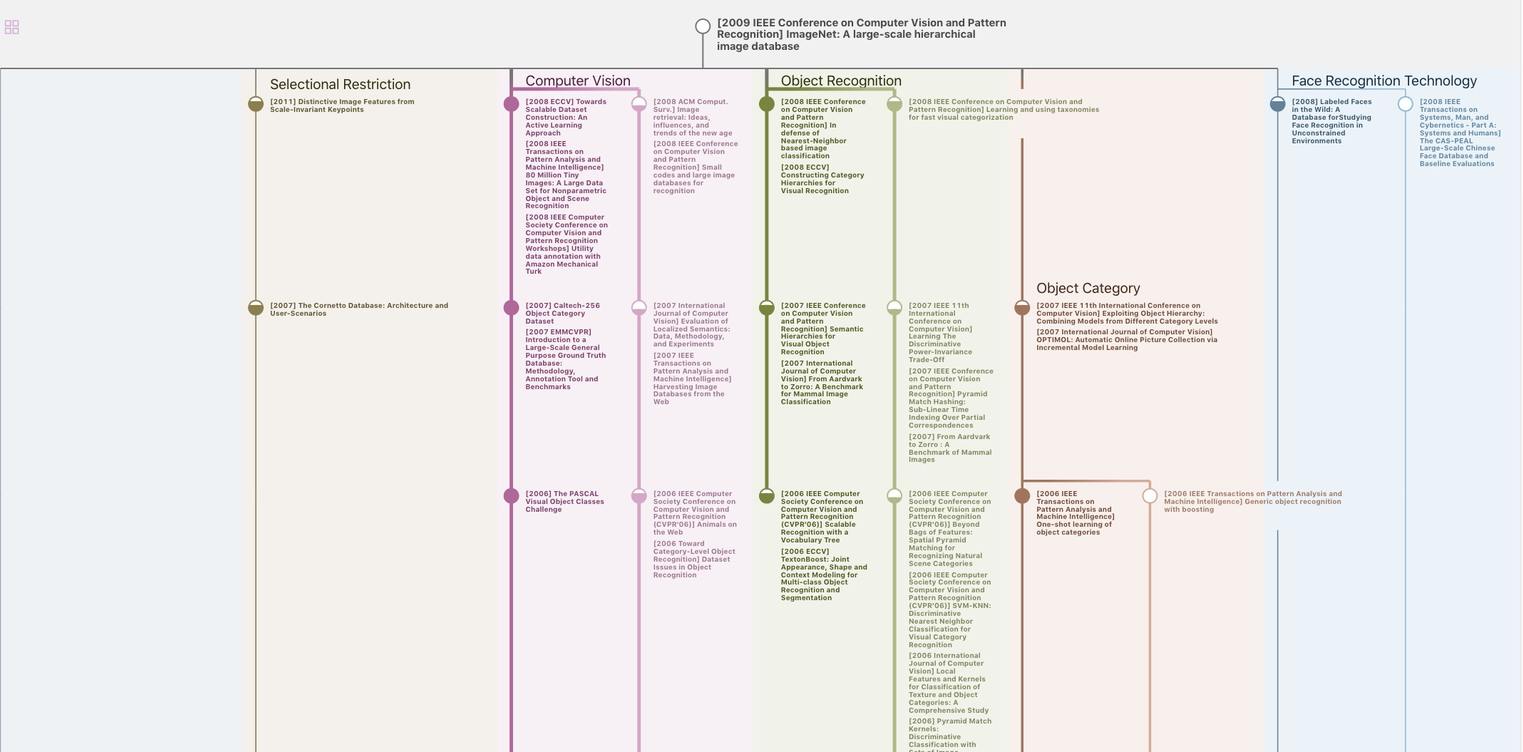
生成溯源树,研究论文发展脉络
Chat Paper
正在生成论文摘要