Completing State Representations using Spectral Learning.
ADVANCES IN NEURAL INFORMATION PROCESSING SYSTEMS 31 (NIPS 2018)(2018)
摘要
A central problem in dynamical system modeling is state discovery-that is, finding a compact summary of the past that captures the information needed to predict the future. Predictive State Representations (PSRs) enable clever spectral methods for state discovery; however, while consistent in the limit of infinite data, these methods often suffer from poor performance in the low data regime. In this paper we develop a novel algorithm for incorporating domain knowledge, in the form of an imperfect state representation, as side information to speed spectral learning for PSRs. We prove theoretical results characterizing the relevance of a user-provided state representation, and design spectral algorithms that can take advantage of a relevant representation. Our algorithm utilizes principal angles to extract the relevant components of the representation, and is robust to mis-specification. Empirical evaluation on synthetic HMMs, an aircraft identification domain, and a gene splice dataset shows that, even with weak domain knowledge, the algorithm can significantly outperform standard PSR learning.
更多查看译文
关键词
constant factors,domain knowledge,principal angles
AI 理解论文
溯源树
样例
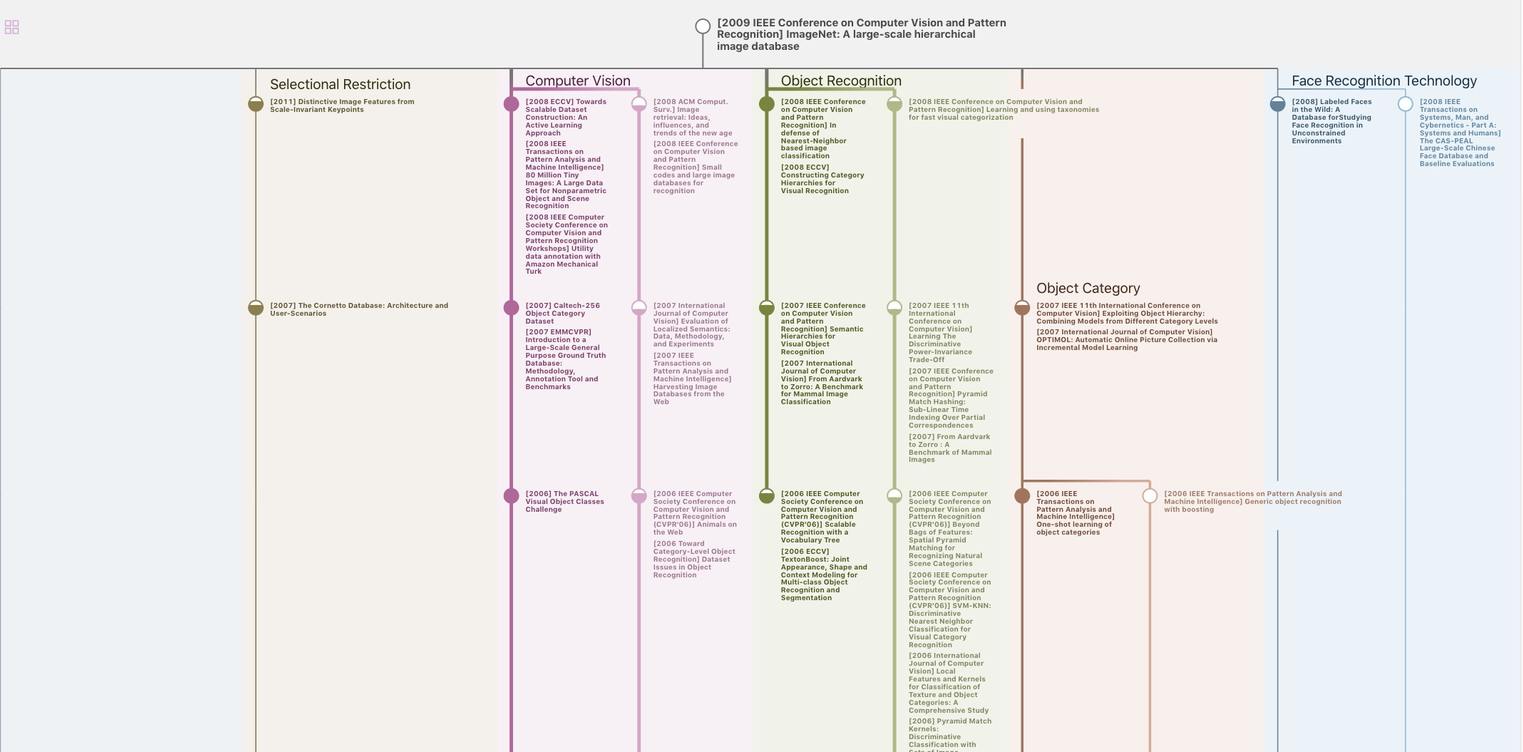
生成溯源树,研究论文发展脉络
Chat Paper
正在生成论文摘要