Synthesize Policies for Transfer and Adaptation across Tasks and Environments.
ADVANCES IN NEURAL INFORMATION PROCESSING SYSTEMS 31 (NIPS 2018)(2019)
摘要
The ability to transfer in reinforcement learning is key towards building an agent of general artificial intelligence. In this paper, we consider the problem of learning to simultaneously transfer across both environments (epsilon) and tasks (tau), probably more importantly, by learning from only sparse (epsilon, tau) pairs out of all the possible combinations. We propose a novel compositional neural network architecture which depicts a meta rule for composing policies from environment and task embeddings. Notably, one of the main challenges is to learn the embeddings jointly with the meta rule. We further propose new training methods to disentangle the embeddings, making them both distinctive signatures of the environments and tasks and effective building blocks for composing the policies. Experiments on GRIDWORLD and THOR, of which the agent takes as input an egocentric view, show that our approach gives rise to high success rates on all the (epsilon, tau) pairs after learning from only 40% of them.
更多查看译文
关键词
the problem,reinforcement learning,the agent,general artificial intelligence,the main
AI 理解论文
溯源树
样例
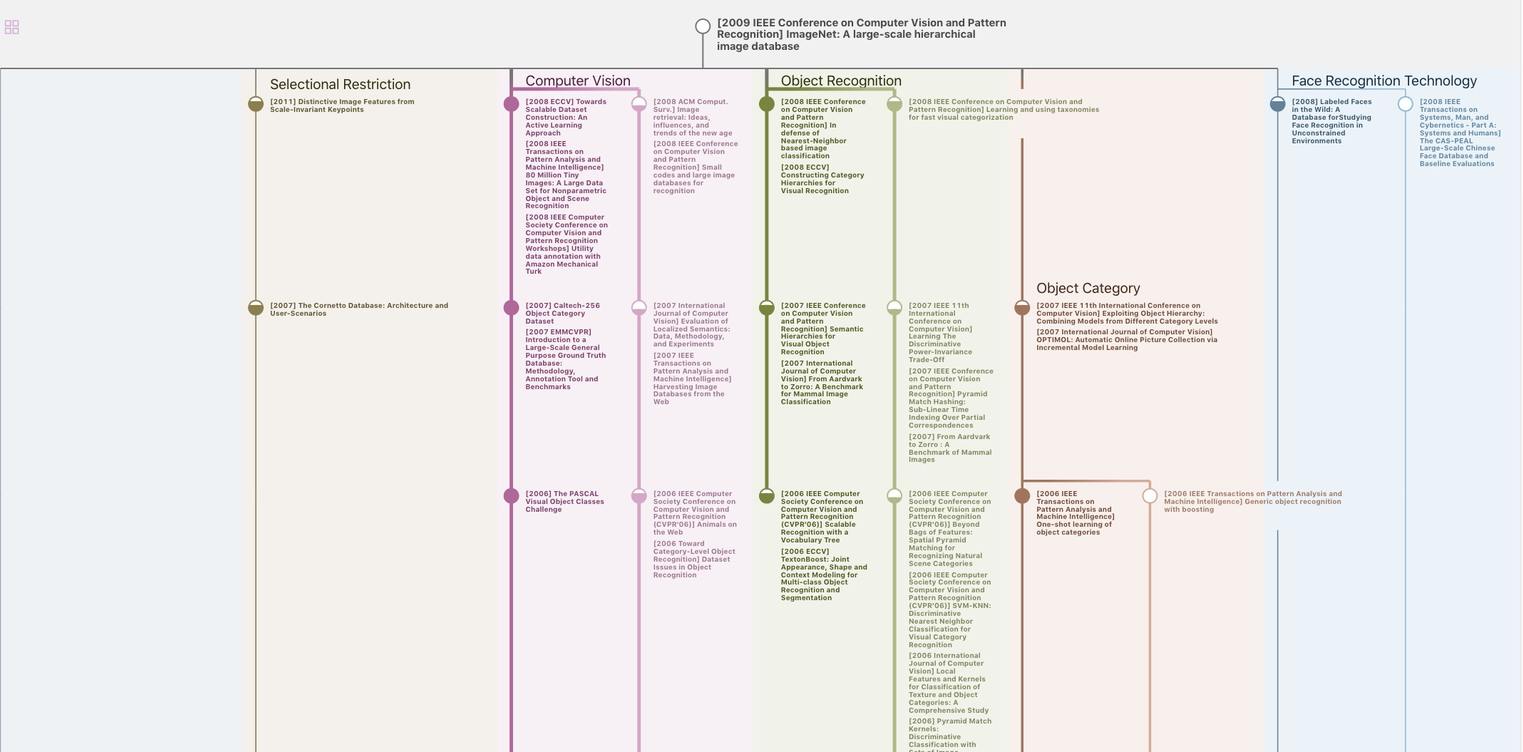
生成溯源树,研究论文发展脉络
Chat Paper
正在生成论文摘要