Integrated accounts of behavioral and neuroimaging data using flexible recurrent neural network models.
ADVANCES IN NEURAL INFORMATION PROCESSING SYSTEMS 31 (NIPS 2018)(2018)
摘要
Neuroscience studies of human decision-making abilities commonly involve subjects completing a decision-making task while BOLD signals are recorded using fMRI. Hypotheses are tested about which brain regions mediate the effect of past experience, such as rewards, on future actions. One standard approach to this is model-based fMRI data analysis, in which a model is fitted to the behavioral data, i.e., a subject's choices, and then the neural data are parsed to find brain regions whose BOLD signals are related to the model's internal signals. However, the internal mechanics of such purely behavioral models are not constrained by the neural data, and therefore might miss or mischaracterize aspects of the brain. To address this limitation, we introduce a new method using recurrent neural network models that are flexible enough to be jointly fitted to the behavioral and neural data. We trained a model so that its internal states were suitably related to neural activity during the task, while at the same time its output predicted the next action a subject would execute. We then used the fitted model to create a novel visualization of the relationship between the activity in brain regions at different times following a reward and the choices the subject subsequently made. Finally, we validated our method using a previously published dataset. We found that the model was able to recover the underlying neural substrates that were discovered by explicit model engineering in the previous work, and also derived new results regarding the temporal pattern of brain activity.
更多查看译文
关键词
the brain,recurrent neural network,neuroscience studies,brain regions,temporal pattern,brain activity,neural substrates,different times
AI 理解论文
溯源树
样例
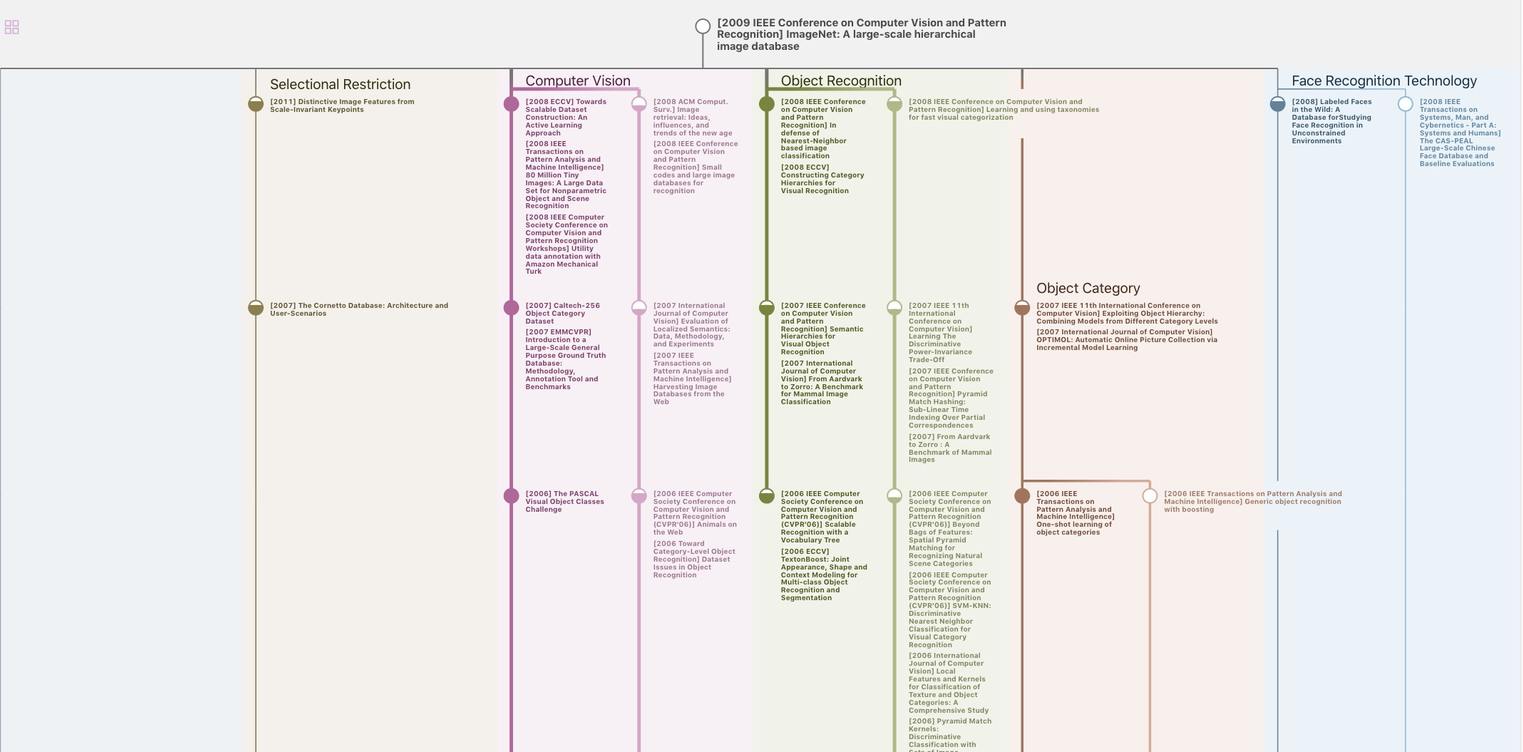
生成溯源树,研究论文发展脉络
Chat Paper
正在生成论文摘要