Efficient Dense-Dilation Network for Pavement Cracks Detection with Large Input Image Size.
International Conference on Intelligent Transportation Systems(2018)
摘要
Window-sliding/region-proposal based methods have been the popular approaches for object detection with deep convolutional neural networks. However, these methods are very inefficient when the input image size is large, such as pavement images (2000×4000-pixel) used for cracking detection. In this paper, we propose a solution to this problem by introducing a fully convolutional dense-dilation network and the corresponding training strategy. The network is trained with small image blocks, then works on full-size images, which only needs to forward once for the process. In the first phase, it trains a classification network which classifies a small image block as crack, sealed crack or background. In the second phase, the fully convolutional layer is employed to convert the classification network into a detection network that is insensitive to the input size. At last, via introducing the equivalent dense-dilation design, it transfers both the low-level and middle-level knowledge from the classification network to facilitate the end-to-end network refining and improve the crack localization accuracy. The proposed approach is validated on 600 pavement images (2000×4000-pixel) obtained by industry equipment and it achieves state-of-the-art performance comparing with that of the recently published works in efficiency and accuracy.
更多查看译文
关键词
classification network,end-to-end network refining,object detection,deep convolutional neural networks,crack localization,pavement images,pavement crack detection,window-sliding based methods,region-proposal based methods,fully convolutional dense-dilation network,deep convolutional neural network
AI 理解论文
溯源树
样例
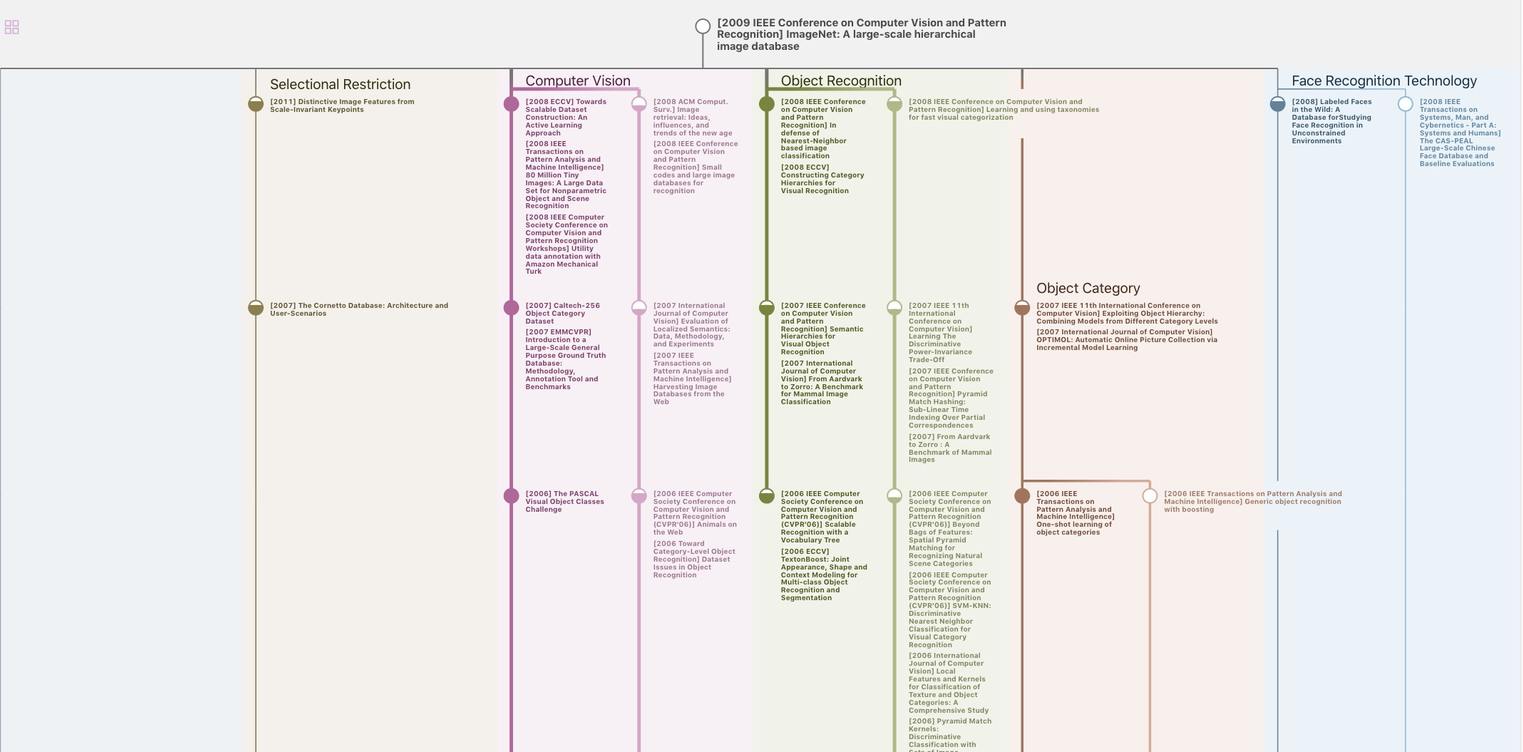
生成溯源树,研究论文发展脉络
Chat Paper
正在生成论文摘要