Learning to Reconstruct Shapes from Unseen Classes
neural information processing systems(2018)
摘要
From a single image, humans are able to perceive the full 3D shape of an object by exploiting learned shape priors from everyday life. Contemporary single-image 3D reconstruction algorithms aim to solve this task in a similar fashion, but often end up with priors that are highly biased by training classes. Here we present an algorithm, Generalizable Reconstruction (GenRe), designed to capture more generic, class-agnostic shape priors. We achieve this with an inference network and training procedure that combine 2.5D representations of visible surfaces (depth and silhouette), spherical shape representations of both visible and non-visible surfaces, and 3D voxel-based representations, in a principled manner that exploits the causal structure of how 3D shapes give rise to 2D images. Experiments demonstrate that GenRe performs well on single-view shape reconstruction, and generalizes to diverse novel objects from categories not seen during training.
更多查看译文
关键词
inference network,causal structure,3d shapes,everyday life
AI 理解论文
溯源树
样例
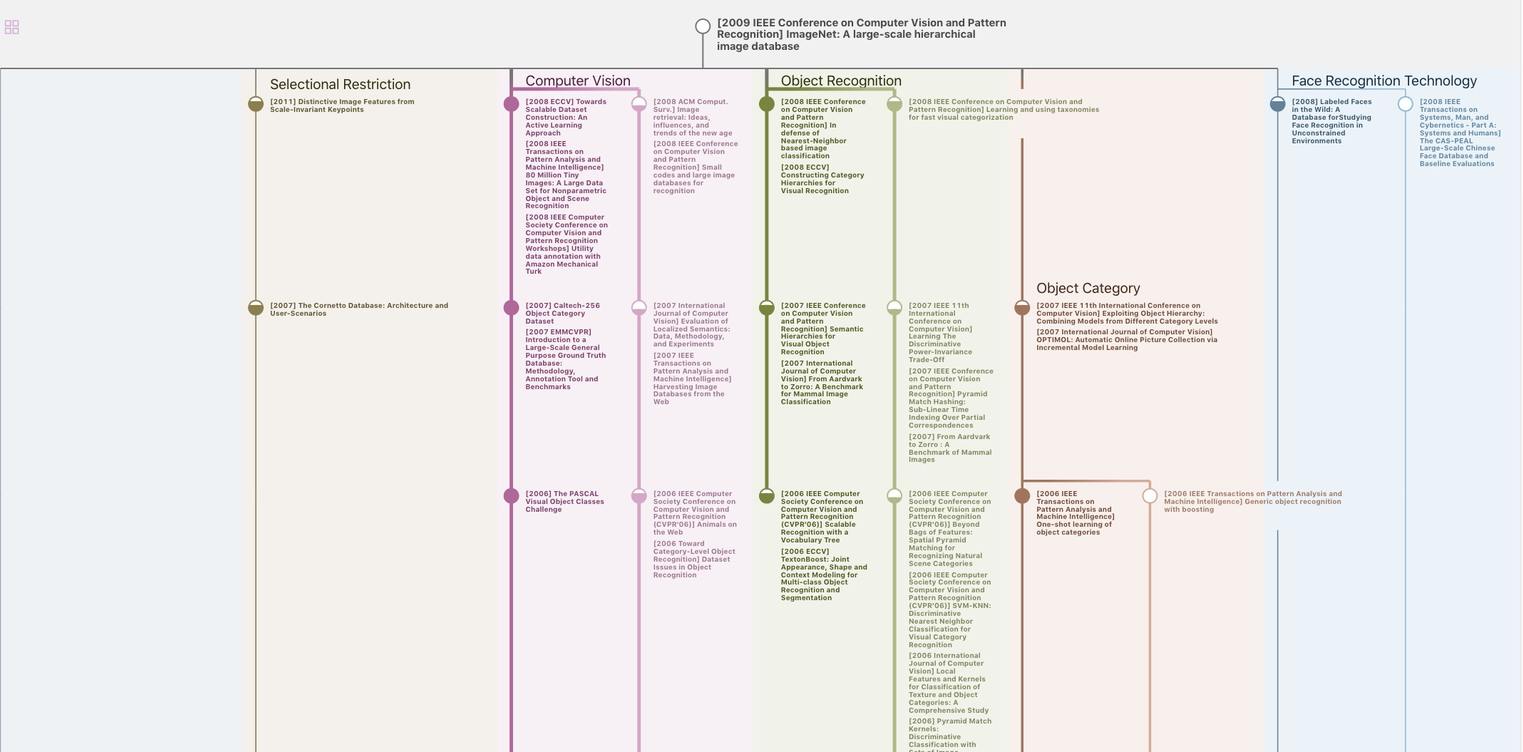
生成溯源树,研究论文发展脉络
Chat Paper
正在生成论文摘要