Computational Diagnosis of Parkinson's Disease from Speech Based on Regularization Methods.
European Signal Processing Conference(2018)
摘要
A computational tool to discriminate healthy people from people with Parkinson's Disease (PD) is proposed based on acoustic features extracted from sustained vowel recordings. Several approaches based on different feature sets and regularization methods (LASSO, Ridge, and Elastic net) are experimentally compared. The effectiveness of these methods has been evaluated on a dataset containing acoustic features of 40 healthy people and 40 patients with PD, who have been recruited at the Regional Association for Parkinson's Disease in Extremadura (Spain). The results show relevant differences when varying the initial feature set but high stability when changing the regularization approach. The three considered methods have achieved very promising classification accuracy rates via 10-fold cross-validation analysis, reaching 88.5%.
更多查看译文
关键词
Acoustic features,Elastic net,Least absolute shrinkage and selection operator,Nonlinear speech signal processing,Parkinson's disease,Regularized regression,Ridge
AI 理解论文
溯源树
样例
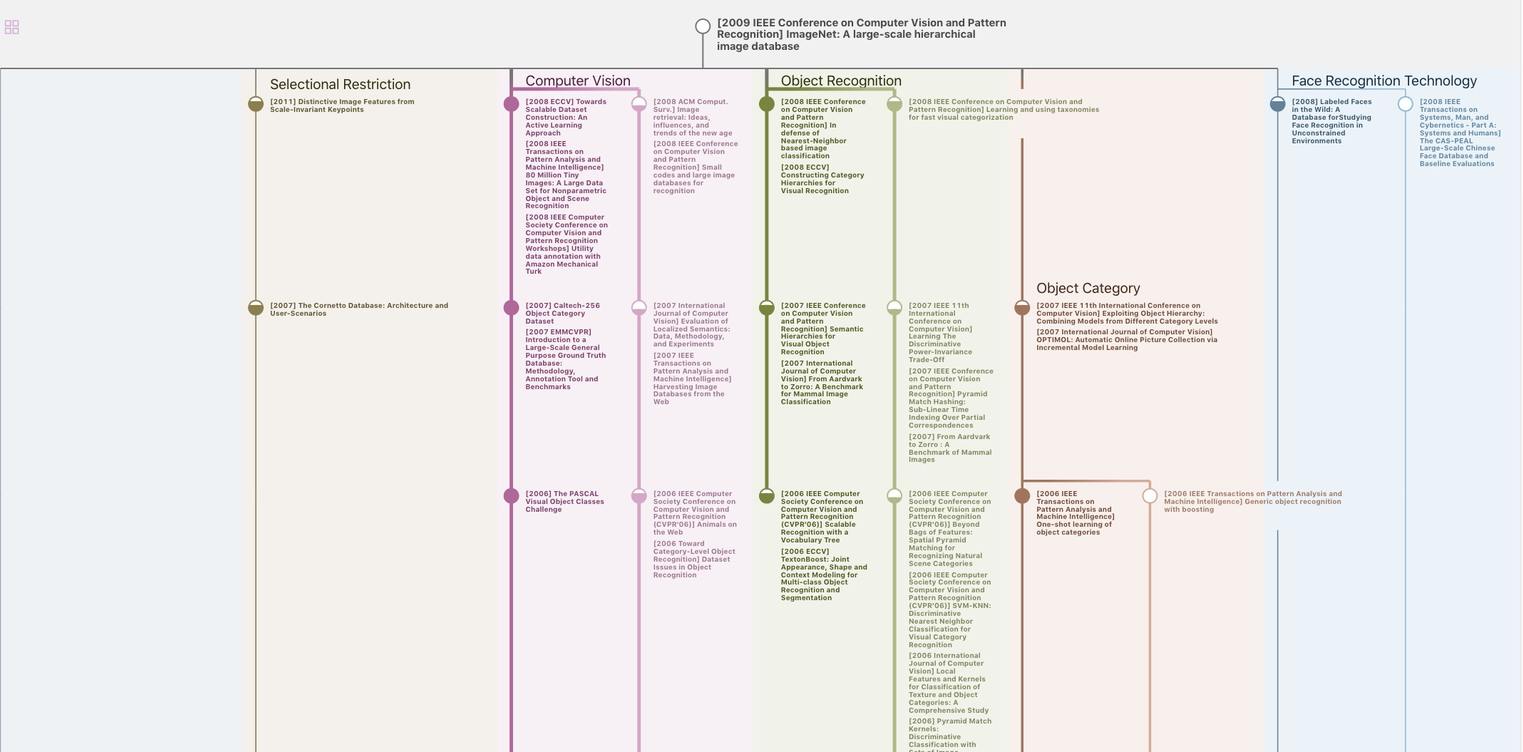
生成溯源树,研究论文发展脉络
Chat Paper
正在生成论文摘要