MacNet: Transferring Knowledge from Machine Comprehension to Sequence-to-Sequence Models
ADVANCES IN NEURAL INFORMATION PROCESSING SYSTEMS 31 (NIPS 2018)(2019)
摘要
Machine Comprehension (MC) is one of the core problems in natural language processing, requiring both understanding of the natural language and knowledge about the world. Rapid progress has been made since the release of several benchmark datasets, and recently the state-of-the-art models even surpass human performance on the well-known SQuAD evaluation. In this paper, we transfer knowledge learned from machine comprehension to the sequence-to-sequence tasks to deepen the understanding of the text. We propose MacNet: a novel encoder-decoder supplementary architecture to the widely used attention-based sequence-to-sequence models. Experiments on neural machine translation (NMT) and abstractive text summarization show that our proposed framework can significantly improve the performance of the baseline models, and our method for the abstractive text summarization achieves the state-of-the-art results on the Gigaword dataset.
更多查看译文
关键词
natural language,natural language processing,neural machine translation,human performance
AI 理解论文
溯源树
样例
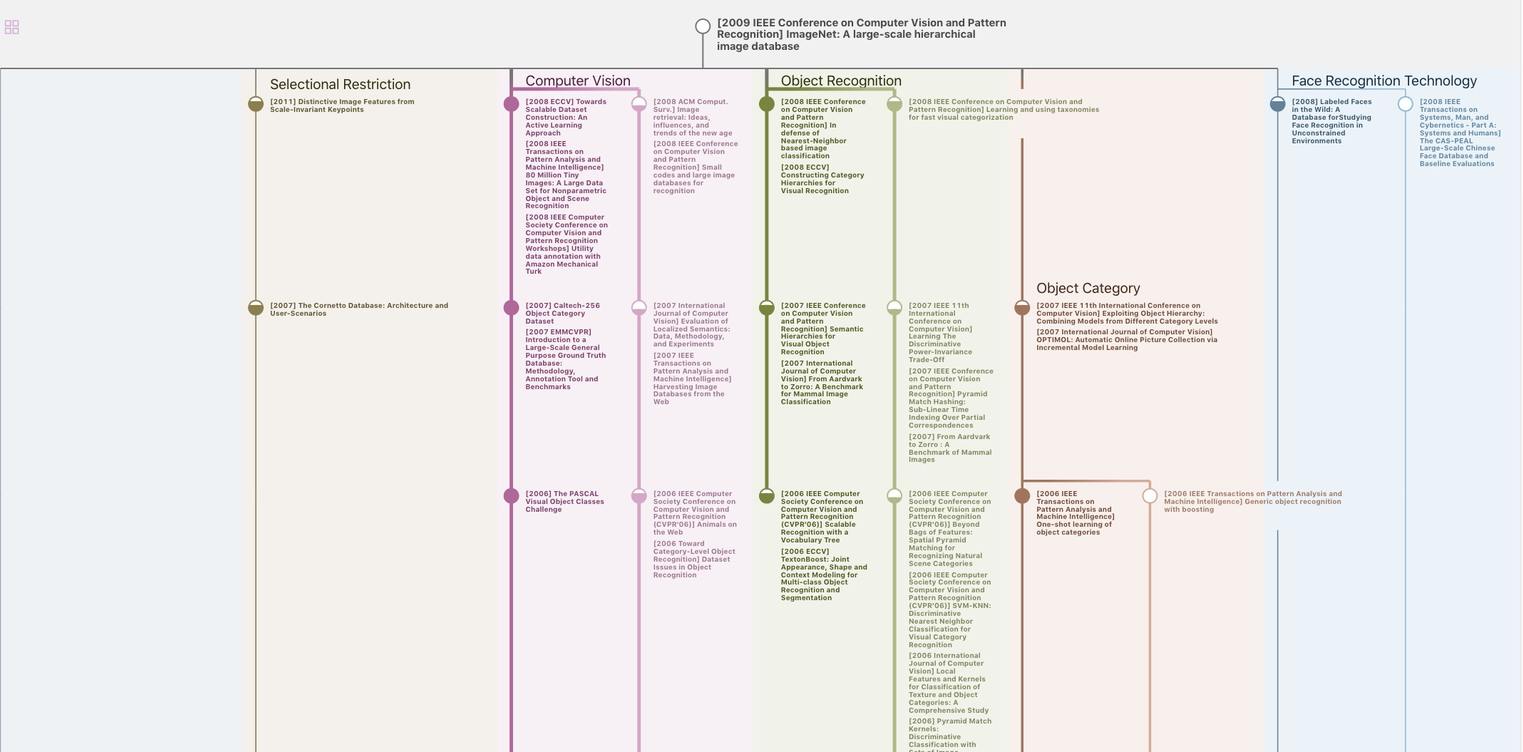
生成溯源树,研究论文发展脉络
Chat Paper
正在生成论文摘要