Hyperspectral Target Detection Based On A Spatially Regularized Sparse Representation
2018 10TH INTERNATIONAL CONFERENCE ON WIRELESS COMMUNICATIONS AND SIGNAL PROCESSING (WCSP)(2018)
摘要
Sparse representation (SR) is an effective method for target detection in hyperspectral imagery (HSI). The structured dictionary is arranged according to the target class and the background class, the sparse coefficients associated with each dictionary element of a given test sample can be recovered by solving an l(1)-norm minimization problem. It is possible to introduce further regularization to improve the detection performance. The classical SR detection algorithms does not consider the spatial information of the detected pixels. It can be expected that sparse coefficients of adjacent pixels are similar due to the spatial correlation. This paper proposes a novel SR model w'hich takes into account a spatial regularization term to promote the piecewise continuity of the sparse vectors. The formulated problem is solved via alternating direction method of multipliers (ADMM). We illustrate the enhanced performance of the proposed algorithm via both synthetic and real hyperspectral data.
更多查看译文
关键词
Hyperspectral imagery, target detection, sparse representation, spatial correlation constraint, ADMM
AI 理解论文
溯源树
样例
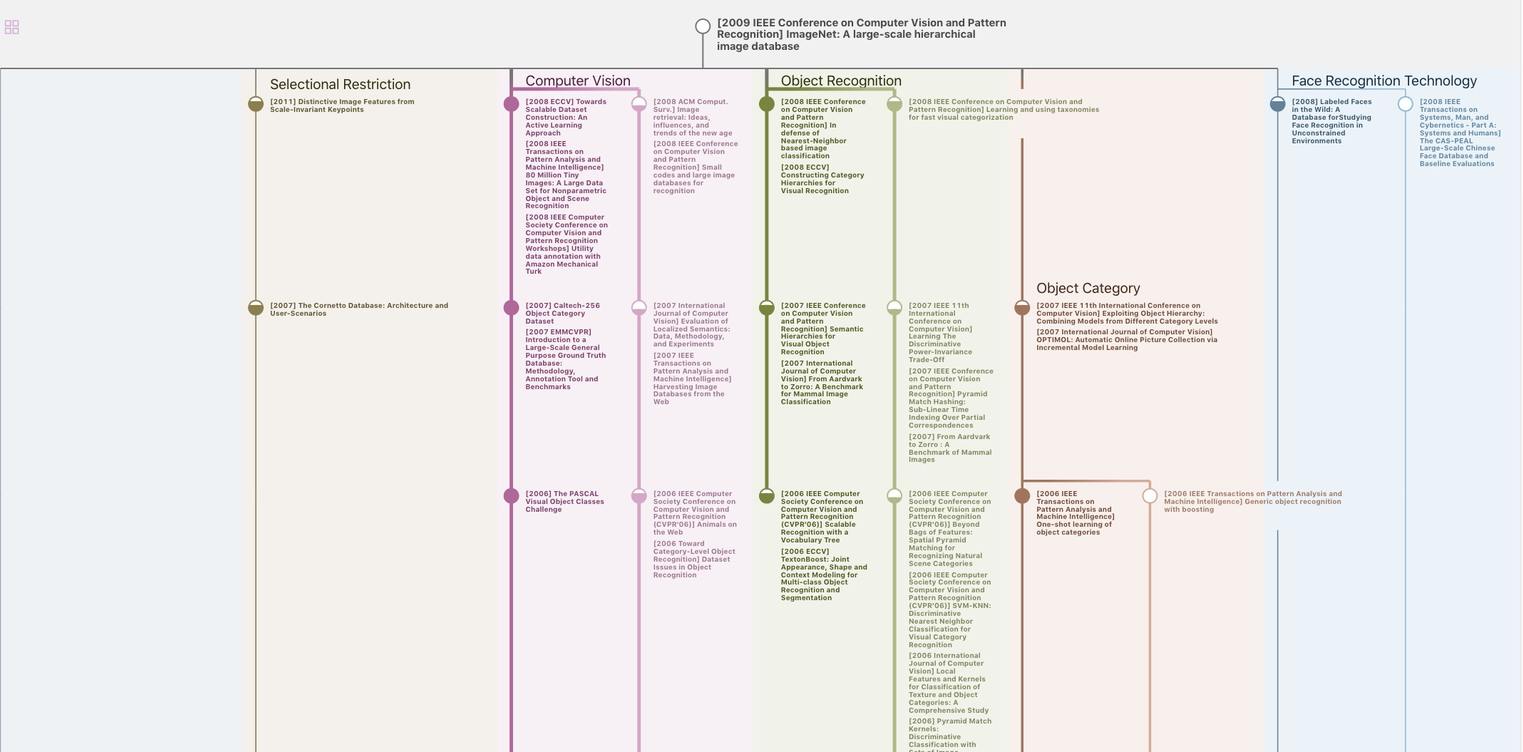
生成溯源树,研究论文发展脉络
Chat Paper
正在生成论文摘要