Traffic Signal Control Based On Reinforcement Learning With Graph Convolutional Neural Nets
2018 21ST INTERNATIONAL CONFERENCE ON INTELLIGENT TRANSPORTATION SYSTEMS (ITSC)(2018)
摘要
Traffic signal control can mitigate traffic congestion and reduce travel time. A model-free reinforcement learning (RL) approach is a powerful framework for learning a responsive traffic control policy for short-term traffic demand changes without prior environmental knowledge. Previous RL approaches could handle high-dimensional feature space using a standard neural network, e.g., a convolutional neural network; however, to control traffic on a road network with multiple intersections, the geometric features between roads had to be created manually. Rather than using manually crafted geometric features, we developed an RL-based traffic signal control method that employs a graph convolutional neural network (GCNN). GCNNs can automatically extract features considering the traffic features between distant roads by stacking multiple neural network layers. We numerically evaluated the proposed method in a six-intersection environment. The results demonstrate that the proposed method can find comparable policies twice as fast as the conventional RL method with a neural network and can adapt to more extensive traffic demand changes.
更多查看译文
关键词
traffic features,multiple neural network layers,extensive traffic demand changes,traffic congestion,model-free reinforcement learning approach,responsive traffic control policy,short-term traffic demand changes,high-dimensional feature space,road network,geometric features,RL-based traffic signal control method,graph convolutional neural network,GCNN
AI 理解论文
溯源树
样例
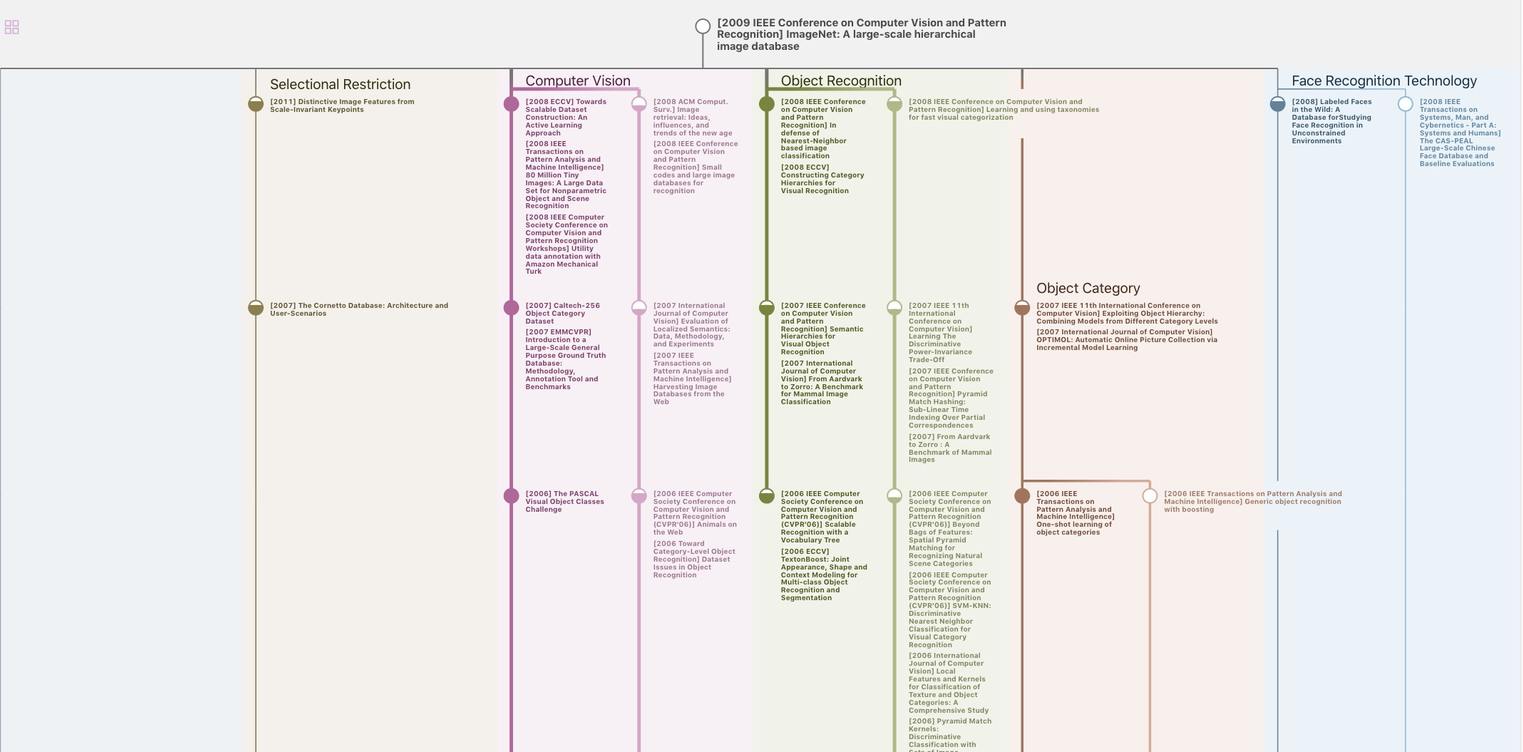
生成溯源树,研究论文发展脉络
Chat Paper
正在生成论文摘要