Crowdsourcing based large-scale network anomaly detection
2018 10th International Conference on Wireless Communications and Signal Processing (WCSP)(2018)
摘要
In recent years, emerging smartphones have proliferated fast growth of Over The Top (OTT) services and rapid expansion of mobile networks. However, the underlying network structure is trouble-prone due to massive integrated Internet Service Providers (ISP). Traditional approaches to maintain the network performance cannot satisfy requirements under such a large-scale network. As a result, a low cost and highly efficient anomaly detection mechanism is still unavailable. In this paper we propose a data mining framework to extract network anomalies from crowdsourced network measuring dataset. We first explore the crowdsourced dataset by feature engineering and instance clustering. On the basis of the preprocessed dataset, we propose weighted-apriori rule mining to find out the association between high RTTs and other features thus localizing the network anomaly. We conduct extensive experiments based on a large-scale crowdsourced network dataset with five million samples. The dataset involves round trip time (RTT) information from 6226 kinds of applications and more than 5000 users. Experiments show that our approaches can effectively detect anomalous network conditions.
更多查看译文
关键词
Network Anomaly Detection,Crowdsourcing,Apriori rule mining
AI 理解论文
溯源树
样例
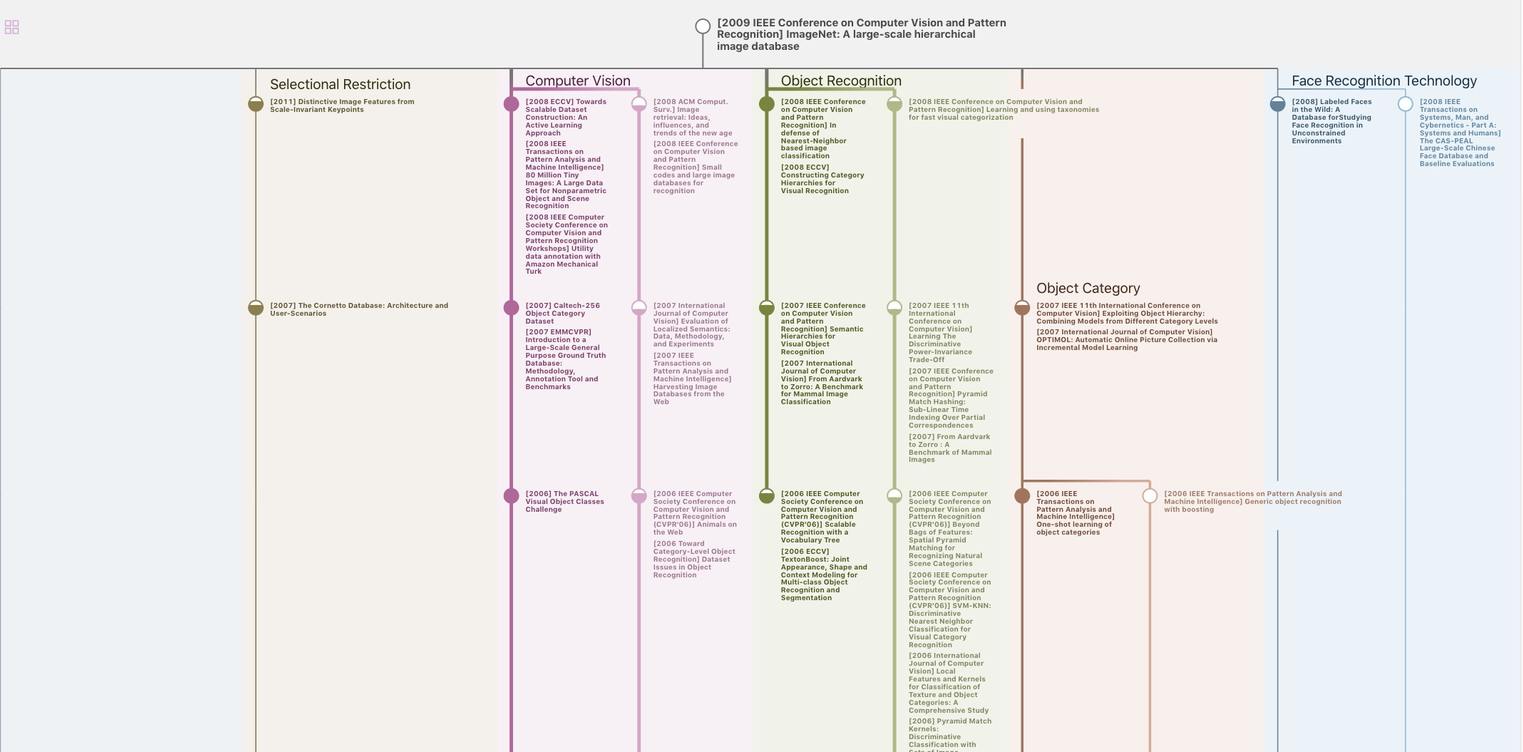
生成溯源树,研究论文发展脉络
Chat Paper
正在生成论文摘要