Progress Maximization Model Predictive Controller
2018 21ST INTERNATIONAL CONFERENCE ON INTELLIGENT TRANSPORTATION SYSTEMS (ITSC)(2018)
摘要
This paper addresses the problem of progress maximization (i.e., traveling time minimization) along a given path for autonomous vehicles. Progress maximization plays an important role not only in racing, but also in efficient and safe autonomous driving applications. The progress maximization problem is formulated as a model predictive controller, where the vehicle model is successively linearized at each time step, yielding a convex optimization problem. To ensure real-time feasibility, a kinematic vehicle model is used together with several linear approximations of the vehicle dynamics constraints. We propose a novel polytopic approximation of the 'g-g' diagram, which models the vehicle handling limits by constraining the lateral and longitudinal acceleration. Moreover, the tire slip angles are restricted to ensure that the tires of the vehicle always operate in their linear force region by limiting the lateral acceleration. We illustrate the effectiveness of the proposed controller in simulation, where a nonlinear dynamic vehicle model is controlled to maximize the progress along a track, taking into consideration possible obstacles.
更多查看译文
关键词
autonomous vehicles,efficient driving applications,safe autonomous driving applications,convex optimization problem,real-time feasibility,kinematic vehicle model,linear approximations,linear force region,nonlinear dynamic vehicle model,progress maximization model predictive controller,vehicle dynamic constraints
AI 理解论文
溯源树
样例
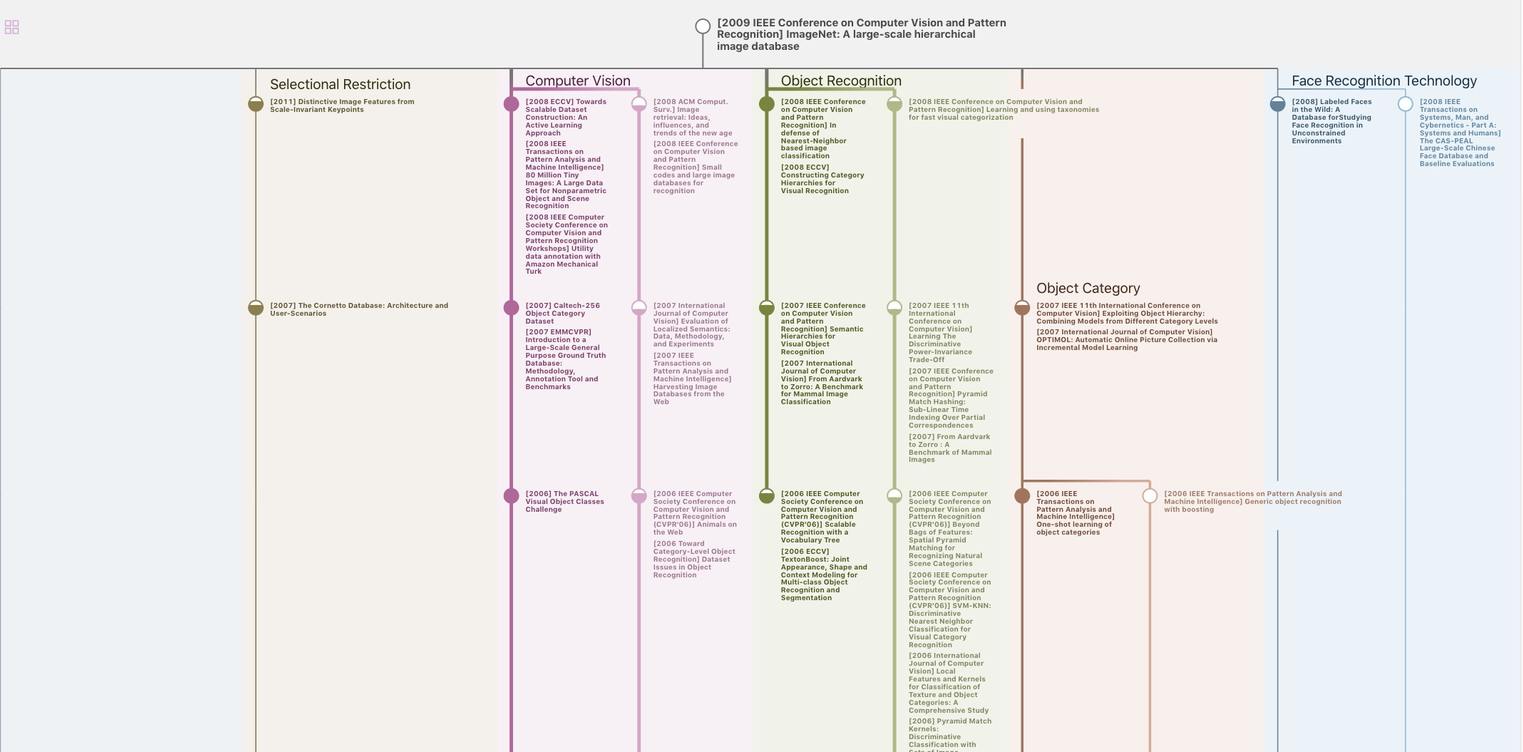
生成溯源树,研究论文发展脉络
Chat Paper
正在生成论文摘要