Contextual Pricing for Lipschitz Buyers.
ADVANCES IN NEURAL INFORMATION PROCESSING SYSTEMS 31 (NIPS 2018)(2018)
摘要
We investigate the problem of learning a Lipschitz function from binary feedback. In this problem, a learner is trying to learn a Lipschitz function f : [0, 1](d) -> [0, 1] over the course of T rounds. On round t, an adversary provides the learner with an input x(t), the learner submits a guess y(t) for f(x(t)), and learns whether y(t) > f(x(t)) or y(t) <= f (x(t)). The learner's goal is to minimize their total loss Sigma(t) l(f(x(t)), y(t)) (for some loss function l). The problem is motivated by contextual dynamic pricing, where a firm must sell a stream of differentiated products to a collection of buyers with non-linear valuations for the items and observes only whether the item was sold or not at the posted price. For the symmetric loss l(f (x(t)), y(t)) = vertical bar f(x(t)) - y(t)vertical bar, we provide an algorithm for this problem achieving total loss O (log T) when d = 1 and O(T-(d 1)/d when d > 1, and show that both bounds are tight (up to a factor of root log T). For the pricing loss function l(f(x(t)), y(t)) = f(x(t)) - y(t)1{y(t) <= f(x(t)) g we show a regret bound of O(Td/(d+1)) and show that this bound is tight. We present improved bounds in the special case of a population of linear buyers.
更多查看译文
关键词
the problem,loss function,full spectrum,lipschitz functions,total loss,lipschitz function,the course,posted price,contextual pricing for lipschitz buyers
AI 理解论文
溯源树
样例
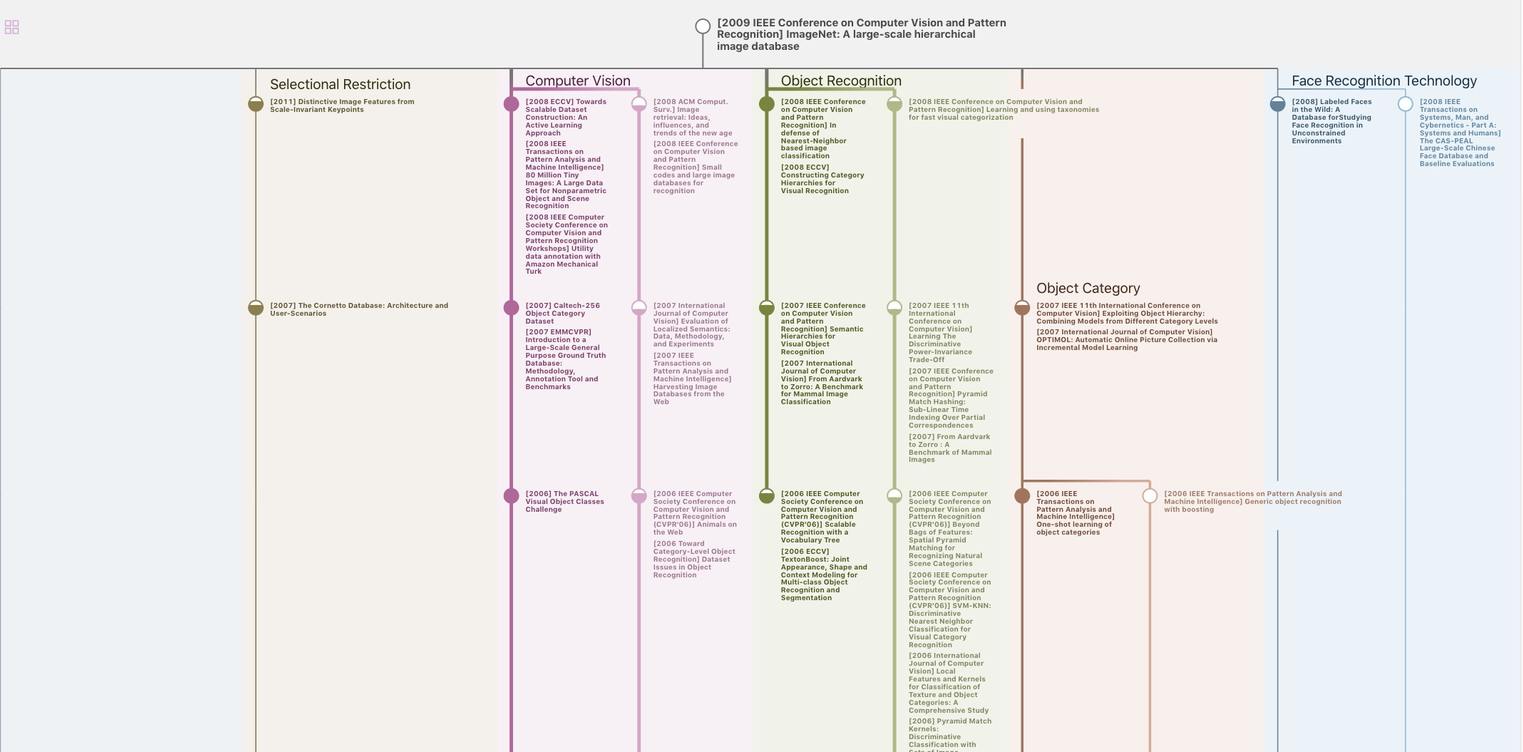
生成溯源树,研究论文发展脉络
Chat Paper
正在生成论文摘要